INTRODUCTION
Low-birthweight piglets are associated with a reduced survivability and performance [1], thus contributing to the high production costs encountered by many commercial swine enterprises. Most of the management interventions to alleviate these impacts, have been based on optimizing intake of nutritious feed and use of growth promoters, including antibiotics [2]. However, these methods have yielded mixed results. Further, the use of antibiotics could have short- and long-term effects on the health of the animals as well as contribute to the propagation of antimicrobial resistance [3,4].
Meanwhile, recent studies have shown that the microbial composition along the digestive tract has a considerable bearing on the development of the host’s immune system [5] and efficiency of nutrient utilization [6] thus potentially promoting survival and weight gain. Other areas where the microbiota has been found to play important roles include drug metabolism [7] and feeding behavior [8] further affecting health and weight gain, respectively. However, the microbiota is simple and unstable in piglets and has to mature into a relatively more complex and stable community in order to optimally confer these benefits to the animal. Around the time of birth, the neonate receives microbial inocula from its interaction with the sow and the immediate environment [9]. This interaction continues throughout nursing, postweaning and the rest of the pig’s life thus shaping the composition of the microbiota. During development, the microbiota can be influenced by several factors including, diet [10], environment [11], weaning [12], antibiotics [13] and probiotics [14].
In order to gain insights into the role played by the microbiota, an understanding of the phylogenetic composition and functional capacity of the microbial community is crucial. With the advent of Next Generation Sequencing techniques, we are able to target and sequence a marker gene such as the 16S ribosomal RNA (16S rRNA) gene in order to describe the phylogenetic composition and diversity of the bacteria and archaea within an environment [15]. And by employing a computational model, such as Phylogenetic Investigation of Communities by Reconstruction of Unobserved States (PICRUSt), we are able to reconstruct microbial metagenomes and ultimately predict metabolic pathways within the communities using only this 16S rRNA gene sequence data and a reference genomes database [16]. While several studies have been done on the microbial communities in the gastrointestinal tract [17,18], the swine oropharyngeal microbiota remains understudied. Interestingly, the oropharynx is in communication with both the digestive and respiratory tracts which are the major routes of infection by pathogenic organisms in the neonatal and weaning stages of life and thus could greatly influence health and performance.
In the current study, we therefore aimed to profile the bacterial composition and predicted metabolic functions of the oropharyngeal microbial community in low and high birthweight piglets and in piglets with low and high average daily gain (ADG) using next generation sequencing. We hypothesized that the rate of weight-gain (ADG) of the heavier piglets would be associated with a characteristic oropharyngeal microbial signature.
MATERIALS AND METHODS
The study was conducted on a commercial swine farm with 1,100 breeding sows (Ogeum farm, Yeoju, Korea). A total of 8 mixed sex piglets (3-way crosses; Landrace × Yorkshire × Duroc) born on the same day to two 3rd parity sows were used in the study. Four (4) piglets with a birthweight ≤ 1.0 kg and 4 with a birthweight ≥ 1.7 kg (Table S1), were selected and identified using an oil-based marker that was replenished every week. The body weight of each piglet was measured every week (Table S1), and the average daily gain was calculated by week (Table S2).
The piglets had ad libitum access to water and feed throughout the study. At 10 days of age, they were introduced to a creep feed until weaning after which they were graduated to a post weaning diet (see Table S3 and S4 in the supplementary materials). The commercial feed was based on corn and soybean, however, modifications to the texture and relative composition of additives was made as the piglets grew. Minerals, vitamins, enzymes, probiotics and occasionally antibiotics were added to the feed, based on the particular requirements of the co-housed piglets (see Tables S4 and S5). Prophylactic therapies instituted during this period included; Kanamycin administered intranasally at day 1 to control incidences of atrophic rhinitis; vaccinations against Mycoplasma hyopneumoniae, Porcine circovirus, Erysipellothrix rhusiopathiae, classical swine fever virus as well as against Foot and Mouth disease virus. In addition, colistin was added into the feed.
