INTRODUCTION
The global human population is estimated to reach 9 billion by 2030 [1]. In South Korea, livestock face several issues such as disease, animal welfare, increasing population age, stinking, and excrement treatment. The Paris Agreement, which encourages countries to submit long-term low greenhouse gas emission development strategies [2], is another issue that must be solved to achieve goals in the livestock sector [3]. These problems are not limited to South Korea but are becoming a reality in livestock farming worldwide.
A smart livestock farm (SLF, also known as Precision livestock farm [PLF]) is defined as a livestock farm where information and communication technology (ICT) systems are used [4]. The SLF produces more data than previously measured information at livestock sites, and SLFs are constructed using these data. Based on the measured data, decisions could be made for all processes, such as stocking, breeding, shipping, and evaluation. Before the smart livestock technology was introduced into the livestock farm, workers manually recorded data in an analog form and subsequently digitized them into a simple datasheet. Moreover, the post-slaughter grading data are less compatible because they existed between the farm and the Institute for Animal Products Quality Evaluation (KAPE), respectively, which limits their availability. An SLF system is an extended concept in SLF and creates new value by utilizing new data produced in SLF and data from the entire existing livestock system. When the SLF system was constructed, the data types that could be collected were increased. Previously, the data could only be recorded when an event occurred or at a specified time; however, as real-time observable data become available as phenotypic and environmental data, they are being built in the form of comprehensive big data to improve the competitiveness of the livestock industry at the individual farm and national level.
Generally, in livestock, phenotype (p) (i.e., appearance, productivity, meat quality, and disease resistance) is obtained by the summation of genetic (G) and environmental (E) values (p = G + E) [5, 6]. The development of next-generation sequencing (NGS) has led to the creation of various platforms for estimating genetic effects on phenotypes. Consequently, the data generated from SLFs have increased the complexity and diversity of phenotypes. Additionally, the expanding range of measurable environmental data enables more detailed and accurate effect estimation compared to traditional technologies. Fused data that integrate environmental and phenotypic information from SLFs with genetic data are valuable for detailed applications in breeding and specifications, as they help understand complex and organic phenotypes and environments. However, its effectiveness is limited by restrictions on data sharing and non-standardized formats. This limitation leads to other restrictions against researchers, such as restrictions on the range of projects, the supply of new technologies or farm species, and policy development or application restrictions. Therefore, promoting a recirculating environment to increase productivity, developing climate-adapted livestock, and implementing policies are necessary. In this review, we introduce a dataset from the SLF and some projects for the recirculation of SLF big data. Moreover, a data recirculation system could provide a blueprint for livestock and related industries.
PHENOTYPIC DATA IN SMART LIVESTOCK
In livestock, phenotypic data represent several phenomena such as growth rate, disease information (infection information or viral load), feed intake, average daily gain, milk production, carcass information, and carbon emissions [5]. In the pre-SLF era, phenotypic data were written manually or simply digitalized as a data sheet. With the development of SLF instruments, the types of enhanced phenotypic data, such as carbon data, have increased.
To facilitate data collection using the SLF, standardization of ICT devices and data is being carried out at the national level. Starting with the establishment of the national standard for ‘Sensor Interface for Smart Livestock’ in 2020, ‘Livestock Specification Management Device Data Collection Standards’ laid the foundation for revitalizing data utilization services by improving the compatibility and quality of livestock ICT devices until the establishment of national standards (2022-23) and the establishment of group standards for the ‘Smart Livestock Data Model’ (2023). In addition, we compiled a list of the step-by-step smart livestock technologies, devices, programs, and systems used in Korea to implement smart livestock farming (as of 2023) (Table 1).
At the beginning of SLF in South Korea, a robotic milking system (RMS, also known as an automatic milking system) was applied to dairy farms. An RMS without human involvement was first introduced in 1986 in Europe [7]. The adoption and spread of RMS in dairy farms has increased milk yield [8]. However, early models of the RMS system had some restrictions on installation and operation, such as cost, population size, and cow-teat arrangement. The RMS was developed in the form of a combination of artificial intelligenece (AI) and Internet of things (IoT)technologies. The developed RMS serves as a comprehensive SLF device capable of recording various phenotypes (e.g., milk quality, ruminating data, and somatic cell counts) in dairy farms in real time. Moreover, a combined AI-based image sensing system can be used to measure body shape, such as the height, angle, and width of the hips, by comparing real data [9].
