INTRODUCTION
The fatty acid composition of meat has recently received more attention among meat producers, researchers, and consumers due to its effects on consumer health and meat quality, particularly meat flavor [1]. The fat content in muscle, intramuscular fat (IMF), and the fatty acid composition greatly influence the flavor, juiciness, and tenderness of meat [2]. Fatty acids are components of fat, and are subdivided into: saturated fatty acids (SFAs) and unsaturated fatty acids (UFAs) [3]. The latter are subdivided into monounsaturated fatty acids (MUFAs) and polyunsaturated fatty acids (PUFAs) [3]. Except for stearic acid, the consumption of SFAs has been reported to increase the content of blood cholesterol which is linked with heart disease [3]. By contrast, UFAs confer health benefits to consumers [4].
In chicken meat, palmitic acid is the predominant SFA, while oleic and linoleic acids are the most abundant MUFA and PUFA, respectively [5]. The composition of acid composition in meat affects its flavor by releasing the final flavor compounds through thermal oxidation during cooking [6]. The flavor compounds of fatty acids include alkanes, aldehydes, ketones, and organic acids [1]. For example, arachidonic acid (C20:4) is associated with better sensory characteristics of chicken meat [6, 7], oleic acid (C18:1) is a good meat flavor precursor in chicken [6], and docosahexaenoic acid (DHA; C22:6) suppresses sourness to improve the sweetness and umami taste of meat [6]. Linoleic acid (C18:2) also improves meat flavor [1].
The fatty acid composition in meat is a polygenic trait controlled by genetic and environmental factors [7]. Some fatty acids have very low heritability, and it is very difficult to improve low-heritability traits using conventional methods [8]. Genomic selection is effective for improving the performance of low- to moderate-heritability traits [9]. Different genes have been reported to influence fatty acid synthesis in chicken meat, including DEGS1, ELOVL6, FABP3, FABP4, FASN, and SCD [10]. Heritability estimates range from low for eicosenoic acid (0.025) to moderate for palmitic acid (0.290) and high for arachidonic (0.552), oleic (0.560), and docosahexaenoic (0.510) acids in Korean native chicken (KNC) breast meat [11], suggesting that it is possible to breed chickens for favorable fatty acid composition using marker-assisted selection (MAS). Genetic methods such as genome-wide association studies (GWAS) are very effective for finding genomic regions and potential candidate genes for traits of interest [12]. For example, GWAS were used to identify the candidate genes for growth traits, disease resistance, and other important traits in chickens [13]. However, the GWAS of the fatty acid composition in chicken meat are very scarce. Therefore, this study sought potential candidate genes for the composition of fatty acid in KNC-red-brown line (KNC-R) chickens using GWAS.
MATERIALS AND METHODS
This study referred to the guidelines established by the Institution of Animal Care and Use Committee of the National Institute of Animal Science (NIAS 20212219).
We used 382 KNC-R chickens (190 males, 192 females) from one population kept at the Poultry Research Institute’s farm of NIAS in Pyeongchang, South Korea. We chose chickens from 2 generations as shown in Table 1, each generation was 52 weeks. Housing, hatching, management, feeding, slaughtering, and carcass storage conditions are described in our previous study [13]. We collected blood samples from 382 KNC-R chickens and stored at −20°C until DNA extraction. At the age of 10 weeks, all 382 KNC-R chickens were slaughtered, carcasses were eviscerated, and then breast meat was separated and kept under −80°C until usage.
Generation | Male | Female | Total |
---|---|---|---|
Generation 1 | 98 | 92 | 190 |
Generation 2 | 92 | 100 | 192 |
Total | 190 | 192 | 382 |
The fatty acid composition was analyzed from breast meat samples collected from 382 KNC-R chickens slaughtered at 10 weeks old. The fatty acid methyl ester (FAME) method was used to extract the fatty acids following all procedures used by [14]. The content of each fatty acid was expressed in percentage. We measured 24 fatty acid traits, including SFA, SFAs: myristic acid (C14:0), palmitic acid (C16:0), stearic acid (C18:0); MUFA, MUFAs: palmitoleic acid (C16:1), oleic acid (C18:1), nervonic acid ([NA] C24:1); PUFA, PUFAs: linoleic acid (C18:2), linolenic acid (C18:3), eicosadienoic acid ([EDA] C20:2), mead acid (C20:3), arachidonic acid (C20:4), eicosapentaenoic acid (C20:5), DHA (C22:6), UFA, ratio between UFAs and SFAs (UFA/SFA), ratio between PUFAs and SFAs (P/S), ratio between omega-6 and omega-3:n-6/n-3, omega-6: n-6, omega-3: n-3, atherogenicity index (AI), thrombogenicity index (TI). We used the Shapiro-Wilk test to normalize phenotypes and different methods including, log, cube, tri, multi, or square root scaling were used for normalizing our phenotypic data as presented in Table 2.