The oropharyngeal sample was collected by swabbing the soft palate, the root of the tongue and the left and right lateral walls of the oropharyngeal cavity, twice at each surface, using a sterile absorbent swab. The samples were collected at 11, 26, and 63 days of age and stored at −20°C for about 2 weeks before being transferred to the lab where they were kept at –80°C.
The piglets were individually weighed weekly and records of clinical condition monitored daily. Coughing, sneezing, diarrhoea, general weakness, fur coat texture, injuries among others were monitored.
DNA from the oropharyngeal swab samples was extracted using the Epicenter MasterPure™ DNA Purification Kit (Epicenter, Madison, WI, USA) following the manufacturer’s protocol. First, each sample was rehydrated in 150 μL of autoclaved deionized water in a micro-centrifuge tube at room temperature (25°C) for 30 minutes, vortexed for 15 seconds and then the swab was removed and squeezed against the inside wall of the micro-centrifuge tube. With the resultant solution, the protocol was then followed verbatim. The purity of extracted DNA was assessed by spectrophotometry (NanoDrop Spectrophotometer, Thermo Fisher Scientific, Waltham, MA, USA) through determination of the 260:280 and 260:230 absorbance ratios, while DNA concentration was determined by fluorometry using Qubit HS dsDNA assay kit (Life Technologies, Thermo Fisher Scientific, Waltham, MA, USA). DNA concentration was then normalised to 5 ng/μL and stored at −20°C.
The V4 hypervariable regions of the bacterial 16S rRNA gene was amplified using the forward primer, 515F (Parada) (5’-GTGYCAGCMGCCGCGGTAA) [19] and the reverse primer, 806R (Apprill) (5’-GGACTACNVGGGTWTCTAAT) [20]. These primers were designed with illumina overhang adapters that are complementary to illumina sequencing primers. The forward overhang nucleotide sequence (5’-TCGTCGGCAGCGTCAGATGTGTATAAGAGACAG-3’) was added to the 515F primer while the reverse overhang nucleotide sequence (5’-GTCTCGTGGGCTCGGAGATGTGTATAAGAGACAG-3’) was added to the 806R reverse primer. A 25 μL PCR reaction mixture was set up, consisting of 12.5 ng of the DNA template, 1.0 Units of Taq DNA polymerase, 1 μM (5 μL) of each forward and reverse primers, 200 μM of each deoxynucleotide triphosphate (dNTP) and 1x buffer. Along with the PCR reaction, water as a negative control, a known positive control and extraction control reactions were included. The thermocycler was set at an initial denaturation temperature of 95°C for 3 minutes followed by 25 cycles of 95°C for 30 seconds, annealing at 56°C for 30 seconds and elongation at 72°C for 30 seconds followed by a final elongation for 5 minutes.
The amplicon libraries were cleaned up using Agencourt AMPure XP beads (Beckman Coulter, Brea, CA, USA) according to the manufacturer’s protocol and then quantified by fluorometry using Qubit High Sensitivity dsDNA assay kit (Life Technologies, Thermo Fisher Scientific, Waltham, MA, USA).
The PCR amplicons were prepared for sequencing by adding indices and illumina sequencing adapters using the Nextera XT DNA index kit (Illumina, San Diego, CA, USA) according to the manufacturer’s instructions for single end sequencing. The indexed libraries were then purified using Agencourt AMPure XP beads, quality checked on a Bioanalyzer DNA 1000 chip and then quantified by fluorometry using Qubit HS dsDNA assay kit. The libraries were then diluted to an equimolar concentration of 4 nM before pooling for sequencing. A final confirmation of the pooled library concentration was done by a fluorometric measurement before denaturing and sequencing. The pooled genomic libraries were then sequenced using the Illumina iSeq 100 platform and using the single-end sequencing method.
