INTRODUCTION
In response to the growing global demand for animal protein, modern livestock agriculture has shifted towards intensive production systems. However, this approach often leads to larger herd sizes, making it challenging to monitor the health and welfare of farm animals effectively [1]. Pigs play a major role in food productivity for human consumption [2]. Globally, pig meat is the second most preferred meat for consumption after poultry because of its relatively low cost.
Pig farms commonly face challenges, including disease outbreaks and events, which can have severe consequences. Diseases such as African swine fever (ASF), porcine epidemic diarrhea, and swine influenza can cause substantial economic losses for farmers and pose risks to food security [3]. Furthermore, events such as crush incidents can result in significant injuries to pigs, leading to decreased productivity and heightened expenses for farmers [4]. The early detection of diseases and accidents is crucial for preventing disease spread and ensuring prompt treatment for injured pigs. Traditional monitoring methods, such as manual observation and physical examination, are time-consuming, labor intensive, and often inaccurate. Advanced sensors and computer vision-based techniques offer a promising solution to these problems by providing real-time, accurate monitoring of pig health and behavior. In order to monitor animals effectively, it is important to embrace modern technologies such as infrared thermography (IRT) for detection, surveillance, and remote monitoring. The utilization of this technology generates a significant amount of data. Therefore, there is a need for automated analysis of extensive thermographic datasets to furnish essential insights for informed decision-making and dynamic interventions. By implementing automated livestock farming systems, many aspects of animal husbandry can be simplified, including early warnings and proactive actions, as mentioned by Lowe et al. [5], where this approach resulted in reduced unnecessary medical treatments, labor, and treatment costs, ultimately leading to higher profit margins.
In the 1960s, IRT was initially developed in the United States, mainly for military purposes such as inspection, security, and thermal signature detection, as mentioned by Rogalski [6]. However, recently, IRT has found extensive applications in various non-military fields. It has been widely utilized in medical imaging and diagnosis [7], ecological research [8,9], and even in engineering for building structure analyses [10]. The versatility of IRT application extends to diverse domains, including human medicine, veterinary medicine, ecological applications, and industrial applications. It is particularly useful for diagnostic purposes in both human and veterinary medicine, as demonstrated in studies by Schaefer et al. [11] and Martins et al. [12]. One of the key advantages of this technique is that it eliminates the need for physical contact with the surface being monitored, enabling remote temperature distribution measurement, as pointed out by Speakmen and Ward [13]. This highlights the diverse range of research and applications where IRT has been successfully used in animal production systems. In the field of veterinary medicine, IRT has gained popularity because of its ability to measure variations in animal surface temperatures. These temperature changes can provide valuable insights into different physiological functionalities [14], behavioral patterns [15], and digestive functions [16]. By utilizing IRT, veterinarians can effectively detect alterations in surface temperature changes in animals, which can aid in early fever, posture, and disease detection.
IRT has proven an effective tool in identifying various diseases in animals. It has been successfully used to identify sick or stressed pigs [17], bovine viral diarrhea [18], cattle lameness [19], foot and mouth disease in cattle [20] and sheep [21], mastitis in cattle [12], and heat stress in poultry [22]. Moreover, IRT is also used to identify non-disease symptoms, including breeding, birth signs, etc. [23], as well as to assess animal well-being and productivity [24]. It provides valuable information for monitoring and evaluating the overall health, well-being, and productivity of animals.
Intelligent computer systems are necessary to enable the implementation of automated systems in agriculture. These systems utilize machine learning algorithms to analyze vast datasets, providing valuable insights to farmers and enhancing productivity. In the context of thermographic data, machine learning algorithms can be employed to identify specific regions of interest (ROIs) and process temperature data within these selected ROIs. This processing enables the prediction of outcomes related to agricultural practices [25]. By harnessing the power of machine learning, farmers can make informed decisions based on thermal data analysis, leading to improved efficiency and productivity in their agricultural operations. Thermal sensing, alongside computer vision techniques such as object detection, image segmentation, and deep learning algorithms, can effectively monitor pig behavior and identify disease symptoms. Thermal sensors can track individual pigs and detect temperature variations on their body surface, allowing for the early detection of health issues such as infections or inflammation. Non-intrusive and cost-effective, thermal sensing offers a viable solution for large-scale pig farming, avoiding stress and discomfort caused by invasive methods such as blood tests. The early detection of diseases and crush symptoms can prevent their spread, improve pig welfare, and enhance farm productivity. However, factors such as lighting conditions and technical expertise may influence the accuracy and implementation of thermal sensing techniques.
Recent advancements in sensors and computer vision-based thermal imaging have demonstrated significant potential for monitoring pig diseases and crush symptoms. This article explores their practical applications within pig farming, highlighting the benefits of non-intrusive monitoring and the ability to detect disease indications through thermal patterns. The implications of this technology for enhancing pig welfare, farm efficiency, and environmental sustainability are also examined. Addressing challenges such as accuracy, lighting, and technical know-how, this research underscores the transformative role of thermal imaging in revolutionizing pig health monitoring and its impact on the future of the industry. First, the principles and data processing of IRT are outlined. Next, its applications in detecting pig diseases and crush monitoring using thermal data processing are highlighted. Then, the integration of the machine learning algorithm with thermography data is explored. Additionally, the commercial aspects of IRT sensors are examined. Finally, the review concludes by addressing future trends in IRT and challenges in the context of pig health monitoring.
INFRARED THERMOGRAPHY IMAGING TECHNOLOGY
IRT is a contactless and non-invasive method that relies on the physical principles explained by the Planck, Wien, and Stefan–Boltzmann laws [26]. In accordance with these principles, any object with a temperature above absolute zero diffuses electromagnetic radiation within the infrared (IR) spectrum. The mathematical relationship between the energy emitted from an object’s surface, the wavelength of this radiation, and the object’s temperature is well described by the Stefan–Boltzmann Law [27], specifically:
where σ = 5.669 × 10−8 W/m2K4, the Stefan–Boltzmann constant, As is the surface area of the blackbody, Ts is the surface temperature in Kelvin (K), and Eb is the total emissivity for the blackbody.
By utilizing a sensor array, this emitted radiation can be detected and generate a thermographic image. In such an image, the color intensity in each pixel corresponds proportionally to the observed body temperature [28]. Fig. 1 shows the measurement principle of IRT sensors.
IRT has emerged as a non-invasive diagnostic tool [29] for measuring radiation. Its effectiveness is influenced not only by the temperature of the target object but also by factors such as emissivity and conductivity [30]. However, it is important to acknowledge certain limitations associated with IRT. Factors such as sunlight, high humidity, convective airflow, and dirt on the livestock’s coat should be carefully considered, as they can impact IRT imaging accuracy [31]. The application of IRT in livestock production offers several advantages, including ease of use, cost-effectiveness, speed, efficiency, and the ability to gather crucial information without the need for physical contact with animals [32]. Consequently, IRT represents an innovative strategy with broad potential for enhancing animal welfare and promoting welfare-related practices.
A thermographic camera, also known as an IR camera or thermal imaging camera, is a device that captures images using IR radiation, similar to how a regular camera captures images using visible light. These cameras are specifically designed to detect the radiation emitted from an object within a specific range of IR wavelengths and convert it into an electrical signal, which is processed to create a visual image. IR radiation falls beyond the range of human vision; however, camera sensors can be engineered to detect and utilize this type of radiation. During the daytime, some cameras employ an IR-cut filter to block out IR light and prevent color distortion in images as perceived by the human eye. When the camera switches to night mode, the IR-cut filter is removed, allowing the camera to capture IR light. Since the human eye cannot see IR light, the resulting images are displayed in black and white.