KNC-R, Korean native chicken-red-brown line; CV, coefficient of variation; SFA, ssaturated fatty acid; log, logarithm; tri, triple; MUFA, monounsaturated fatty acid; sqrt, square root; PUFA, polyunsaturated fatty acid; multi, multiple; UFA, unsaturated fatty acid; P/S, ratio between PUFAs and SFAs; AI, atherogenicity index; TI, thrombogenicity index.
Genomic DNA from blood samples of 382 KNC-R chickens was extracted by using a commercial toolkit of GeNetBio (GeNetBio, Daejeon, Korea). The Illumina chicken 60 K single nucleotide polymorphism (SNP) chip (Illumina, San Diego, CA, USA) was used for genotyping 382 DNA samples. We used the PLINK1.9 version 1.90b5.2 software [15] to control of the quality of the genotypic data. We based on four criteria: low genotyping call rate (< 0.9), minor allele frequency (< 0.01), missing genotype call (> 0.1), and low Hardy–Weinberg (< 10−6), to exclude SNPs from the analysis. A total of 44,573 SNPs remained and were used for GWAS.
GWAS between SNPs from 382 genotyped samples and twenty-four fatty acid traits was conducted by the mixed linear model (MLM) leaving-one-chromosome-out (MLMA-LOCO) of genome-wide complex trait analysis (GCTA) software, version 1.93 [16]. The covariates were: sex, generation, body weight, and the top two principal components. The mathematical model used was as follows.
where, y is the phenotypic value of each fatty acid corrected with covariates (sex, generation, body weight, and PC1 and PC2 of a principal component analysis), a is the mean of the phenotypic value; b is the additive effect of the tested SNP marker; x is the genotype of the SNP; g − is the effect of all SNPs excluding SNPs on the chromosome where the candidate SNP is mapped; and e is the residual effect vector. Bonferroni-corrected p-value (α = 0.05) was used to identify the significant SNPs among the tested SNPs. We used the restricted maximum likelihood (REML) of the GCTA software to estimate the heritability of each fatty acid.
We screened the significant SNPs by setting the Bonferroni-corrected p-value (α = 0.05), and the candidate genes were identified within the 1 Mb (0.5 Mb upstream and downstream) region surrounding the significant SNP, and then annotated based on the GRCg6a 106 version from the Ensembl genome database. Then, candidate genes were used to perform (Kyoto Encyclopedia of Genes and Genomes [KEGG]) pathways and gene ontology (GO) analyses by using the g: profiler database [17] by considering the significance level of 5%. Moreover, we searched different databases such as PubMed and NCBI to find the biological functions of the candidate genes.
RESULTS
Table 2 provides descriptive statistics for the different fatty acid profiles in 382 KNC-R chickens. The predominant fatty acids in KNC-R chickens were oleic (C18:1; average 28.252%), palmitic (C16:0; 20.895%), linoleic (C18:2; 15.975%), and arachidonic (C20:4; 10.541%) acids. Table 3 gives the heritability estimates for the fatty acid profiles of 382 KNC-R chickens. The heritability range was 0–0.438 (Table 3).
We identified significant SNPs based on the Bonferroni-corrected p-value (p < 0.05). Significant SNPs were identified for NA (C24:1), linoleic (C18:2), and EDA (C20:2) acids. The top significant SNP for C24:1 was rs13667281 (p = 5.25 × 10−07) on chromosome 2 at bp 31215920, the most significant SNP for C18:2 was rs14011157 (p = 7.69 × 10−07) on chromosome 10 at bp 16289438, and the most significant SNP for C20:2 was rs10731996 (p = 7.89 × 10−07) on chromosome 22 at position 2910806 bp (Table 4). Here, we defined the significant genomic region for all traits as the region within 0.5 Mb upstream and downstream of the most significant SNP. The top significant SNP for nervonic acid (C24:1) was rs13667281 which is intron variant located within IGF2BP3 gene. The top significant SNP found for linoleic acid (C18:2) was rs14011157 is intergenic variant while the most significant SNP for eicosadienoic acid (C20:2) was rs10731996 which is a missense variant located with in PPP1R3E gene (Table 4).