Analysis was done using QIIME 2 2018.11 [21]. Raw FASTA files were quality filtered and denoised using DADA2 [22] through the q2-dada2 plugin. This involved clipping off of illumina associated adaptor and barcode sequences, followed by trimming of low-quality ends [at 200 base pairs (bp)] as well as removal of low-quality bases. All the sequences were then aligned to the MAFFT [23] and the aligned sequences used to construct a rooted phylogenetic tree using fasttree2 [24] via q2-phylogeny. We then rarefied the samples to 28,076 sequences per sample and estimated alpha diversity metrics, beta diversity metrics and Principle Coordinate Analysis (PCoA) using q2-diversity. The alpha diversity metrics included; Observed operational taxonomic units (OTUs), Evenness, Shannon diversity [25] and Faith’s Phylogenetic Diversity [26] while the beta diversity distance matrices included unweighted UniFrac [27], weighted UniFrac [28] and Bray-Curtis dissimilarity [29]. The estimated beta diversity between communities was then visualised using principal coordinate analysis (PCoA) plots. To assign taxonomy to the amplicon sequence variants (ASVs), we used the q2-feature-classifier [30] basing on the classify-sklearn naïve Bayes taxonomy classifier with the Greengenes 13_8 99% OTU’s as reference sequences [31]. We assessed the progress of development within the microbiota composition from 11 days through weaning to 63 days of age using q2-longitudinal [32]. Using PICRUSt (phylogenetic Investigation of Communities by Reconstruction of Unobserved States) [16] we predicted KEGG orthology (KO) metagenomes, enzyme commission (EC) metagenomes and MetaCyc pathway abundances through the q2 picrust2 plugin.
The data from QIIME 2 was imported into R statistical software [33] for statistical analysis using vegan [34] and Bioconductor packages. To test for statistically significant associations between ADG and relative abundance of genera or pathway abundances, we fit a generalized linear regression using the edgeR package [35]. We corrected for multiple hypothesis testing using the false discovery rafe (FDR) correction method.
RESULTS
The twenty-four samples sequenced yielded a total of 3,212,205 sequences ranging from 4 to 284,317 sequences per sample (mean = 133,841.875 and median = 164,482). Quality control and subsequent removal of poor-quality reads in QIIME2 reduced the sequences to a total of 2,390,641 sequences with a mean of 99,610 sequences per sample (ranging from 3 – 243,908 sequences while median = 117,350.5).
In order to create an even subsample from each of the samples for calculation of diversity, a sub sampling depth of 28,076 was used. This eliminated 2 samples (B1P2 and B1P5) with fewer reads and left 22 samples with a total of 617,672 reads that were then used to calculate alpha and beta diversities among the samples. The rarefaction plot based on the observed OTU’s (Fig. S1) showed a trajectory that initially steeply increased before levelling off at around 40,000 sequences. This indicated that there was good coverage of the communities among all samples.
The sequences were then clustered into 1,848 OTUs. Taxonomy was assigned by aligning the sequences against the Greengenes 16S rRNA data base at 99% similarity using a Naïve Bayes classifier trained on the V4 hypervariable region. The sequences were dominated by members of the kingdom Bacteria except for two genera belonging to the kingdom Archaea. Proteobacteria and Firmicutes were the most dominant phyla, with a combined relative abundance ranging from 53.17%–97.4% (Fig. 1A). Streptococcaceae, Pasteurellaceae, Moraxellaceae, Veillonellaceae, Neisseriaceae, Leptotrichiaceae, and Lachnospiraceae were the most represented families (Fig. 1B). Lactobacillaceae tended to increase in relative abundance post weaning. The most prevalent genera within the samples were Streptococcus (Firmicutes) Actinobacillus (Proteobacteria), Moraxella (Proteobacteria), Veillonella (Firmicutes) and Haemophilus (Proteobacteria) (Fig. 1C).