An automated measurement system (AMS) was developed to measure carcass phenotypes and evaluate them to grade abattoirs [10]. In the past, workers were evaluated using a ruler and scale to measure the carcass directly [10]. However, as the number of slaughtered animals increases, this method becomes less efficient because of the time required for measurements [11]. There are two types of AMS, the Fat-O-Meat’er (FOM) and VCS2000 systems, which are distributed in Europe [11]. The FOM measurement system measures the lean meat percentage and fat thickness of the carcass using an ultrasonic instrument [12,13]. The VCS2000 system, another AMS instrument, uses a video-based image analysis system [14,15]. The types of phenotypes that were scanned and measured in these AMS were lean percent, back fat thickness, carcass weight, meat cut percentage, and weight [12]. Based on measured phenotype data, the KAPE evaluates animal products in abattoirs. The measured data were created as a database and uploaded to the server for the SLF system.
AI-based monitoring systems are used to measure the behavior of livestock as a phenotype. The monitoring system installed in the shed tracks the behavioral patterns of livestock 24 hours a day and records their physical characteristics, such as body temperature [16]. In the swine industry, there are challenges to improving productivity and disease issues; thus, monitoring body conditions is important for the swine industry. Ear tag sensors are useful devices for tracking swine behavioral patterns. The sensor measures the body temperature in direct contact with the tissue [17]. The tag also includes radio frequency identification (RFID), which can recognize individuals and forward the measured information to an AI-based monitoring system.
GENETIC DATA IN SMART LIVESTOCK FARMS
Genetic data are used to estimate phenotypes based on the genotypes of livestock. Previously, animal breeding used phenotypes and statistical data; however, recent animal breeding studies have used genetic data [6]. The Human Genome Project has led to the development of technologies to detect genomic sequences and identify their functions. Currently, sequencing costs have decreased to less than $ 100 per individual, which has enabled researchers to generate a wide range of genetic data [18,19]. Biological research on livestock and agriculture has been conducted to improve genetics and reduce environmental stress [20]. Therefore, it is necessary to comprehensively understand their complex interactions because various phenotypes are organically connected [20]. In the field of livestock, genetic data have been collected by many research groups worldwide, including South Korea, and shared at the national or consortium level to secure publicity. In addition, Korea is making great efforts to build a platform for producing large-scale single nucleotide polymorphism data for genetic and genome selection for paternity testing, preprocessing analysis, and breeding value estimation, and is considered a smart livestock technology (Table 1).
In South Korea, two main public bioinformatics centers operate to save bioinformatics data, including genetic, transcriptomic, and metabolic data. The Rural Development Administration of Korea operates the National Agricultural Biotechnology Information Center (NABIC) to preserve agricultural bioinformatic data, such as biosequence, transcriptome, proteome, variation, and metabolome data, to create a database. The Korea Bioinformation Center (KOBIC) is a national interministerial center that comprehensively manages domestic bio-research data and provides an advanced data research utilization environment. KOBIC has prepared a standardized registration form for collecting various bioresearch data and has efficiently collected data scattered across ministries, businesses, and researchers. In addition, it plans to provide data storage space for each researcher so that they can conduct data-based research and build a virtual research environment that allows data sharing and collaboration among researchers. In addition, the National Bioresearch Resources Information Center is conducting various activities such as information linkage between ministries, signing memorandum of understandings (MOUs) for research cooperation between institutions, and bioinformatics education and research support.
Milk containing A2 protein is more digestible than milk containing A1 protein [21]. A2 milk was produced on farms where cows with A2 genotypes were identified through the analysis of cow genetic data, and only these were closed and raised. Kim et al. [22] reported the large-scale production of African cattle genomes, shared data with research teams from Ethiopia, Sudan, Kenya, Sweden, and the United Kingdom, and discovered the process of African cattle adaptation to the environment [22].
Several consortia have been established globally to share genetic data within the livestock sector. Notable among these are the Functional Annotation of Animal Genomes (FAANG) and the Agricultural Genome-to-Phenome Initiative (AG2PI) [20]. These initiatives aim to enhance connectivity with the crop research community, promote the sharing of genetic data, conduct diverse research, and concurrently operate specialized educational programs (Fig. 1) [20]. Other consortia of the genetic field in livestock are presented in Table 2.