For nervonic (C24:1), linoleic (C18:2), and eicosadienoic (C20:2) acids, the most significant genomic regions were 30,725,920–31,715,920 bp on chromosome 2, 15,789,438–16,789,438 bp on chromosome 10, and 2,410,806–3,410,806 bp on chromosome 22, respectively. Fig. 1 and Table 4 present the GWAS results for each SNP. We identified 5, 1, and 7 functional candidate genes for C24:1, C18:2, and C20:2, respectively (Table 5). There were no significant GO terms nor KEGG pathways for genes in the significant genomic regions. However, we searched the literature to identify functional candidate genes for C24:1, C18:2, and C20:2.
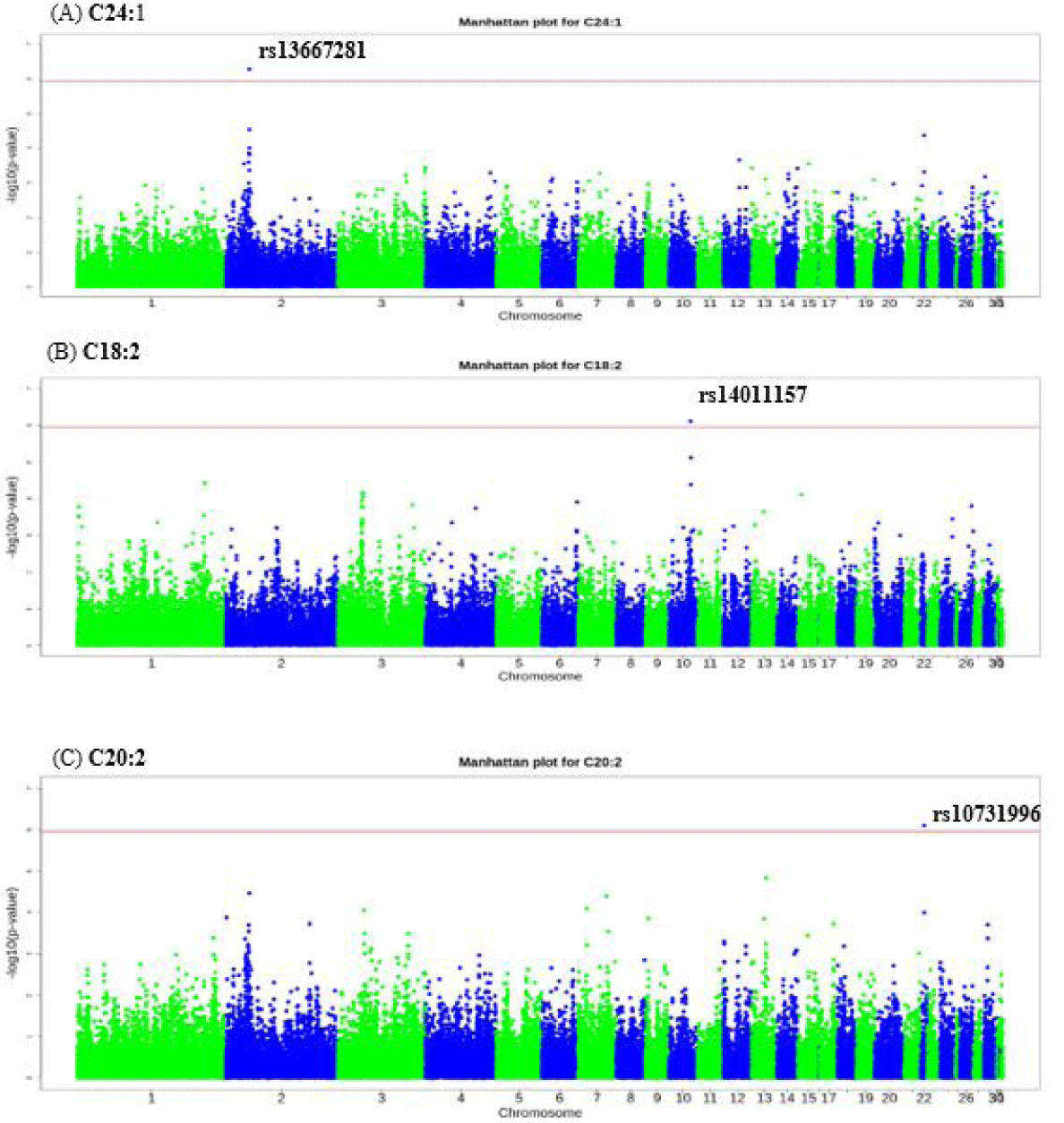
DISCUSSION
Animal fatty acid composition is controlled by genetic and environmental factors [18]. The fatty acid composition of meat markedly influences meat quality and consumer health [3,6]. We found that the predominant fatty acids in KNC-R chickens were oleic (C18:1; 28.252%), palmitic (C16:0; 20.895%), linoleic (C18:2: 15.975%), and arachidonic (C20:4; 10.541%) acids (Table 2), in agreement with previous findings [6,7]. This study also calculated the AI and TI. The AI is the ratio of all pro-atherogenic SFAs (C12:0, C14:0, and C16:0) and anti-atherogenic UFAs (MUFAs and PUFAs) [14,19] while the TI is the ratio of pro-thrombogenic SFA (C14:0, C16:0, and C18:0) and anti-thrombogenic fatty acids (MUFAs and PUFAs: omega-6 and omega-3) [14,19]. AI and TI are good indicators of the quality of the fat in meat and milk, and lower values of AI and TI indicate that the fat contains more MUFAs and PUFAs [19,20].
Estimating heritability is very important for designing breeding programs and predicting the selection response [21]. We estimated heritability ranging from 0 for C14:0, C18:3, and n-3 fatty acids to 0.438 for SFAs (Table 3). Generally, the heritability estimates were lower than those reported in [11] but in the same range as those in [7]. Heritability estimates depends on the genetic background of the sampled population [21]. Here, we report the heritability estimates of AI (0.391) and TI (0.395) traits for the first time. The AI and TI traits have moderate heritability, suggesting that breeding chickens with favorable AI and TI is possible. Genetic selection is the best tool for improving meat quality traits with low or high heritability [22].