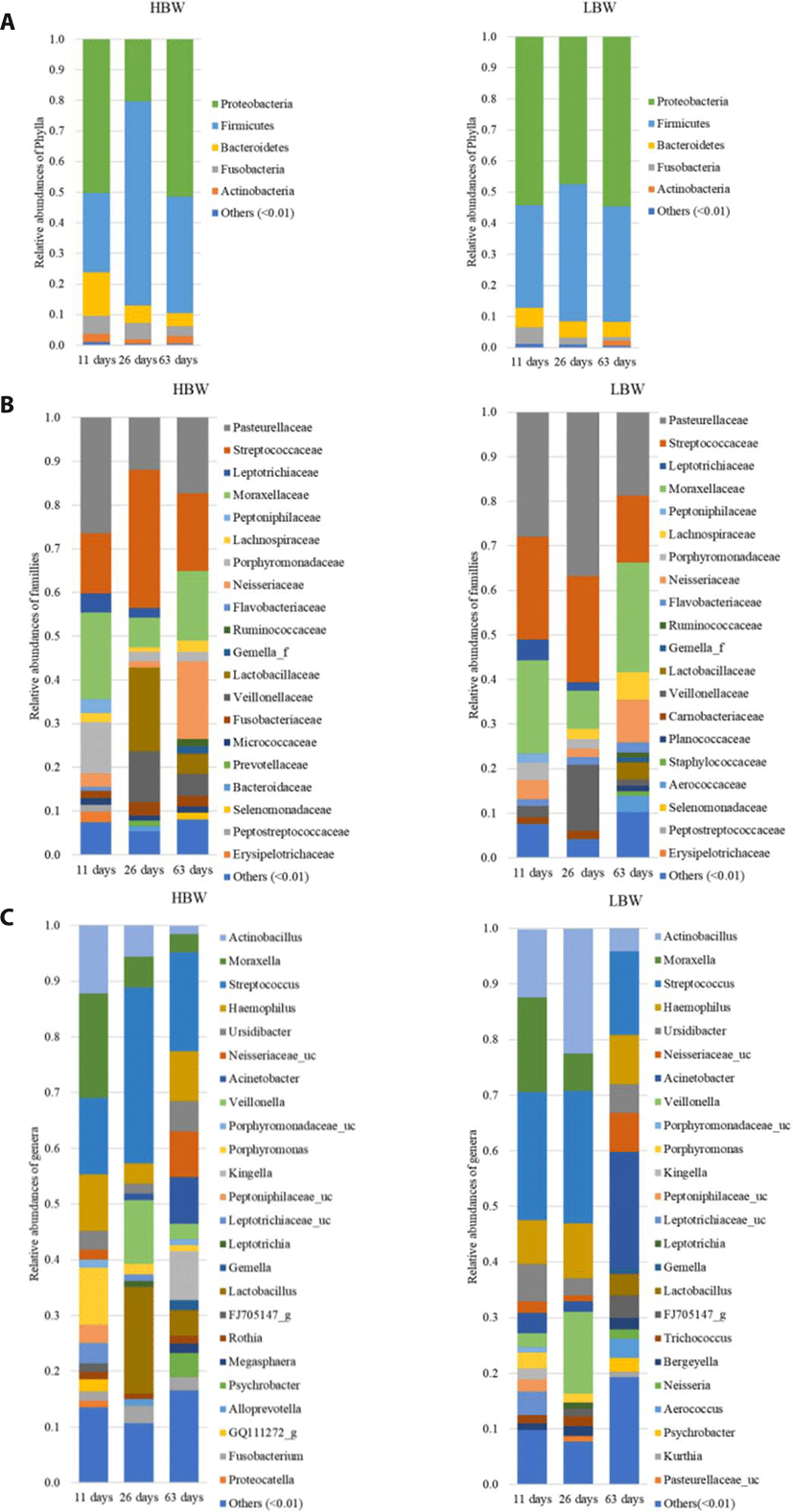
In order to study the broad changes in microbiota during development, samples were collected at 11, 26, and 63 days of age. Within the first 11 days of life, the piglets’ diet was composed entirely of sow milk and creep feeding was initiated at day 12 of life. The samples collected on the 26th day of life represent the oropharyngeal microbial community at the end of nursing. While the samples collected at 63 days of life represented the oropharyngeal microbiota before the end of the weaner stage of life.