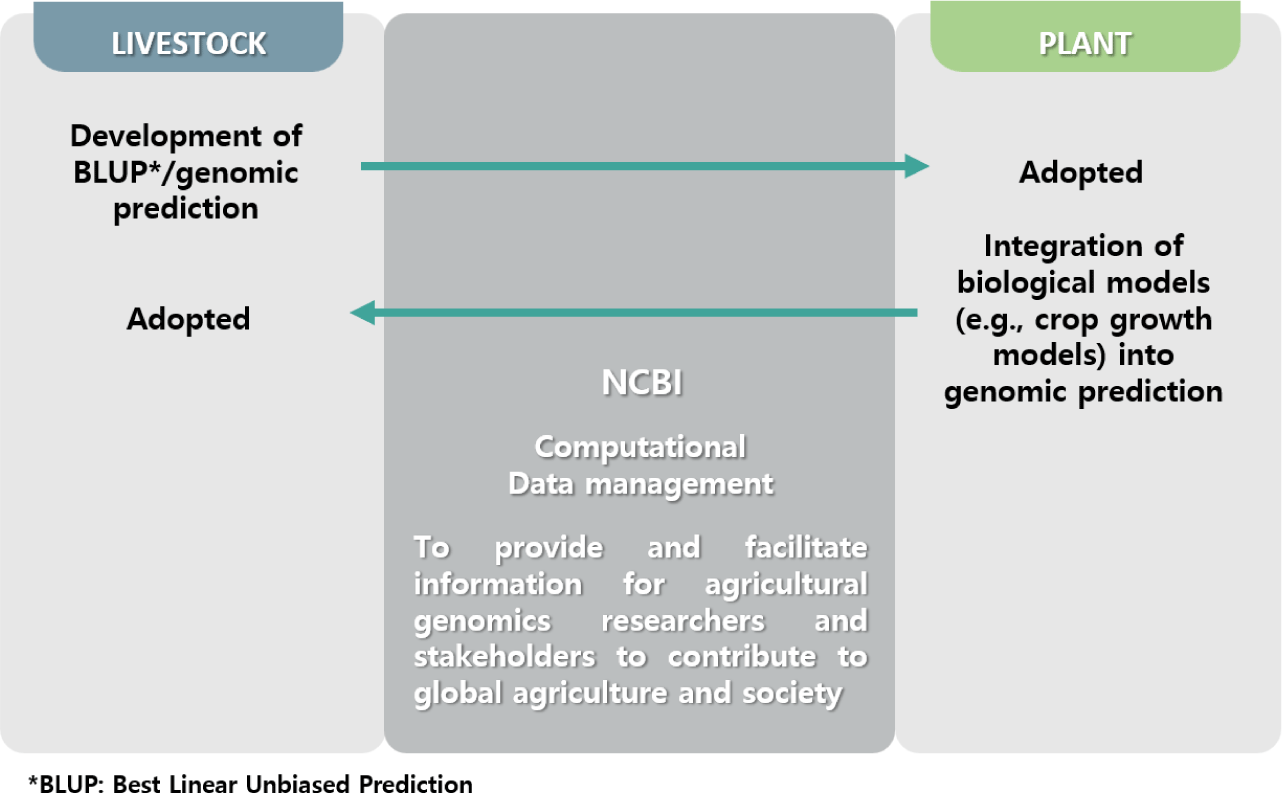
ENVIRONMENTAL DATA IN THE SMART LIVESTOCK INDUSTRY
The establishment of an SLF has enabled the production of comprehensive environmental data, including real-time measurements of the farm environment. These data, which encompass climate data, smart farm data, and more, can be linked to SLFs from the farm to the regional scale. Furthermore, SLFs integrate disease data affecting the livestock industry to establish appropriate quarantine measures or strategies for feed supply, breeding support, and animal welfare.
As previously explained for genetic data, AG2PI cooperates in livestock and crop research fields for common data feedback because there are methodological similarities in research. From the perspective of livestock farming, crops are applied to livestock feed and affect the phenotype of livestock; therefore, crop research fields interact with each other within the ecosystem [20]. The research methods and goals are consistent, such as increasing productivity and developing new varieties to respond to climate change by revealing the relationships between phenotypic, genetic, and environmental data. In addition, because it utilizes a public resource database rather than forming individual communities, it aims to create an integrated community and educate researchers and stakeholders in the agricultural and livestock fields about the flow of information, thereby increasing the utility value of the data. With the list of smart livestock technologies currently in use, we expect large-scale data production and utilization to be possible (Table 1).