A thermogram is a representation of the apparent temperature distribution on an object’s surface, consisting of pixels [33]. IR cameras measure the intensity of incident radiation, not the temperature directly. Pseudo colors are used to display this data, making invisible electromagnetic spectrum areas visible or highlighting values. The resulting thermogram is monochromatic, but pseudo colors can be applied based on temperature using color palettes such as iron, grayscale, or rainbow [34]. The temperature scale can be fixed or variable, and it can be set in the camera or analysis software [35]. Different manufacturers might have unique color palette names or additional options. To facilitate the detection of near-IR light, a light source is required, either natural, such as moonlight, or artificial, such as streetlights, or a dedicated IR lamp. With advancements in electronics and instrumentation technology, there is a wide range of thermal camera models available on the market, catering to various price points and requirements. Table 1 shows the details of various thermal camera models used in different livestock production applications.
IRT imaging is widely used in animal production to measure object temperature using an IRT detector. However, accurate temperature measurement requires consideration of important thermal imaging parameters, such as emissivity, reflected temperature, distance to the target, ambient temperature, and humidity [36,37]. These parameters must be taken into account in calculations to ensure reliable temperature determination. The proper use of a professional-grade IRT imager, selecting the right model, and considering the imaging angle and thermal imager resolution are also crucial for accurate temperature acquisition. Fernández-Cuevas et al. [38] proposed a classification of factors that influence thermal evaluations as categorized:
-
Environmental factors: These factors pertain to the surrounding conditions in which the thermal evaluation is conducted. They include the ambient temperature, humidity levels, and the presence of any radiation sources.
-
Individual factors: This category can be further divided into intrinsic and extrinsic factors. Intrinsic factors are inherent to the individual being evaluated and may include age, gender, and medical history. Extrinsic factors are related to the activities carried out prior to the evaluation, such as exercise, medical treatments, or food intake.
-
Technical factors: These factors encompass various technical aspects of the thermal evaluation process. They involve considerations such as the resolution and thermal sensitivity of the camera used, the specific protocol followed during the evaluation, and the statistical methods employed for data analysis.
The application of IR in animals might seem unapproachable after this classification, but the good news is that almost all factors affect bodies symmetrically. Therefore, the use of the methodology of thermal asymmetries and a proper protocol to know factors that might create nonsignificant alerts is a strong and reliable way to reduce their impact and optimize the use and benefits of thermography.
IRT is a non-destructive method that uses IR radiation to detect and visualize temperature variations swiftly. This versatile technology serves various purposes, including quality control and the inspection of different electrical and mechanical components and manufacturing parts, defect detection within materials, and continuous monitoring of environmental conditions [28]. The non-invasive nature and ability to swiftly identify temperature anomalies make IRT an invaluable tool in diverse industries and applications. IRT involves capturing temperature variations between different parts of an animal body and its surroundings through thermal radiation [39]. However, the specific ROIs need to be determined based on study objectives before acquiring data. There are two primary approaches to obtaining IR images: capturing a static thermal image for a specific ROI and continuous video recording for continuous image frames [40]. Achieving accurate body temperature measurements with these methods necessitates maintaining the correct distance and angle between the camera and animals [40].
A schematic diagram of the data acquisition and processing procedure for IRT imaging is shown in Fig. 2. Data acquired from IRT devices typically require preprocessing due to various influencing factors, including environmental interference, image quality, data extraction methods, etc. Environmental factors such as visible light and illumination levels can impact IR image quality, while temperature and humidity can affect temperature measurements [36]. Correcting for these influences through preprocessing is essential. Factors such as distance, angle, and obstructions (e.g., mud or dirt) on the animal body can impact image quality and temperature accuracy, requiring the exclusion of low-quality images [20]. A large number of high-quality thermal images are typically collected in experiments, emphasizing the need for thorough image data preprocessing prior to precise temperature analysis [40]. IRT data processing can be categorized into two aspects, spatial and temporal processing. Spatial processing enhances spatial resolution through noise reduction and edge detection, feature extraction, aiding defect detection, and understating thermal distribution [41]. Temporal processing improves temporal resolution by averaging images over time and using statistical methods to identify significant temperature changes, particularly valuable for time-dependent defect identification [42].
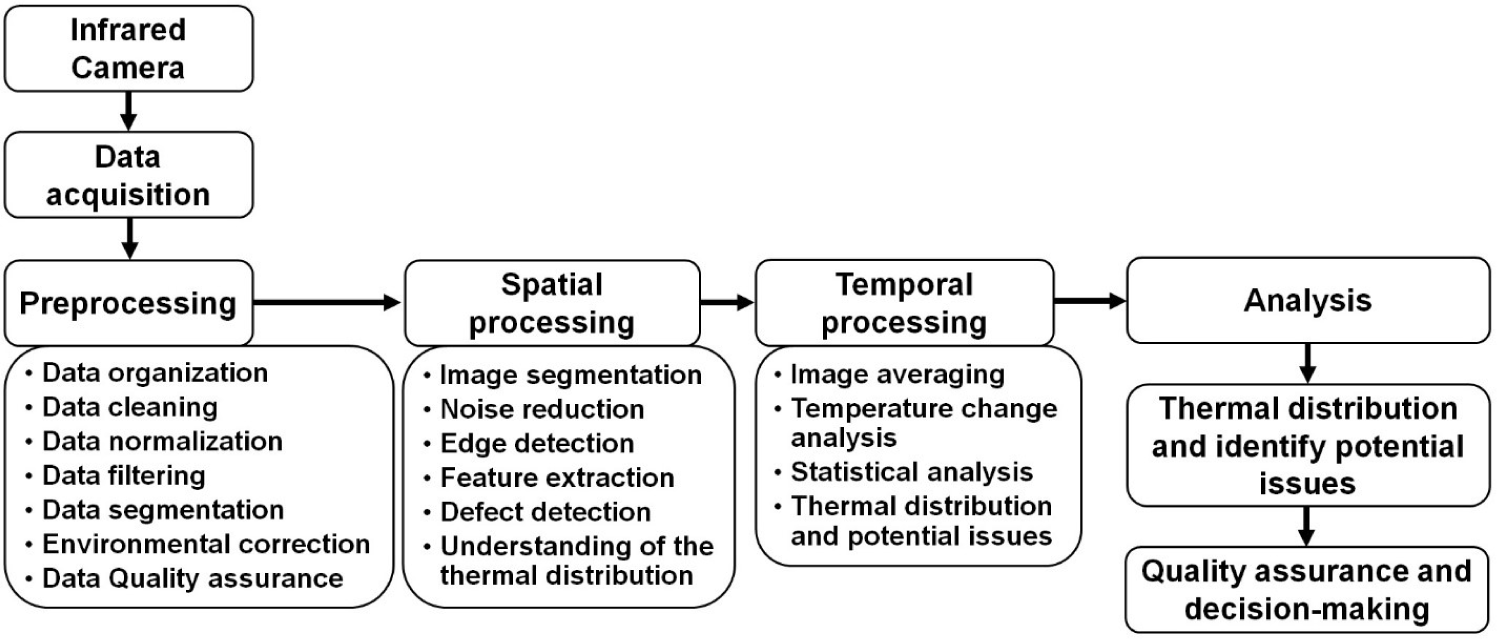
APPLICATION OF INFRARED THERMOGRAPHY FOR PIG DISEASES AND CRUSH SYMPTOM MONITORING
IR imaging technology is valuable in rapidly detecting temperature variations between the surface and core areas of animal bodies, enabling early diagnosis based on differences between natural and pathological conditions [28,43]. IRT has found valuable applications in the monitoring and detection of pig diseases and the assessment of crush symptoms in pig farming. Its application in animal production has grown, with recent research emphasizing parameters such as emissivity, distance, temperature, and humidity [44]. IRT is a valuable tool for detecting and monitoring pig diseases and crush symptoms. It is a non-invasive technique that is safe for pigs, and it can be used to collect data quickly and easily. IRT is a promising technology that has the potential to improve pig welfare and productivity.