PCoA showed that the samples already clustered closely by day 11 of age regardless of maternal or foster sow, that nursed them. Pena Cortes et al. [36] reported a litter effect in the tonsillar microbiota that disappeared by the third week. We did not observe this litter effect by the 11th day of life, probably due to the advancement in age of the piglets in our study compared to those in their study [36]. Further, the samples tended to clusters by age of the piglets except for 2 piglets at 26 days of age, that had been weaned 6 days earlier (Fig. 2). The divergence of the weaned piglets microbiota was expected since weaning stress has been found to disrupt the tonsillar microbial composition [37].
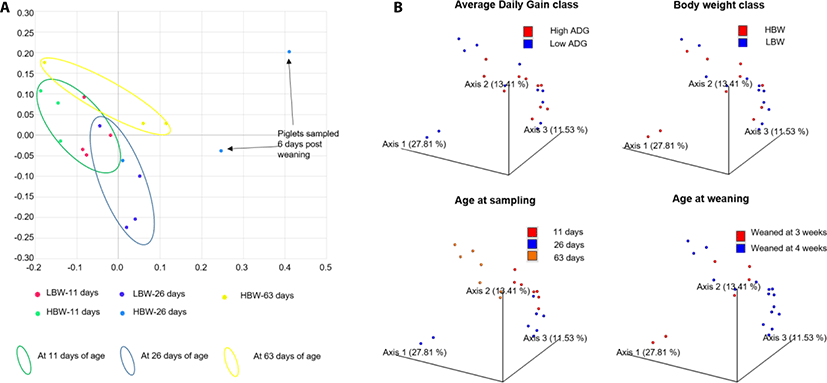
In order to investigate the development pattern influenced by birthweight, we assessed the longitudinal changes in alpha and beta diversity between the birthweight groups (high birthweight, HBW and low birthweight, LBW groups). We found that the Shannon diversity tended to decline between 11 and 26 days of age in both groups of piglets and gradually increased between 26 and 63 days of age (Fig. 3A). Furthermore, we found that the beta diversity tended to change faster in HBW than the LBW piglets during suckling (between 11 and 26 days) (Fig. 3B), however by 63 days of age, the microbiota composition in both groups was relatively equally different from their relative compositions at 11 days of age (Fig. 3C).
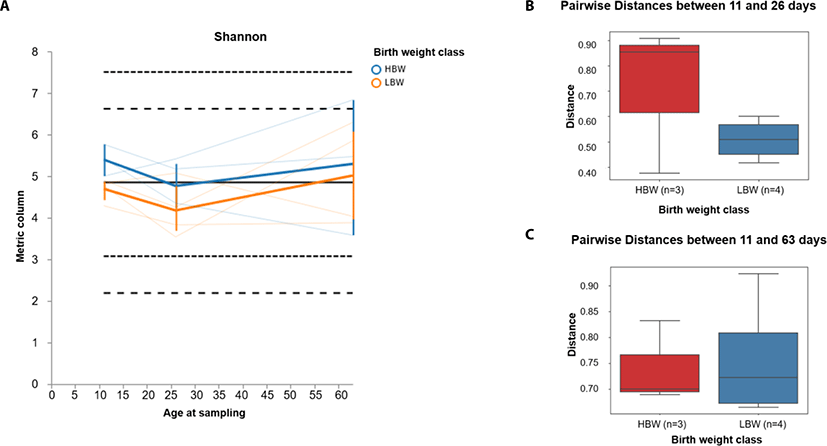
In order to test whether there was an association between the oropharyngeal microbiota and the ADG of piglets, we run a generalized linear regression using the edgeR package in R. We found 9 genera that were significantly associated with ADG at 11 days of age (FDR < 0.05) and 9 genera (FDR < 0.1) at 26 days of age (Table 1 and 2). However, at 63 days of age, no genera were found to be associated with ADG. The association between the microbiota and ADG was stronger earlier in life and reduced post weaning implying that it is potentially more effective to influence weight gain by manipulating the microbiota earlier in pre-weaning life of the piglets.