An air-recirculated ventilation system based on ICT technology is another environmental system in the SLF. Existing livestock facilities have problems such as a high energy load, poor breeding environment owing to dense breeding, inflow and outflow of diseases into the air, and odors. These problems increase the likelihood of diseases on farms, leading to lower feed efficiency and daily increases in heat and wheat stress, as well as an increase in civil complaints and legal disputes. An ICT-based air circulation ventilation system minimizes the outflow or inflow of pathogens in the air, reduces odors, analyzes the complex environment inside the shed, and optimizes air circulation. The air circulation ventilation system measures air quality, temperature, and humidity inside the shed, stores the data in a farm information system, and operates a control system using ICT devices [23].
Public data, such as climate, disease, market, and economic data, can be used as environmental data for the SLF system. In the past, only the individuals and surrounding environment within the farm were calculated as factors affecting the phenotype; however, with the development of science and technology, the scope of the environment that can be measured is gradually expanding owing to the invention of various means. Because public data include various elements that affect these environmental factors, it can be seen as a factor that can affect the phenotype of the SLF.
The Animal and Plant Quarantine Agency (QIA) provides information on domestic and international animal disease outbreaks. Information conjugated with SLF big data can help farms take proactive measures using the data to prevent or minimize damage from diseases. Weather or feed market information can help make economic forecasts for farm operations. Advanced SLF systems using big data, AI, and machine learning can utilize market information to predict the optimal dispatching time and scale, making farm production plans and profitability predictions more accurate. Thus, if public data can be applied as an environmental factor in the livestock industry, it is expected that the existing labor-intensive livestock industry will develop into a more advanced technology-intensive industry. However, there are still many hurdles to overcome before the commercialization of systems and technologies that can automatically link public data to smart livestock farming. Because no data center can automatically collect and link public data and no software can analyze them for SLF, these two are essential prerequisites for applying environmental factors in the SLF.
A VISION OF THE SLF SYSTEM VIA DATA RECIRCULATION
In an SLF system, it is essential to record the data monitored on farms and animals. The recorded real-time field data in the animal uses sensors (i.e., location, accelerometer data, and temperature data). These real-time data are shaped into big data formats for each SLF. In Europe, SLF systems were installed in five broiler houses and 10 pig houses as part of the EU-PLF project [24]. For 3 years, 90 fattening periods for pigs were monitored, which resulted in a total of 5,475 measuring days. This extensive monitoring generated over 120 terabytes of image data and 4,906,000 sound files, each lasting 5 min [24].