Body temperature, a crucial health indicator, is categorized into three classes based on the measurement zone: core temperature (measured close to the main organs via various sensors), mid-peripheral temperature (measured between the core and skin using implanted microchips), and peripheral or surface temperature (measured from the animals skin, eye, ear, udder, or leg) [45]. External measurements are less reliable because of factors such as vasodilatation, vasoconstriction, and external heat sources. However, they offer less invasive and less painful methods for animals, making them reasonable monitoring alternatives. Stukelj et al. [46] conducted a study to determine the most appropriate anatomical site for temperature measurement in healthy pigs using thermal imaging. Correlations between skin temperature and rectal temperature assessed the feasibility of predicting pig health through thermal imaging and compared low and high-spatial-resolution cameras. Two calibrated thermal imaging cameras were used to measure skin temperature at four different anatomical sites in sows, the ear canal, eye canthus, outer ear, and perianal area. The findings indicated that using a high noise equivalent temperature difference (NETD), low accuracy, and low spatial resolution thermal imaging camera did not permit accurate temperature measurement or prediction of pig body temperature. Fig. 3 shows different pig body temperature measurement and monitoring using IRT imageing.
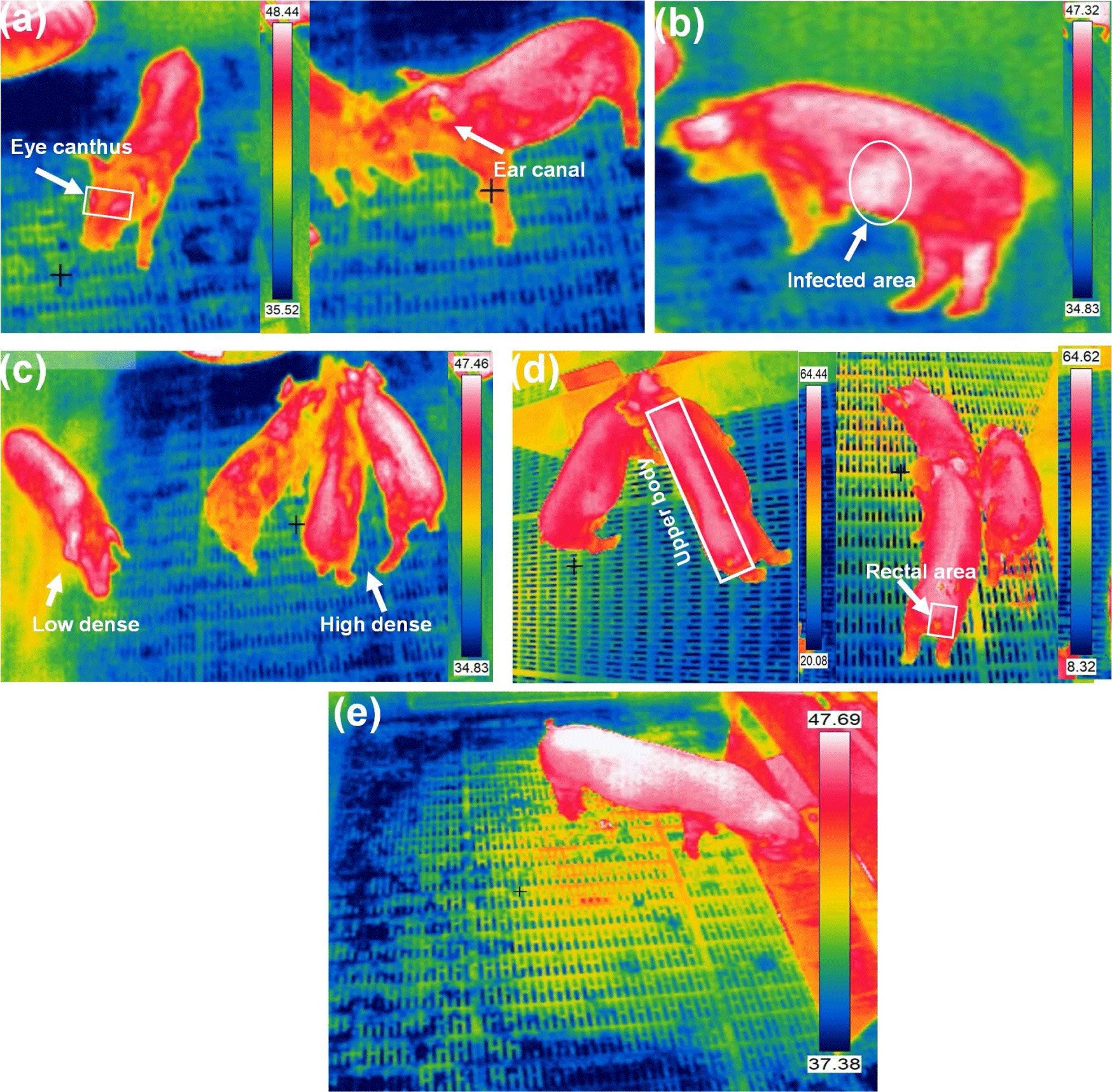
A precise thermal camera accurately predicted temperatures, with the ear canal, outer ear, and perianal area equally suitable for this purpose. Uncooled IRT offers a promising method for quickly screening pig body temperatures. However, accuracy can be compromised by variations in body surface emissivity caused by skin blemishes or differences between body parts. To solve this issue, Jiao and Jiao [47] introduced a rotatable IR filter into the optical path of a smartphone-based uncooled IR detector. This filter allows for the acquisition of IR radiation at different wavelengths, enabling the creation of a temperature measurement equation that mitigates the impact of emissivity variations. Building on this approach, they developed a smartphone sensor equipped with an uncooled IRT system featuring wavelength alteration. This sensor facilitates rapid and precise temperature measurements in group settings, offering valuable support for diagnosing physiological abnormalities and diseases in pigs. Zhang et al. [48] demonstrated that body surface emissivity in pigs fluctuates based on growth stage, sex, species, and specific body regions, spanning a range from 0.92 to 0.97. However, there is inconsistency in the applied research community regarding the emissivity values employed for pig body surfaces. Often, the same emissivity is applied uniformly across different pig parts, potentially resulting in errors in IRT temperature measurements and, consequently, inaccurate research outcomes. In growing pigs, automated feeding systems tracked individual feed intake and weight. Cook et al. [49] used IR imaging during feeding to capture thermal data from the dorsal surfaces of pigs. Fasting periods at 35, 65, and 105 kg showed a decrease in maximum surface temperature of 0.28°C and an average surface temperature of 0.48°C. These temperatures correlated negatively with feed intake and positively with residual gain variables. Residual feed intake negatively correlated with temperatures, while residual gain and intake and gain positively correlated.
The precision of IR temperature measurements is significantly influenced by both distance and viewing angle. Wang et al. [50] assessed the horizontal distance, camera height, pig height, and view angle between the object on IR temperature measurements. Their proposed mathematical model, using response surface methodology, shows a correction method reducing the mean relative error from −4.64% to −0.70%. This correction significantly enhances skin temperature monitoring in commercial pig farms. Chung et al. [51] introduced non-contact IRT for measuring various body areas and established a strong linear correlation with rectal temperature. They noted that areas with fewer piglet hairs allowed for more accurate skin temperature measurements, as piglet hair tends to cool the skin when using IRT. Soerensen et al. [30] assessed IR temperature measurement technology for determining pig body temperature and understanding the relationship between skin, environment, and body temperature. They identified optimal skin position as highly correlated with rectal temperature. Emissivity values were found to be 0.946, 0.975, and 0.978 for the hairy shoulder, udder, and ear base, respectively. In skin areas without blood perfusion, emissivity tended to be lower compared with perfused areas (p = 0.06). The study validated using a human skin emissivity value of 0.98 for IR measurements on sows but found that hairy or non-perfused skin areas have lower emissivity values.