Nine genera (Veillonella, Aerococcus, Bergeyella, Prevotella, Psychrobacter, Kurthia, Enterococcus, Acinetobacter and Facklamia) within the oropharyngeal microbiota were significantly associated with ADG at 11 days of age (FDR < 0.05).
[ ]; The names in square brackets [ ] within the Greengenes database are still contested at that taxonomic level.
ADG, average daily gain; logFC, log fold-change; logCPM, log counts per million; LR, likelihood ratio; FDR, false discovery rate (adjusted p-value).
Phylla; Cyan – Cyanobacteria, Firm – Firmicutes, Bact - Bacteroidetes, Prot – Proteobacteria. Classes; Nega – Negativicutes, Baci – Bacilli, Flav – Flavobacteriia, Bact – Bacteroidia, Gamm – Gammaproteobacteria, Alph- Alphaproteobacteria. Orders; Vell – Vellionellales, Lact – Lactobacilliales, Flav – Flavobacteriales, Bact – Bacteroidales, Pseu – Pseudomonadales, Baci – Bacillales, Lact – Lactobacillales, Past – Pasteurellales. Families; Veil – Veillonellaceae, Aero – Aerococcaceae, Mora – Moraxellaceae, Past – Pasteurellaceae, Plan – Planococcaceae, Past – Pasteurellaceae, Ente – Enterococcaceae.
Nine genera (Helcococcus, Helcobacillus, Aerococcus, Weissella, Dietzia, Arcanobacterium, and 3 unidentified genera) within the oropharyngeal microbiota were significantly associated with ADG at 26 days of age (FDR < 0.1). Because 3 sequence data of 16S RNA have not been identified in the phylogenetic level of genus in the Greengenes database, they are remarked as 3 unidentified genera.
ADG, average daily gain; logFC, log fold-change; logCPM, log counts per million; LR, likelihood ratio; FDR, false discovery rate (adjusted p-value).
[ ]; The name in square bracket [ ] within the Greengenes database is still contested at that taxonomic level.
Phylla; Firm – Firmicutes, Acti - Actinobacteria, Bact - Bacteroidetes, Fuso - Fusobacteria, Prot - Proteobacteria Classes; Clos- Clostridia, Acti - Actinobacteria, Baci - Bacilli, Bact - Bacteroidia, Fuso - Fusobacteriia, Erys - Erysipelotrichia, Gamm – Gammaproteobacteria. Orders; Clos- Clostridiales, Acti - Actinomycetales, Lact - Lactobacilliales, Bact – Bacteroidales, Fuso - Fusobacteriales, Erys - Erysipelotrichiales, Pseu – Pseudomonadales. Families; Derm - Dermabacteraceae, Aero – Aerococcaceae, Lept - Leptotrichiaceae, Erys - Erysipelotrichaceae, Leuc - Leuconostocaceae, Diet - Dietziaceae, Acti - Actinomycetaceae, Mora – Moraxellaceae.
The association with ADG might be explained better by the metabolic functions performed by the microbial communities in the piglets with diverging ADG’s. To investigate this, we used q2picrust2, which uses 16S rRNA gene sequence data to predict the microbial functional repertoire within the samples based on curated reference databases such as the KEGG [38] and MetaCyc [39]. The output from q2picrust2 analysis included predictions of 6,490 KO metagenomes based on the KEGG’s Orthologs, 1,980 EC metagenomes and 389 MetaCyc pathway abundances within the 24 samples.