Phenotypic, genetic, and environmental data from SLF have been accumulated as independent big data. However, a comprehensive utilization system that integrates them to produce information with new value is still lacking. The potential of SLF big data is immense and can be doubled by combining and utilizing data from livestock farms and the government, public institutions, researchers, companies, and consumers. This realization should motivate stakeholders to actively participate in the integration and utilization of big data by the SLF. Therefore, recirculating big data in SLF means that farms, livestock product quality evaluation institutes, genetic information data centers, and government public data (e.g., weather and disease information) can be gathered for reprocessing and utilization according to the user’s purpose (Fig. 2).
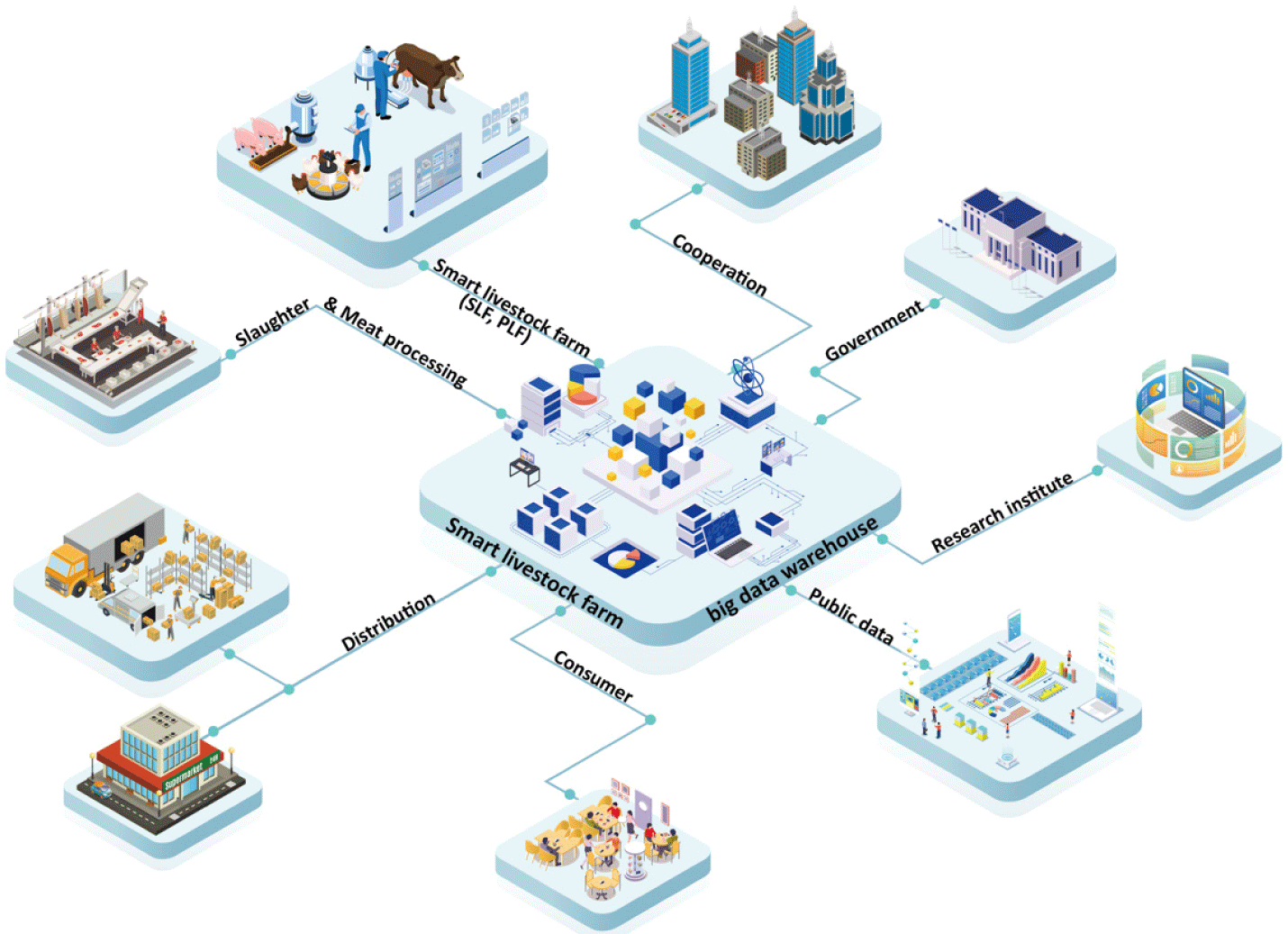
The concept of recirculation of SLF big data has been suggested in the ‘Future Livestock Forum’ in Korea in 2021. The forum also suggests a data warehouse for recirculating SLF big data. A data warehouse is a more advanced concept than a traditional database and can be seen as more structured and systematic than a user-based, free-form data lake. This type of data warehouse differs from the databases listed in Table 3. Moreover, because SLF big data cannot exclude information that falls under the category of private property, the need for an integrated operating entity has been raised from the perspective that it is necessary to establish a governance system that can be operated by a public institution or consultative body for big data collection and management. Owing to the governance needs, the work related to the SLF big data integrated operation, previously managed by the ‘Korea Agency of Education, Promotion & Information Service in Food, Agriculture, Forestry & Fisheries (EPIS)’, has been transferred to the KAPE. This ensures that information on smart livestock from farms to animal product quality is gathered in one place. Farm environmental information and information on the objects collected from each farm for smart agriculture, including SLF data, are converted into a database and built into the ‘Smart Farm Data Mart’ DB of Smart Farm Korea. However, although individual information, farm information, etc., are collected in one place, the genetic information to be used for genetic improvement is collected separately. Therefore, it is necessary to realize complete SLF big data via a data center, forming a data warehouse. Looking at the list of smart livestock technologies (Table 1), we can see that much effort is being devoted to collecting and utilizing the collected data. Therefore, as smart livestock technologies are developed and distributed in the field, the effects of data feedback are expected to increase.