The GWAS identified genes related to lipid metabolism, which influences the synthesis of different fatty acids. Genetically, the composition of fatty acid is controlled by many genes with small effects [23]. Different candidate genes that influence the fatty acid composition of chicken meat have been reported on chromosomes 1 (ADIPOR2, LRP6, and FAR2), 2 (GCNT2, FABP4, and LRP12), 3 (FABP7 and DEGS1), 4 (ELOVL6), 6 (SCD), 7 (MGAT5 and LRP1B), 9 (HDLBP and ADIPOQ), 10, and 18 (FASN) [10, 11]. Recent findings have mapped candidate genes for different fatty acid profiles on chromosomes 1, 3, 4, and 10 [7]. Here, we reported candidate genes influencing C24:1, C18:2, and C20:2 fatty acids on chromosomes 2, 10, and 22, respectively.
The NA (C24:1) plays a role in brain development, brain maintenance, and memory improvement [24]. In poultry, fat is mainly synthesized in the liver [25], while de novo NA (C24:1) synthesis occurs in the cytoplasm and results from the elongation of oleic acid (C18:1) by ELOVL3 [26]. Exploration of the significant genomic region based on the significant SNP associated with NA revealed 16 protein-coding genes on chicken chromosome 2. However, only a few are functionally linked to lipid metabolism: the IGF2BP3, GPNMB, NPY, OSBPL3, and IL6 genes.
Glycoprotein non-metastatic melanoma protein B (GPNMB) is encoded by the GPNMB gene, which is involved in melanin deposition, bone mineral deposition, regulation of inflammation, and lipid metabolism [27]. The GPNMB gene SNP (rs31126482) was reported to be linked with the weight of abdominal fat [27]. Additionally, GPNMB overexpression significantly increased the expression of the FASN, SCD, ACACA, ACSL1, SREBP1, and PLIN2 genes, involved in the synthesis of different fatty acid [27]. The GPNMG gene might be a useful genetic marker in poultry breeding for fatty acid profiles. Insulin-like growth factor binding protein-3 (IGF2BP3) is a RNA-binding protein that controls IGF2 expression [27]. IGF2BP3 is also associated with IMF deposition in chickens [28].