Body temperature monitoring in animals, specifically in pigs, is a critical aspect of veterinary and agricultural research. This temperature measurement can provide valuable insights into the health and well-being of these animals. However, the choice of measurement method and location is pivotal for accurate and reliable results. Nevertheless, the variability in body surface emissivity values, as demonstrated by Zhang et al. [39], highlights a challenge in the field. Inconsistencies in the application of emissivity values across different pig body regions can lead to errors in IRT measurements, potentially affecting research outcomes. Table 2 summarizes various studies on pig body temperature measurements using the IRT imaging technique.
No. | Camera | Application | Measuring part | Performance | References |
---|---|---|---|---|---|
1 | Flir T650sc Fluke TiS45 | Temperature monitoring | Ear canal, eye canthus, outer ear, perianal area | R2 = 0.49–0.54 Error = ±0.2°C, ±0.3°C | Stukelj et al. [46] |
2 | Rotatable infrared filter | Temperature screening | Body surface | Error = ±0.3°C | Jiao and Jiao [47] |
3 | FLIR SC620 | Infrared emissivity | Body, trotter, and eye | R2 = 0.89–0.93 R2 = 0.94–0.97 | Zhang et al. [48] |
4 | Hikvision TB-1217A-3’PA | Body surface | Skin temperature | Error = 4.63% | Wang et al. [50] |
5 | Allflex PNL4060 RFID | Temperature screening | Dorsal surface | Error = −0.28°C to −0.48°C | Cook et al. [49] |
6 | ThermaCAM E2 FLIR | Temperature monitoring | Auricle, ear canal, frontoparietal, infraorbital, axillar, dorsum, abdomen, anus regions | R2 = 0.19–0.34 MSE= 0.12–0.17 | Chung et al. [51] |
In pig farming, IRT serves as a non-invasive and non-contact technology for early disease detection and tracking. By measuring the IR radiation emitted from pigs’ bodies, IRT can identify elevated body temperatures, which often signal the presence of illnesses such as swine fever or respiratory infections. Regular IRT scans enable farmers to pinpoint sick individuals or groups, facilitating prompt intervention and treatment. Moreover, IRT aids in monitoring respiratory health and detecting stress, both of which can compromise pigs’ well-being and increase disease susceptibility. Additionally, it helps in tracking disease spread within the farm, segregating infected pigs, and evaluating vaccination effectiveness. This technology not only minimizes human contact with animals but also allows for data analysis, aiding in trend prediction and enhancing overall farm management and disease prevention strategies. However, it should complement, not replace, conventional veterinary care, and specialized knowledge is essential for accurate interpretation and effective disease management. Fig. 4 shows different pig diseases detection and ROI tracking using IRT imaging system.
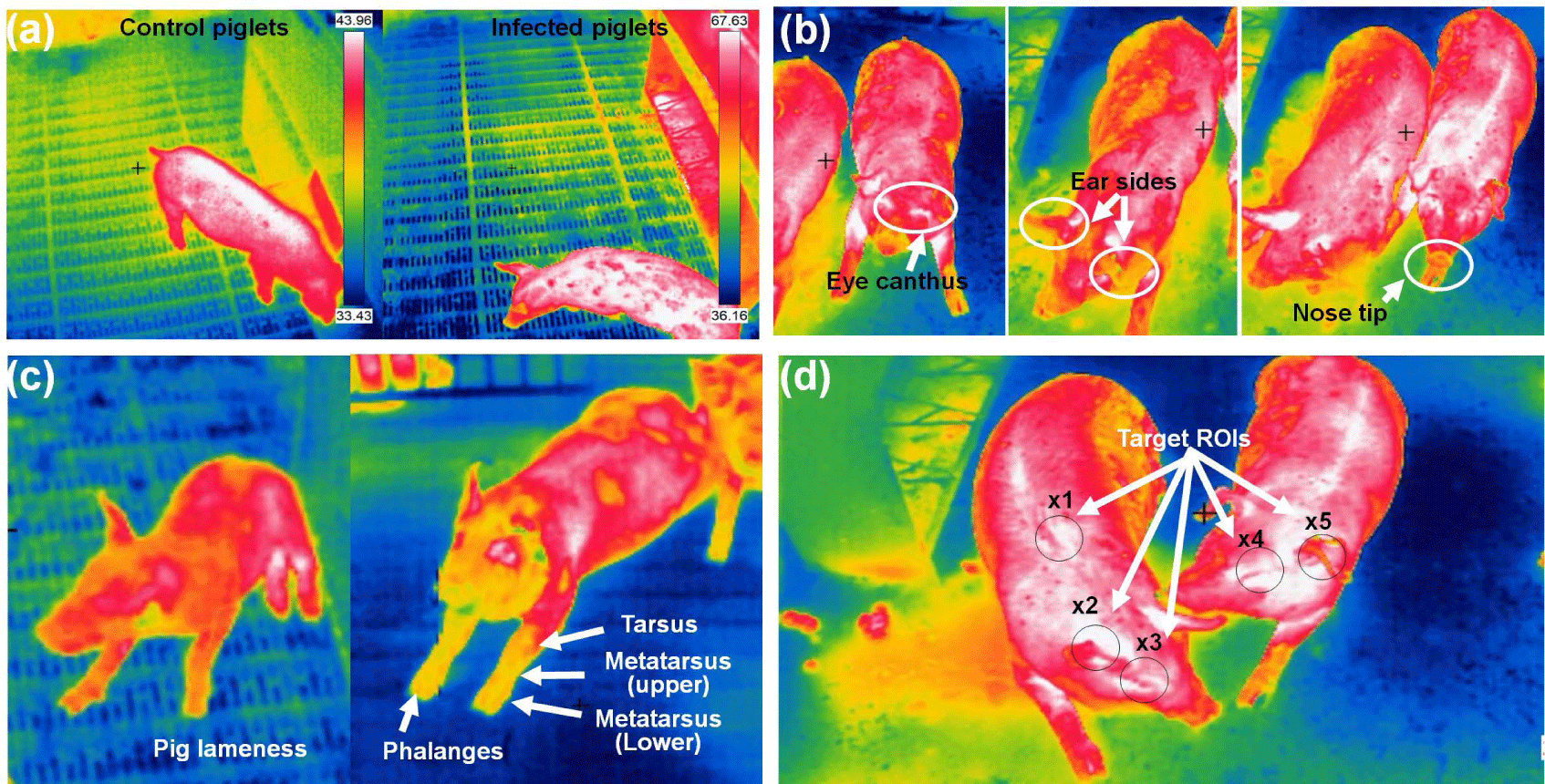
Islam et al. [52] aimed to explore the early detection of disease signs based on changes in pig body temperature. The researchers conducted three inoculations and monitored the piglet body temperatures at specific intervals: 0, 2, 6, 12, and 24 hours post-inoculation and, subsequently, every 24 hours for a period of up to 14 days. In the case of diseased infected piglets, body temperature increased significantly at 24 hours, reaching a peak at 72 hours (p < 0.05), and remained elevated after that. The findings demonstrated that thermal imaging can serve as a quick and efficient method for the early detection of disease signs in pigs. Jorquera-Chavez et al. [53] utilized computer-based methods to measure temperature changes in the eye and ear-base temperatures, heart rate, and respiration rate in pigs using both thermal IR and conventional images. Sick pigs showed higher increases in both eye and ear-base temperatures compared with healthy pigs. The average eye temperature in the sick group rose by 8.1°C between 7:30 h and 14:30 h, while the healthy group increased by only 1.4°C in the same period. The ear-base temperature remained consistently 0.8°C–1.8°C higher in sick pigs across the four measured time points. The findings revealed distinct variations in eye temperature, ear-base temperature, and heart rate between healthy and sick pigs during the observation period. This could aid the potential of computer vision techniques to offer valuable data on physiological changes, enabling early detection of respiratory infections in pigs.