PCoA was used to show the distribution of samples based on the Bray-Curtis distances within their composition of KO metagenomes, EC metagenomes and MetaCyc pathway abundances (Fig. S2). From the PCoA plots it could be seen that weaning had the strongest effect on microbial functional profile and that the samples tended to cluster by age.
We used the MetaCyc pathways output from q2picrust2 to identify metabolic pathways that were associated with ADG in the piglets. To do this we again used a generalized linear regression model within the edgeR package in R. We found 45, 10, and 2 metabolic pathways that were significantly associated with ADG at 11, 26, and 63 days of age, respectively (FDR < 0.05). The MetaCyc pathways found to be significantly associated with ADG were cross referenced with the MetaCyc catalogue [39] to identify their names and the super pathway categories to which they belonged (Tables 3–5).
The pathways associated with ADG belonged to the following categories of super pathways; aromatic compound degradation; nucleoside and nucleotide biosynthesis; nucleoside and nucleotide degradation; sugar nucleotide biosynthesis; sugar acid metabolism, Vitamin K2 biosynthesis; amino acid biosynthesis; amino acid degradation; amine and polyamine degradation; amine and polyamine biosynthesis; Secondary metabolite degradation; Cell structure biosynthesis; polymyxin resistance; Fatty acid and lipid biosynthesis; Fatty acid Lipid degradation; Inorganic Nutrient Metabolism; Cofactor, prosthetic group, electron carrier and Vitamin biosynthesis; Generation of precursor metabolite and energy; aldehyde degradation; sugar biosynthesis and Antibiotic resistance (Polymyxin resistance) (Tables 3–5).
The aromatic compound degradation category included pathways that are involved in the degradation of Catechol. Apart from the breakdown of xenobiotics, these pathways are capable of degrading the catechol residue derived from the host’s catecholamines hormones. Within the nucleoside and nucleotide metabolism pathways, we found that pathways involved in biodegradation significantly increased with ADG during the pre-weaning stage (11 and 26 days of age) while their biosynthesis significantly reduced with increasing ADG (Table 3 and 4). In the Amine and polyamine degradation category, pathways involved in the degradation of allantoin and aromatic biogenic amines increased with ADG while biosynthesis of ectoine decreased with ADG (Table 3). Allantoin is a by-product of purine degradation and therefore it’s degradation is connected to the microbes’ degradation of nucleotides and nucleosides as a nitrogen source [40,41]. We also observed an increased representation of pathways involved in the degradation of D-glucarate and D-galactarate in high ADG piglets implying that organisms associated with high rates of weight gain have the ability to degrade sugar acids and utilize them as a carbon source for growth (Table 3 and 4).
DISCUSSION
This study investigated the association between the oropharyngeal microbiota and the piglets’ birth-weight and rate of weight gain. Results revealed microbial clades and pathways that were associated with the piglets’ weight gain. However, the associations were stronger in the pre-weaning phase and appeared to wane with age implying that an attempt to improve weight gain through modulation of the microbiota should target early life of the piglet, particularly before 4 weeks of life.
Our findings from the taxonomic analysis of the oropharyngeal microbiota were largely in agreement with studies focusing on the tonsillar microbiota [36,42,43]. However, the dominance of Streptococcus, in our study, was striking since it was only represented as a minor community member within the tonsils [42]. It has been found that the catecholamine stress hormones, norepinephrine (NOR) and dopamine (DOP), induce the proliferation of some species of Streptococcus [44]. In our study, the piglets were raised under a commercial farm setting, which is characterized by cross fostering, weaning and frequent regrouping of the piglets. These events involve changing the piglets environment and disruption of their social structure, consequently elevating the levels of circulating catecholamines [45]. In addition, Pasteurella, which was found to dominate in the tonsillar microbial communities [42] had a surprisingly low relative abundance among our samples (1% or less). These slight differences can partly be explained by the fact that the above studies focused on the tonsillar microbiota while this study included pooled samples of the oropharyngeal cavity.