A digital twin is a representative field application of data recirculation, a process in which data are continuously collected, analyzed, and fed back into a system [4,25]. It is a digital replica of a real object that is kept up-to-date with continuous data inflow. Applying digital twins in the livestock industry is expected to be very useful for understanding and improving current complex systems and building new ones. However, digital twinning occurs at the toddler stage because of some challenges such as inadequate communication, data recirculation, and conflicts of interest among stakeholders (Fig. 3) [4,25,26]. In Europe, some countries have attempted to construct digital twins based on SLFs. Wageningen University in the Netherlands is at the forefront of research on digital twins in the agricultural sector, particularly in livestock farming [16,25]. The university is defining digital twins as applicable to livestock farming and continuously enhancing the connection between physical and virtual environments, keeping the industry informed [25]. In England, the University of Leeds constructed the National Pig Centre for digital twinning [4]. The center was developed to cooperate with engineering and computer sciences. The center set a goal for achieving net zero production by 2030 via multi-platform SLF technologies, including digital twinning [4].
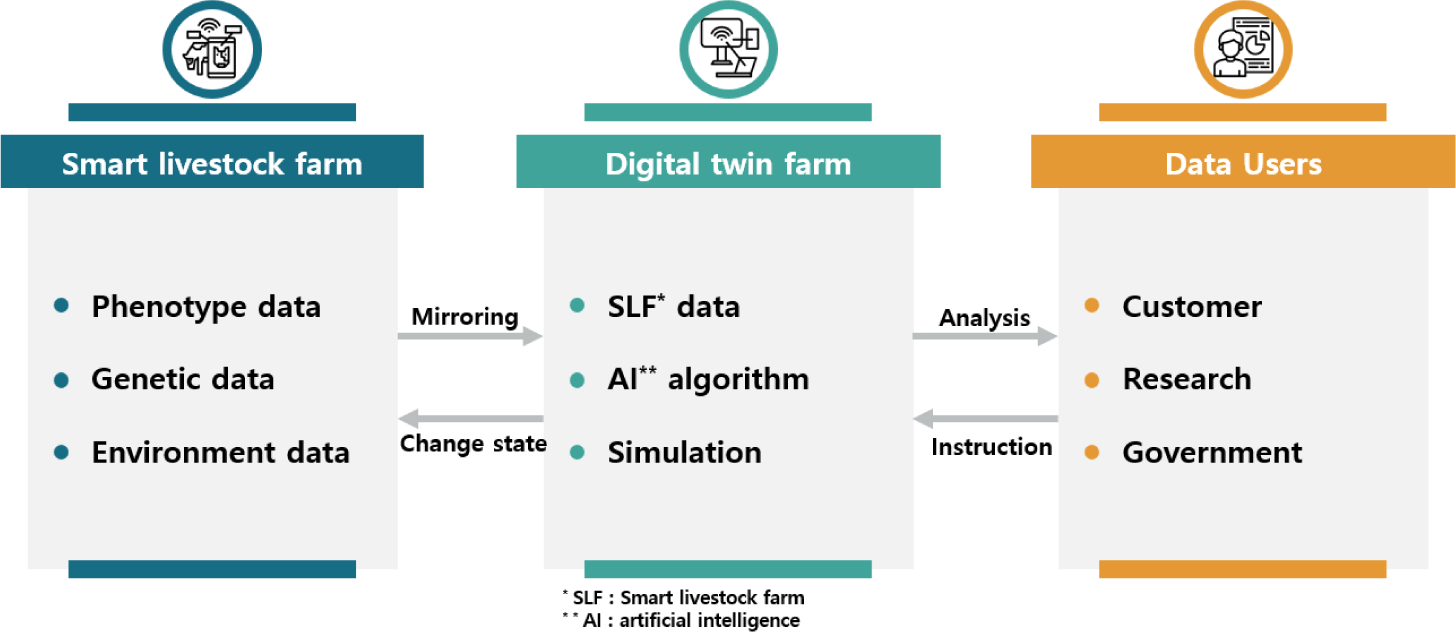
Several conditions must be met before South Korea adopts digital twinning:
-
SLF, in its total sense, must be implemented for each livestock species. A policy encouraging extensive equipment investment is required to achieve a complete understanding of SLF.
-
It is necessary to recognize the publicity of the data produced by the SLF and cooperate to improve mutual understanding when sharing data. The data produced by each farm and institution often contain sensitive information. However, efforts are required to reassure people about the security of this information through robust legal mechanisms.
-
An SLF big data recirculation center is required. The SLF data produced by many farms are big data, and the information produced by each farm is often too large for the farm to process on its own. Therefore, it is necessary to build and operate a data center that can recirculate and store data for public purposes.
In addition, by combining public data and farm information operated by the state with AI and machine learning technologies and integrating SLF big data, the digital twin is expected to further increase the economic added value of the livestock industry by operating farms or implementing policies through simulations in advance.