Amezcua et al. [54] used a handheld FLIR T300 camera and captured thermal images of sow legs with a second repeated scan. The study showed IRT as a sensitive and non-invasive means to distinguish between lame and non-lame sows. Upon converting surface temperature distribution into visual images, IRT, previously applied in equine medicine for lameness detection, demonstrated its sensitivity. Images were taken at close range (0.5–0.8 meters) and a 0° angle for both legs while the sow stood. Although not diagnostic for specific conditions, IRT effectively monitors surface blood flow in some animals, indicating changes in temperature related to physiological and painful states. Despite variations in mean temperature, the study emphasized the effectiveness of differentiating between lame and non-lame sows using IRT imaging. IR imaging emerged as a rapid and straightforward technique for assessing temperature distribution on pig heads. Siewert et al. [17] suggested that using IR imaging and a specific ROI method allows for the early detection of elevated body temperatures in pigs (> 39.5°C) with approximately 86% sensitivity and 85% specificity. Noise is minimized by averaging temperatures within these regions. However, because of the limited number of animals in the study, caution is advised. Further measurements are recommended despite notable differences between the test and control groups. Kammersgaard et al. [55] explored the use of IRT to non-invasively estimate body temperature and evaluate the thermal condition of newborn pigs. They studied 91 neonatal pigs in loose farrowing pens with floor heating at 34°C, exposed to three different ambient temperatures (15°C, 20°C, and 25°C). Utilizing 1,695 thermogram and 915 rectal temperature measurements, they analyzed the relationship between surface temperatures and rectal temperatures in these conditions. The study derived maximum, minimum, and average body and ear surface temperatures from these thermogram. Statistical analysis revealed a strong correlation ranging between 0.82 and 0.85 between body and ear temperatures and rectal temperature. Additionally, they observed a slightly higher maximum temperature when capturing a thermogram from the side of the pig compared with the back. Table 3 summarizes various studies on pig diseases and tracking using the IRT imaging technique.
No. | Camera | Application | Measuring part | Performance | References |
---|---|---|---|---|---|
1 | Thermo Tracer TH5104R | Disease diagnosis | Head, body, and tail region | p <0.05 | Islam et al. [52] |
FLIR AX8 | Disease diagnosis | Eye, ear, nose | LSD 0.6–0.84 | Jorquera-Chavez et al. [53] | |
3 | FLIR T300C | Disease diagnosis | Foot | - | Amezcua et al. [54] |
4 | VarioCAM IR Flex CAM R2 | Health monitoring | Head | Error= ±1.5°C to ± 2.0°C | Siewert et al. [17] |
5 | Testo T880-3 | Health monitoring | Ear, head, armpit | R2 = 0.82–0.85 | Kammersgaard et al. [55] |
Knizkova et al. [56] explored thermal imaging cameras for diagnosing orthopedic diseases in livestock and poultry. They highlighted the role of IR thermal imaging in animal breeding, aiding thermoregulation for improved welfare and assisting in lactation. However, the use of IRT requires consideration of limiting factors such as sunlight, moisture, dirt, and weather conditions. Bleul et al. [57] evaluated the effectiveness of a low-cost, handheld IRT camera connected to a smartphone for disease detection in farm animals. The findings indicated that the camera’s performance was limited, resulting in significant measurement variations. Using a higher-quality thermal camera for future experiments was recommended. Additionally, the respiratory rate analysis based on non-radiometric IR videos might be influenced by fluctuations in lighting conditions, potentially affecting measurement sensitivity.
IRT was explored as a tool for detecting diseases and tracking pigs on livestock farms. However, several constraints are associated with its use in this context, such as the requirement for calibrated and accurate equipment, the complexity of the operational method, and the influence of environmental factors such as sunlight, wind, humidity, and dirt [58,59]. The distance between the thermal camera and the animal is another factor affecting temperature measurement accuracy [39]. Additionally, varying results in studies may stem from inadequate equipment, differing knowledge about its reliable operation, and site-specific factors not considered in assessments [60]. Further research and development are necessary to address these constraints and enhance the diagnostic value of IRT for pig disease detection and tracking. IRT holds promise for improving pig health and disease management in the agricultural sector. While IRT shows promise for early disease detection, it is essential to pair it with traditional veterinary care and interpret results using specialized knowledge [58].
Unhealthy pigs may exhibit various non-specific behavioral changes, such as reduced food intake and typical sickness behavior. Additionally, certain diseases can lead to specific signs that aid in their diagnosis and intervention. These signs include lameness in foot rot, coughing during respiratory infections, high-pitched squealing in edema disease, and scratching in pruritic mange. Some common early signs of an unhealthy pig include reduced activity, increased resting time, separation from other pigs, drooping ears while lying down, huddling, shivering, walking with an arched back and drooping tail, and exhibiting labored breathing, thumping, or coughing while sitting [61].
In a study by Boileau et al. [62], 1,284 thermal images from 46 pigs engaged in a controlled agonistic encounter were analyzed. The thermal camera is positioned approximately 5 meters above at an angle of 30 degrees. An average temperature of 31.6°C ± 0.3 with a coefficient of variation (CV) of 2.75 ± 0.03% was found using the ROIs in IRT images during different behavior patterns. The findings indicate that the dorsal plane in pigs is effective for detecting surface temperature changes in response to stress. Utilizing the dorsal plane instead of the eye or ear for IRT imaging simplifies its application in research and practical settings, offering a non-invasive method to gauge additional welfare indicators. Costa et al. [63] evaluated the relationship between pig activity and environmental factors in a mechanically ventilated barn for 350 fattening pigs. The study, conducted over 30 days, employed an IR-sensitive CCD camera mounted 5 meters above the floor. The analysis revealed a significant correlation between pig activity and ventilation rate (p < 0.01), temperature (p < 0.01), and humidity (p < 0.001). Fluctuations in ventilation, followed by temperature and humidity changes, significantly impacted the pig behavior patterns, affecting their distribution within the pen. In a study by Garrido-Izard et al. [64], the behavior of 30 Landrace pigs over an 81-day fattening period was explored by integrating their thermal and food intake patterns. The ear skin temperatures revealed a clear relationship between mean temperature and its variability (r = 0.83), distinguishing pigs with distinct thermal patterns. Those with higher temperatures displayed less variability, and vice versa. Analysis of feeding station data demonstrated consistent relationships among frequency, size, and duration parameters, revealing significant differences in the feeding strategies of pigs.
Courville et al. [65] developed a learning algorithm to identify mounting behavior in pigs using visible light image data features. They analyzed recorded videos, isolating frames displaying mounting behavior as positive dataset samples, while images depicting inter-pig adhesion and separated pigs were categorized as negative samples. Viazzi et al. [66] developed an automated system using image processing to detect aggressive behavior in pigs continuously. With a dataset containing 150 aggressive interaction episodes and 150 non-aggressive episodes from video recordings, they used linear discriminant analysis (LDA) based on motion intensity and occupation index, achieving successful aggression classification. Through cross-validation, the system attained 89.0% accuracy, with 88.7% sensitivity and 89.3% specificity. Lee et al. [67] introduced an economical and non-invasive prototype system for automatic monitoring in commercial pig pens. Their system utilized a Kinect depth sensor to identify aggressive behavior among pigs. This system employed a detection and classification module, which used a two-step approach with binary-classifier support vector machines (SVMs) to identify and categorize aggressive actions, including head-to-head or body knocking and chasing. Their experimental findings demonstrated the effectiveness of this method in cost efficiency and accuracy, with detection and classification rates of 95.7% and 90.2%, respectively. Detecting pig behavior is crucial for effectively identifying abnormalities, including diseases and abnormal activities, thereby safeguarding the overall health and welfare of pigs [68].