We did not find any statistically significant difference between the development of oropharyngeal microbiota in HBW and LBW piglets. However, we found subtle differences that tended to disappear by 63 days of life implying that birthweight only had a slight effect on the oropharyngeal microbiota during the first few weeks of pre-weaning which gradually wanes as the piglets grow older. In contrast to our findings, studies done in the piglets’ gut have found statistically significant differences between the microbiota of low birthweight and normal birthweight piglets during the pre-weaning phase [46,47]. However, these differences also tended to disappear by 35 days of age [46].
The current trend in microbiome research has been to explore the microbial metabolic functions that may have clinical, therapeutic or nutritional relevance to the host [48]. Previous studies in domestic pigs have reported correlations between feed efficiency and the illeal, caecal, colonic and fecal microbiome [49–51]. In this study, we explored the association between the predicted metabolic pathways within the oropharyngeal microbiome and ADG in the piglets. Our results showed an enrichment of pathways involved in the biodegradation of nucleosides and nucleotides in heavier piglets during the pre-weaning phase. The reason for this association is not clear, however one possible explanation could be, that the relationship is a consequence, and not a cause, of the ADG among the piglets. It is known that sow milk, like all mammalian milk is a very rich source of nucleosides [52]. It is therefore possible that, as a result of their competitive advantage, the larger piglets have more access to the best teats and also spend more time suckling [53] resulting in a consistently higher concentration of sow milk-derived nucleosides within the saliva matrix in their oropharynx. This would then favor the proliferation of bacteria with the ability to utilize nucleosides and nucleotides. On the other hand, members of the oropharyngeal microbiota within the lighter piglets have to biosynthesize their nucleotides and nucleosides to sustain their growth and proliferation.
The association of catechol degradation pathways with ADG, suggests an enhanced ability to degrade host derived catecholamines within the oropharyngeal microbiome of piglets having high rates of weight gain. Catecholamines, which include Dopamine (DOP), Epinephrine (EPI) and Norepinephrine (NOR), are secreted during stressful conditions [54]. It is likely that the high ADG piglets have higher appetite levels compared to the low ADG piglets and are therefore more inclined towards aggression and competition for feed with their pen mates leading to higher levels of stress hormones (EPI and NOR) within their circulation [55,56]. Circulating catecholamines are partly secreted into the saliva matrix and this may lead to an accumulation of bacteria, within the oropharynx, that have the ability to degrade catechols. Catecholamines are also involved in the regulation of hedonic feeding in mammals [57]. Hedonic feeding refers to the non-homeostatic feeding that is not driven by energy requirements but simply driven by pleasure or desire to consume palatable feed [58]. Some catecholamines have been shown to induce feeding behavior such as food-anticipatory activity, search for food and motivation to feed [59]. Breakdown of these catecholamines, interferes with systemic regulation of their production [60]. Interfering with the feedback control of the central catecholamine release could then lead to a sustained secretion and consequently, a sustained appetitive feeding in pigs thus contributing to attainment of higher rates of weight gain.
In summary, we identified microbial biomarkers within the oropharyngeal microbiome that may be used as targets for modulating rates of weight gain in piglets. However, our study does not confirm whether the detected microbial signature is a cause or an effect of the ADG therefore, requiring further studies. In future, studies that employ ‘omics’ techniques are needed in order to better understand the roles played by this oropharyngeal microbiota. Combining techniques that can reveal the active genes (transcriptomics), synthesized protein molecules (proteomics) and their metabolic products (metabolomics) could provide deeper insights into the role of this oropharyngeal microbiome.