Other candidate genes identified on chicken chromosome 2 include neuropeptide Y (NPY), which is encoded by the NPY gene. Its variants, such as rs16139, have been associated with obesity, high plasma LDL-cholesterol, and coronary artery disease in humans [29]. Oxysterol-binding protein-like 3 (OSBPL3), a member of the OSBP family, is encoded by the OSBPL3 gene and has a key role in hepatic fat accumulation [30]. Interleukin-6 (IL-6), encoded by the IL6 gene, influences peripheral lipid metabolism [31].
Linoleic acid influences meat flavor [1] and is synthetized via the desaturation of oleic acid (C18:1) by fatty acid desaturase 2 (FADS2) [26]. On chromosome 10, we found one candidate gene, the NR2F2 gene, also known as COUP-TFII. NR2F2 is involved in adipogenesis, lipid metabolism, and insulin secretion [32].
EDA (C20:2) is an omega-6 PUFA that is formed through the elongation of linoleic acid (C18:2) by ELOVL5 [26]. Among the 32 protein-coding genes found in the significant genomic region on chromosome 22, only 7 had functions related to lipid metabolism: the GPAT4, PPP1R3E, NKX6-3, ANK1, SFRP1, ERLIN2, and STAR genes. Glycerol-3-phosphate acyltransferase 4 (GPAT4) is the rate-limiting enzyme in the synthesis of glycerophospholipids (phosphoglycerides) and triacylglycerol (TAG or triglycerides) [33]. GPAT4 influences hepatic lipid accumulation [34].
Protein phosphatase 1 regulatory subunit 3E (PPP1R3E) encodes a regulatory subunit of PP1, which is involved in glycogen metabolism [35]. PPP1R3E expression is regulated by insulin [35]. NK6 homeobox 3 (NKX6-3) is encoded by the NKX6-3 gene and its expression is associated with increased triglyceride levels; thus, it is involved in lipid metabolism [36]. ANK1 belongs to the ankyrin family and is linked to IMF and meat quality traits, such as tenderness in pork [37].
Secreted frizzled-related protein 1 (SFRP1) is an SFRP protein that controls adipogenesis [38]. SFRP1 regulates other genes involved in de novo fatty acid synthesis, including FASN, ACACA, ACLY, ELOVL, and SCD1 [38]. ER lipid raft-associated protein 2 (ERLIN2) is a prohibitin that regulates lipid metabolism [39]. Steroidogenic acute regulatory protein (STAR) is the rate-limiting step in steroidogenesis [40]. To our knowledge, this is the first report of genes on chicken chromosome 22 that are involved in lipid metabolism.
The human body needs fatty acids for important functions, including brain development. However, it is unable to synthesize fatty acids such as omega-3 (n-3) and omega-6, which must be obtained from the diet [41]. Chicken, a globally popular and inexpensive meat [42], and is a good source of these fatty acids. Excessive consumption of some fatty acids is dangerous to humans, while other fatty acids are very beneficial. Fatty acids influence the flavor of meat and can also affect consumer health. Thus, selecting and producing meat with desirable fatty acids not only improves meat flavor but can also improve human health. This study reported different candidate genes affecting the fatty acid composition of chicken meat. The limitation of the current study was the small sample size because we only used 382 samples. Therefore, these results need to be validated in larger sample size. Furthermore, mapping studies are also needed to validate the effects of different variants in the candidate genes.
CONCLUSION
Fatty acid composition is a polygenic trait influenced by many genes with small effects, as well as by environmental factors including diet. These traits have low-to-moderate heritability, and it is possible to improve them using genomic selection. Through GWAS, we identified potential candidate genes affecting the fatty acid profile, such as IGF2BP3, GPNMB, NPY, OSBPL3, IL6, NR2F2, GPAT4, PPP1R3E, NKX6-3, ANK1, SFRP1, ERLIN2, and STAR. Interestingly, we found the GPNMB and SFRP1 genes, whose expression regulates other genes related to fatty acid synthesis, including the FASN, ACACA, ACLY, ELOVL, and SCD genes. Our findings provide insight into the genes influencing lipid metabolism and fatty acid synthesis. Moreover, the identified SNPs might be used as biomarkers in chicken breeding.