Pig behavior plays a crucial role in the productivity, health, and welfare of pig farming [69]. These behavior patterns are essential for timely diagnosis and intervention. The ability to continuously monitor and detect pig behavior contributes not only to the early identification of diseases and abnormalities but also plays a crucial role in ensuring the overall health and welfare of pigs in commercial farming settings [70]. Both image-based recognition and video-based approaches, aimed at extracting spatial–temporal features, contribute to achieving superior performance in this context [71]. Nevertheless, numerous challenges persist in pig behavior recognition. Addressing issues related to excessive crowding and captivity among pigs, leading to the loss of major behavioral characteristics, is a significant challenge [69,72]. Enhancing the accuracy of recognizing similar and multi-behaviors in complex environments remains a priority [73]. Additionally, the fast-moving pigs result in body deformation and drift, further complicating the task of behavior recognition [71].
Remote health monitoring in pig farming has gained prominence in animal welfare and farm management by capturing thermal images from a distance, revealing temperature variations indicative of pig health and well-being [74]. This innovative technology enables early disease detection, even before visible symptoms appear, reducing the risk of disease spread within the farm. Additionally, it assesses comfort levels, identifies opportunities to optimize feed efficiency, and offers automated, continuous monitoring [70]. Data analysis software detects anomalies and trends, allowing for comprehensive pig health assessments, while automated alerts prompt timely action [75]. This approach contributes to better pig welfare, minimizes medication use, lowers mortality rates, and improves feed efficiency, all while integrating thermal data with other farm metrics for a complete performance overview. Oh et al. [76] explored IRT as a potential diagnostic tool for early-stage ASF detection in pigs. They observed that ASF-infected pigs exhibited an increase in skin temperature, rising from 35.0°C to 38.5°C between 2 and 3 days post-infection. The IRT approach holds potential for pig farms at high risk of ASF outbreaks. While the study focused on controlled conditions, further research is needed to determine optimal skin and ambient temperatures across different age groups. This will ensure the effective application of the IRT technique in real-world pig farm settings.
Remote health monitoring using thermal imaging in pig farming offers a non-invasive and effective method to monitor pig health, improve welfare, and optimize farm management practices. This technology has the potential to revolutionize how farmers care for their pigs, ensuring better health outcomes and increased efficiency in the industry [45]. Integrating IRT into sustainable pig production offers multifaceted benefits. Through real-time health monitoring, IRT enables early detection of anomalies and promotes timely intervention, reducing antibiotic use. It enhances energy efficiency by optimizing temperature control and minimizing heat loss, contributing to environmentally friendly operations. IRT also aids in resource management, precision farming, and stress reduction, ultimately improving animal welfare and productivity. However, successful implementation requires addressing initial costs, maintenance, and ethical considerations. Overall, IRT empowers sustainable pig farming by fostering efficient, eco-friendly practices while ensuring animal well-being.
DEEP LEARNING ALGORITHMS FOR INFRARED THERMOGRAPHY APPLICATION
The convergence of deep learning and IRT has ushered in a new era of temperature-based analysis. Inspired by the human brain, deep learning algorithms have emerged as potent tools for unlocking insights within thermal images. These algorithms are reshaping thermal data interpretation, enhancing accuracy and efficiency across diverse fields. With their multi-layered neural networks, deep learning excels at uncovering intricate patterns in complex datasets. In IRT, these algorithms provide a transformative approach, revealing subtle thermal cues that evade human detection. This capacity enhances anomaly detection, behavior prediction, and informed decision-making.
In recent times, deep learning and IRT have held immense promise in various domains. From predicting stress levels in animals to disease detection, disease symptoms, behavior patterns, daily activity monitoring, food habit analysis, etc., the applications are extensive and far-reaching. Fig. 5 shows deep learning algorithm application along with IRT imaging to detect pig body parts, diseases monitoring, tracking, and real-time application. Küster et al. [77] introduced an automated approach for evaluating thermal images of neonatal piglets to assess body temperature. This method integrates climate sensor data with thermal images, considering surface emissivity and camera-to-object distances for accuracy. It utilizes a convolutional deep neural network (CDNN) (YOLOv3-SPP) to identify key areas in piglet images, distinguishing them from the background using the Otsu algorithm. YOLOv3-SPP achieved precise detection of trained body parts with 0.78 precision, 0.98 recall, and a mAP of 0.97. The regression method exhibited a strong correlation of body temperature estimation with an R2 = 0.774. Lu et al. [78] proposed an automated method for extracting ear base temperature from top-view thermal images of piglets. They developed a SVM classifier to identify piglet head parts. Using the head contour shape, they located two ear base points. Ear base temperatures were determined by extracting the two highest temperatures within circles centered on these points. The approach achieved high accuracy, with less than a 0.4°C inaccuracy for 97% and 98% of left and right ear bases, respectively. However, variations in head and ear positions in different photos led to occasional incorrect ear temperature readings. Wang et al. [79] introduced the SwinEAR technique for measuring pig IR temperature. This method employs pig ear segmentation and multi-variable calibration. The study examined environmental factors, such as temperature, humidity, detector drift, target-object distance, and emissivity, affecting the IR temperature model. The findings demonstrated a substantial enhancement in temperature measurement accuracy through the IR temperature calibration model. The mean absolute error dropped from 0.89°C to 0.29°C compared with the built-in IR thermal imager module.
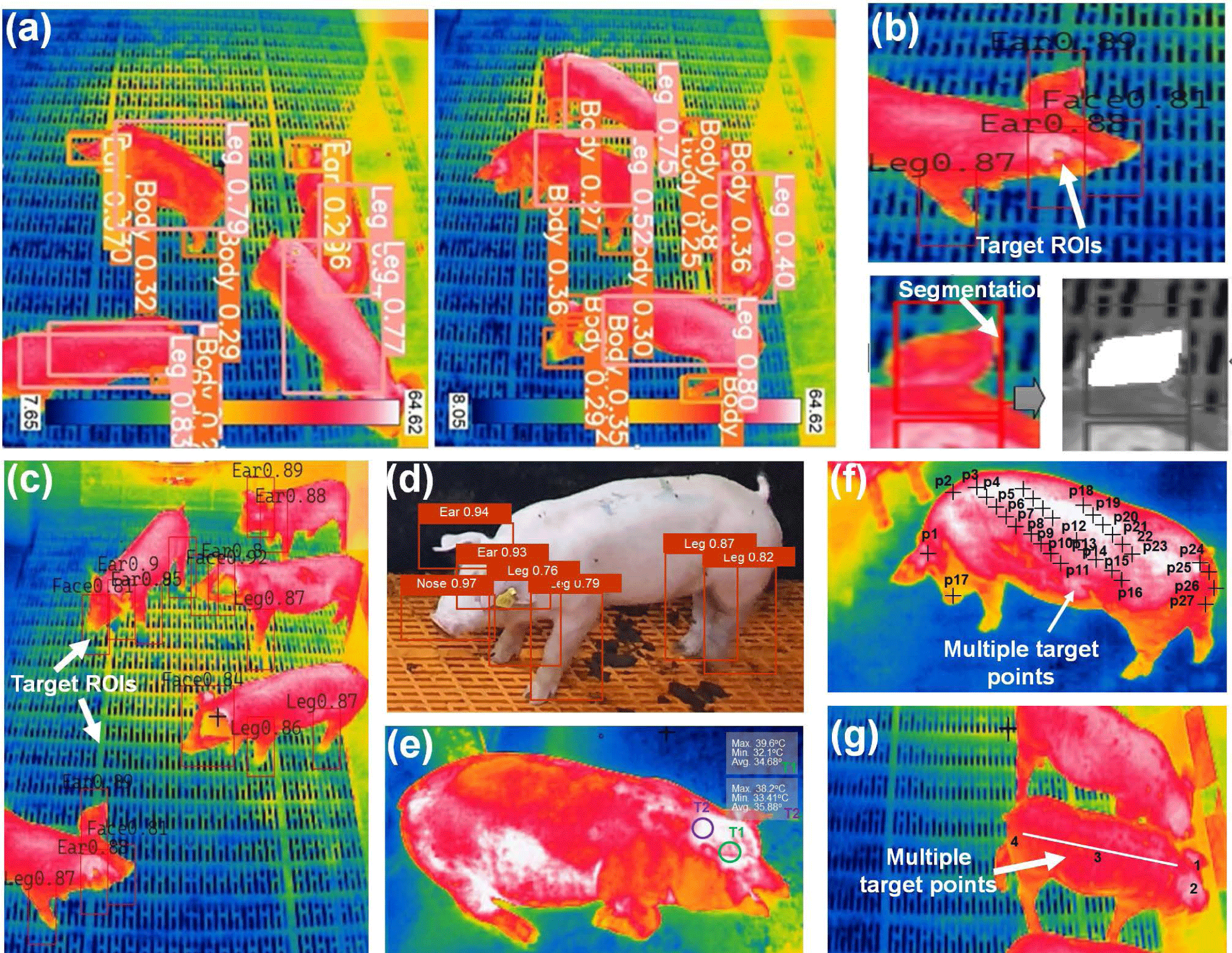
Despite this improvement, environmental factors may still impact temperature measurement precision. Jia et al. [80] introduced a non-contact IRT approach for measuring skin temperature in specific ROIs but showed limitations in predicting internal temperature reliably. They examined the impact of air temperature and humidity on ROI temperature and utilized this data to develop a prediction model employing backpropagate neural net (BPNN), random forest (RF), and support vector regression (SVR) algorithms. When environmental factors were accounted for, SVR yielded the best results, achieving a maximum error of 0.478°C, minimum error of 0.124°C, and MSE of 0.159°C. Future research should expand dataset diversity, encompassing varied seasons to capture temperature and humidity fluctuations more comprehensively. Modeling efforts should be grounded in broader datasets, enhancing year-round applicability. As pig farm setups differ, investigations should dynamically adjust environmental factors to accommodate specific circumstances, considering varying degrees of influence.
Subsequent difference imaging significantly reduces variable offset errors present in each IRT image. Xie et al. [81] proposed an IRT and visible imaging (VI) method to develop a deep learning-based fusion technique for measuring temperatures across various pig body parts. They identified IRT measurement regions on pig skin and created an automatic detection method using VI and YOLOv4 models for body part recognition. Utilizing semantic segmentation, they extracted pig body surface temperatures through the fusion approach. Temperatures ranged from 33.6°C on the nose to higher values on the tail root, ear root, anal, and forehead, with means of 36.2°C, 37.0°C, 37.1°C, and 36.0°C, respectively. Significant disparities in minimum temperatures were observed between automatic and manual temperature measurements. Despite this, the innovative model shows potential for effective monitoring of abnormal body temperatures. Xiong et al. [82] introduced IRT and machine learning to predict neonatal piglet rectal temperature. They collected data on rectal temperatures and thermal ear images at various time points after birth. The average rectal temperature decreased by 5.1°C post-birth, hitting a low of 33.6°C after around 30 minutes, significantly below the birth temperature of 38.7 ± 0.8°C. Evaluating the model, they classified data by piglet gender, initial weight, and environmental factors (room temperature, humidity, wet bulb temperature). The Lasso regressor machine-learning model outperformed direct regression models with a prediction standard error of about 1.5°C, enhancing accuracy by around 0.2°C. However, potential factors beyond consideration could lead to unaccounted variations among individual pigs within each litter.
Caldara et al. [83] explored the impact of the environment on newborn piglet weight using IRT imaging and variance analysis. Thermal images were analyzed for multiple point-based temperature detection. Piglet heat loss was studied shortly after birth and emphasized the importance of maintaining a comfortable birthing environment. After 15 minutes from birth, the body surface temperature of piglets showed significant negative correlations (−0.824 and −0.815) with the ambient temperature and humidity. The study highlighted significant thermal exchange between piglets and the farrowing crate floor, potentially impacting their heat loss and overall performance. IRT images indicated that piglets with lower birth weights experienced more temperature drop within their first hour of life compared with others. A similar study by Jensen et al. [84] also found that the sow has a strong effect on piglet rectal temperature at birth, but this effect retreated by 2 hours after birth. This suggests that IRT may play a role in monitoring piglets’ thermoregulation in the first few hours after birth. de Oliveira et al. [85] developed a fuzzy model to predict respiratory frequency and rectal temperature in pigs subjected to acute and chronic heat stress. The model used air dry bulb temperature and average surface temperature as input variables and respiratory frequency (movements/minute) and rectal temperature as output variables. The Mamdani inference method was used with six rules and the center of gravity defuzzification technique. The model was evaluated using experimental data and showed satisfactory efficiency in both respiratory frequency and rectal temperature determination, with R2 values of 0.997 and 0.951 for acute stress and 0.993 and 0.964 for chronic heat stress, respectively.
Tabuaciri et al. [86] suggested an effective regression method for diagnosing hypothermic piglets and a practical alternative for assessing core body temperature in newborns. Emphasizing immediate attention for all shivering piglets, particular focus should be directed toward those exhibiting low IR body temperatures within 24 hours of birth. The ear-tip temperatures remained consistently low (averaging 34.2 to 34.4°C), and even in warm summer conditions with heat lamps, 9% of piglets displayed shivering, with higher rates in cooler months. The correlations between ear bases and tips (0.33–0.80) were weaker, as extremity temperatures tend to drop in cold conditions to conserve core body heat. da Fonseca et al. [87] proposed a predictive model for assessing stress in piglets across various conditions such as pain, cold/heat, hunger, and thirst using an IRT camera placed at a distance of 0.30 cm from the measurement site. By considering minimum and maximum IR skin temperatures along with piglet sex, they constructed a machine learning and paraconsistent logic model. The decision tree model demonstrated robust accuracy, detecting cold and thirst stress with precisions of 100% and 91%, respectively. Additionally, hunger stress prediction achieved an accuracy of 86% using only minimum temperature. The model performance in predicting pain stress (< 50%) was notably limited or potentially unattainable.
Table 4 summarizes various studies on deep learning algorithms for IRT applications. Graciano et al. [24] used IRT imaging to detect edema in pigs on a farm, showcasing its efficacy in early arthritis diagnosis. The need for automatic pig disease, behavior, and size assessment has increased due to extensive phenotyping. Although automated tracking in labs has proven effective, challenges arise when dealing with diverse agricultural settings and large animal groups [88]. By harnessing the prowess of deep learning, IRT can transcend its traditional boundaries and unlock new dimensions of understanding temperature-based phenomena. The fusion of deep learning algorithms with IRT presents compelling advantages such as heightened accuracy, efficient data analysis, versatility, and unbiased interpretation. However, challenges encompass data dependency, computational demands, interpretability concerns, overfitting risks, and the need for continuous learning. Striking a balance between these benefits and drawbacks is crucial as this synergy offers remarkable potential to elevate temperature-based analysis across diverse fields.
No. | Camera | Measuring part | Method used | Performance | References |
---|---|---|---|---|---|
1 | FLIROnePro | Head, back, back end | YOLOv3-SPP + Otsu | R2 = 0.774 | Küster et al. [77] |
2 | Fluke TI32 | Head, ear | SVM | Acc: 0.97–0.98 | Lu et al. [78] |
3 | MAG64AI | Ear | Semantic segmentation | Acc: 0.921 | Wang et al. [79] |
4 | Fluke Ti-300 | Skin | BPNN, RF, SVR | MSE = 0.159°C | Jia et al. [80] |
5 | Fotric 287-L20 | Body surface | Semantic segmentation and YOLOv4 | mAP = 94.20 | Xie et al. [81] |
6 | Fluke Ti55 | Body surface | Fuzzy model | R2 = 0.95–0.99 | Oliveira et al. [85] |
7 | FLIR T450sc | Back, head, ears | Regression models | Error= 1.5°C–1.7°C | Xiong et al. [82] |
8 | Testo 876-1 | Body surface | Variance analysis | R2 = 0.81–0.82 | Caldara et al. [83] |
9 | Flir ThermaCam | Body surface | Regression method | R2 = 0.38–0.80 | Tabuaciri et al. [86] |
10 | Testo 876-1 | Skin | Decision tree | Acc: 0.91–0.99 | da Fonseca et al. [87] |
COMMERCIAL UTILIZATION OF INFRARED THERMOGRAPHY IN PIG HEALTH MONITORING
The economic prospects of monitoring pig diseases and crush symptoms hold substantial value within the global pig industry, which is a multi-billion-dollar sector. The demand for technologies that enhance pig health and productivity is substantial. Profitable avenues include creating and selling monitoring devices, offering data interpretation services to farmers, and establishing data analytics companies. In recent years, pig farming has seen the emergence of various innovative commercial products aimed at improving efficiency, animal welfare, and overall farm management. These products cater to different aspects of pig farming, ranging from health monitoring to environmental control.
InfiRay (IRay Technology, Yantai, China) concentrates on developing IR thermal imaging technologies and manufacturing relevant products with completely independent intellectual property rights. InfiRay thermal cameras feature non-contact measurement, accurate measurement, and quick measurement of multiple points. They can effectively improve the measurement efficiency and avoid causing animal discomfort, which is suitable for temperature measurement of scaled farms. For large farms, the AT300 thermal cameras can be installed on robots on suspended rails for patrol temperature measurement of all animals. ATS300 fever screening systems have a measurement distance of 1–5 m with a measurement accuracy of ±0.3°C and flux of 100 objects/min.
The iButton® temperature/humidity logger (DS1923, Maxim Integrated Product, Dallas, TX, USA) is a durable and self-contained system that measures and records temperature and/or humidity in a secure memory section. Users can set the recording interval, with storage capacity for either 8192 8-bit readings or 4096 16-bit readings. The intervals can range from 1 second to 273 hours. It also has 512 bytes of SRAM for specific data and 64 bytes for calibration. Data collection missions can be scheduled to start immediately, after a set delay, or triggered by a temperature alarm. Password protection ensures memory and control access. To measure pig ear temperature, iButtons (DS1923) can be used in farm conditions with a resolution of 0.5°C and a reported accuracy of ±0.5°C[89], as shown in Fig. 6. iButton can be fastened to the ID tag on the ear of each animal in such a way that it was in contact with the inner part of the ear.

Various implantable devices and evolving wearables offer temperature-sensing solutions. Implants, including rumen-placed ones in cattle and microchips in pigs, monitor body temperature. Some microchips enable easy reading via radio telemetry. Comparably, these devices align well with rectal temperature, often measuring around 1°C lower. While diverse implantable options exist, they necessitate invasive procedures. An innovative wearable, positioned on cattle legs, closely gauges body surface temperature and exhibits a strong correlation with rectal readings [90]. In adult pigs, a self-sustaining pacemaker for cardiac pacing was successfully implanted, utilizing energy harvested from the pig’s own heartbeat [91]. Pigs, given their anatomical similarity to humans, serve as valuable models for testing medical devices and treatments before human application. A new model has been effectively adopted in the development and assessment of neural prosthetic devices [92]. These advancements collectively contribute to the modernization and sustainability of pig farming, meeting the demands of a growing global population while ensuring ethical and responsible farming practices. Joosen et al. [93] employed a torso-mounted sensor to measure a pig’s heart rate, while Suresh et al. [94] utilized recommended pulse sensors for their automatic cattle health monitoring system. Antanaitis et al. [95] created an experimental device based on piezoelectric and electrocardiogram (ECG) sensors to measure both respiration and heart rates. These methods involve close contact with the skin of animals and are generally considered high-stress techniques, which should be taken into account. Wearable devices have also been developed for tracking and tracing animals, such as wireless ear tags that can monitor body temperature, physical signs, and pig mating cycle data [96].
FUTURE PERSPECTIVES AND CHALLENGES
As the field of pig farming continues to evolve, advanced sensors and computer vision-based thermal imaging techniques hold promising potential for enhancing pig health and management. These innovations offer valuable insights into disease detection, piglet crush monitoring, and overall animal well-being [71]. However, several key perspectives and challenges shape the future trajectory of this technology.
Key Perspectives:
-
Enhanced Disease Detection: Advanced sensors and thermal imaging can provide real-time data on pig body temperatures, aiding in the early detection of diseases. This proactive approach enables timely intervention and disease prevention, contributing to healthier herds and improved farm productivity [58].
-
Automated Symptom Monitoring: Computer vision-based techniques can automate the monitoring of diseases and behavior patterns, ensuring rapid response to any distress signals. This can lead to more efficient care and reduced stress for animals [71].
-
Remote Monitoring: Remote access to thermal imaging data allows farmers to monitor their herds from afar, facilitating prompt decision-making and reducing the need for constant on-site presence [97].
-
Innovative Management Practices: Data-driven insights based on IRT technologies can drive innovative management practices, promoting animal welfare, sustainable farming, and resource optimization [98].
-
Precision Livestock Farming: The integration of advanced sensors and computer vision with data analytics offers precision livestock farming. Farmers can gain deeper insights into pig behavior, health trends, and environmental conditions, enabling optimized management strategies [92].
Challenges:
-
Accuracy and Reliability: Ensuring the accuracy and reliability of IRT sensor measurements and thermal imaging interpretations is crucial for effective disease detection and symptom monitoring [73,82].
-
Data Management and Analysis: Handling an influx of IRT data generated by advanced sensors and thermal imaging requires robust data management and sophisticated analytical tools to extract meaningful insights [41].
-
Implementation Costs: The initial investment for adopting advanced sensors and computer vision systems may pose financial challenges for smaller pig farms [32,57].
-
Animal Behavior Variability: Pig behavior can vary widely, and some animals might react differently to sensor attachment or thermal imaging, impacting data collection consistency [71,73].
-
Ethical and Privacy Concerns: The use of technology for monitoring animals raises ethical and privacy concerns that need careful consideration, particularly regarding data collection and sharing.
-
Integration and Training and Regulatory Considerations: Incorporating IRT technologies into current farm operations and training farm staff for proficient data interpretation can pose complexities. It is crucial to adhere to pertinent regulations regarding animal welfare, data privacy, and technology usage standards.
The integration of advanced sensors and computer vision-based thermal imaging holds significant promise for pig disease and piglet crush symptom monitoring. While challenges exist, addressing these issues can lead to transformative advancements in pig farming, benefiting both animal health and farm productivity. It requires a collaborative effort between technology developers, researchers, and the farming community to realize the potential of these innovations fully.
CONCLUSION
In pig farming, the integration of advanced sensors and computer vision-based thermal imaging techniques represents a remarkable leap forward in disease detection and crush symptom monitoring. This synergy of technology offers a proactive and data-driven approach to pig health management, promising improved animal well-being and enhanced farm productivity. While challenges such as data accuracy, ethical considerations, and implementation costs remain, they serve as stepping-stones toward refining these innovations for practical application. As we stand at the cusp of a new era in precision livestock farming, the potential benefits of these advancements underscore a path toward more efficient, humane, and sustainable pig farming practices. Through collaboration, research, and responsible implementation, the future holds the promise of healthier herds, reduced disease impact, and elevated standards of care, all made possible by the fusion of advanced sensors and cutting-edge computer vision-based thermal imaging techniques.