INTRODUCTION
In Korea, genetic evaluation was performed with data obtained from progeny testing and commercial data to improve carcass and meat quality traits of Hanwoo beef cattle [1]. Recently, a genetic breeding program has started, and many studies assess the use of genomics in small sample sizes [1–7]. Higher to moderate heritabilities were reported for most carcass traits and marbling scores (MS) [2–5,7–12]. Other traits such as meat and fat colour (FC) were moderate to lower heritable in Hanwoo cattle [2,3,5,7–11]. Carcass and meat quality traits have a major influence on the profitability of beef production but represent a challenge since they are often hard to measure and select for. This is because carcass traits cannot be recorded on selection candidates and therefore time-consuming progeny tests are often used to gain selection accuracy. Due to this cost, breeders often look to commercial animals recorded for carcass performance. Performance records on commercial animals are interesting because the large number of animals being harvested, which presents opportunities to improve accuracy at a perceived low cost [13]. However, there are often drawbacks to this commercial data due to the production management strategies. For instance, animals are usually recruited or sorted into management groups according to their weight, and similarly, they arrive in abattoirs in homogenized cohorts. Such selective formation of contemporary groups referred to as ‘harvesting’ could lead to biased evaluation of genetic merit.
Sources of bias in genetic evaluation have been discussed widely in literature [14]. The main sources of bias in animal breeding are non-random selection of animals for breeding (parental selection), sequential selection, culling of animals before records, preferential treatment, selective reporting, and misclassification or manipulation of contemporary groups [15]. Regardless of the source of information, genetic evaluation methods can be used to account for non-genetic effects [14]. Various methods have been deployed for genetic evaluation in livestock and mixed model evaluation of single and multiple traits [16–19]. For unbiased genetic evaluation, fixed effects, such as a contemporary group, and covariates such as age, live weight and carcass weight (CWT) are fitted in the model to account for non-genetic factors [20]. For instance, adjustment of slaughter endpoints such as harvesting age and weight has an impact on genetic evaluation, Pollott et al. [21] found that the heritability for daily CWT gain was higher with slaughter at fixed weight (h2 = 0.63 ) than at fixed age (h2 = 0.11), indicating that animals can attain similar slaughter weight at different age. In general, field data are consistently provided by herds in which artificial selection is continuously occurring and where heavier animals are sent first to slaughterhouses. Consequently, the usual assumption of random sampling invoked for estimation and prediction [22] is no longer valid in genetic evaluation. The extent of a possible bias in genetic evaluation can be evaluated using simulated data instead of field data. The first component of this study aimed to estimate genetic parameters for carcass and meat quality traits in Korean Hanwoo cattle using industry-based carcass performance records. Following, we aimed to assess the impact of sorting animals based on early measured traits on the genetic evaluation of subsequently measured traits using simulated data.
MATERIALS AND METHODS
All phenotypic and pedigree data used in the present study were obtained from the Korea. Individuals without a record of sire or dam were discarded from the data. After filtering the raw data, 469,002 Hanwoo cattle from 3,464 distinct farms were used in the analysis. The pedigree file comprised 1.23 million ancestors over 13 generations, including 646 sires and 390,166 dams in the analysis. The animals were born between the year of 2008 and 2014, and slaughtered between the ages of 28 and 35 months. All studied traits were recorded between the years 2010 and 2016.
A preliminary analyses of variance was performed using the linear model in R [23] to identify the most appropriate fixed effects (contemporary groups) to be used in the subsequent analyses for all studied traits. The most significant factor was the interaction between herd (3,646 herds), birth-year (7-levels), and birth-season (4-levels), which were combined to form the contemporary group. The final dataset consisted of 469,002 animals from 31,403 contemporary groups (Table 1). A contemporary group of herd-year-season (HYS) with less than five records was omitted from the analysis. The distribution of animals across various ages and herds, as well as animals born in each year, and season, are shown in Fig. 1. The description of population structure, as well as the distribution of animals across age, herd, birth year, and season are summarized in Table 1 and Fig. 1.
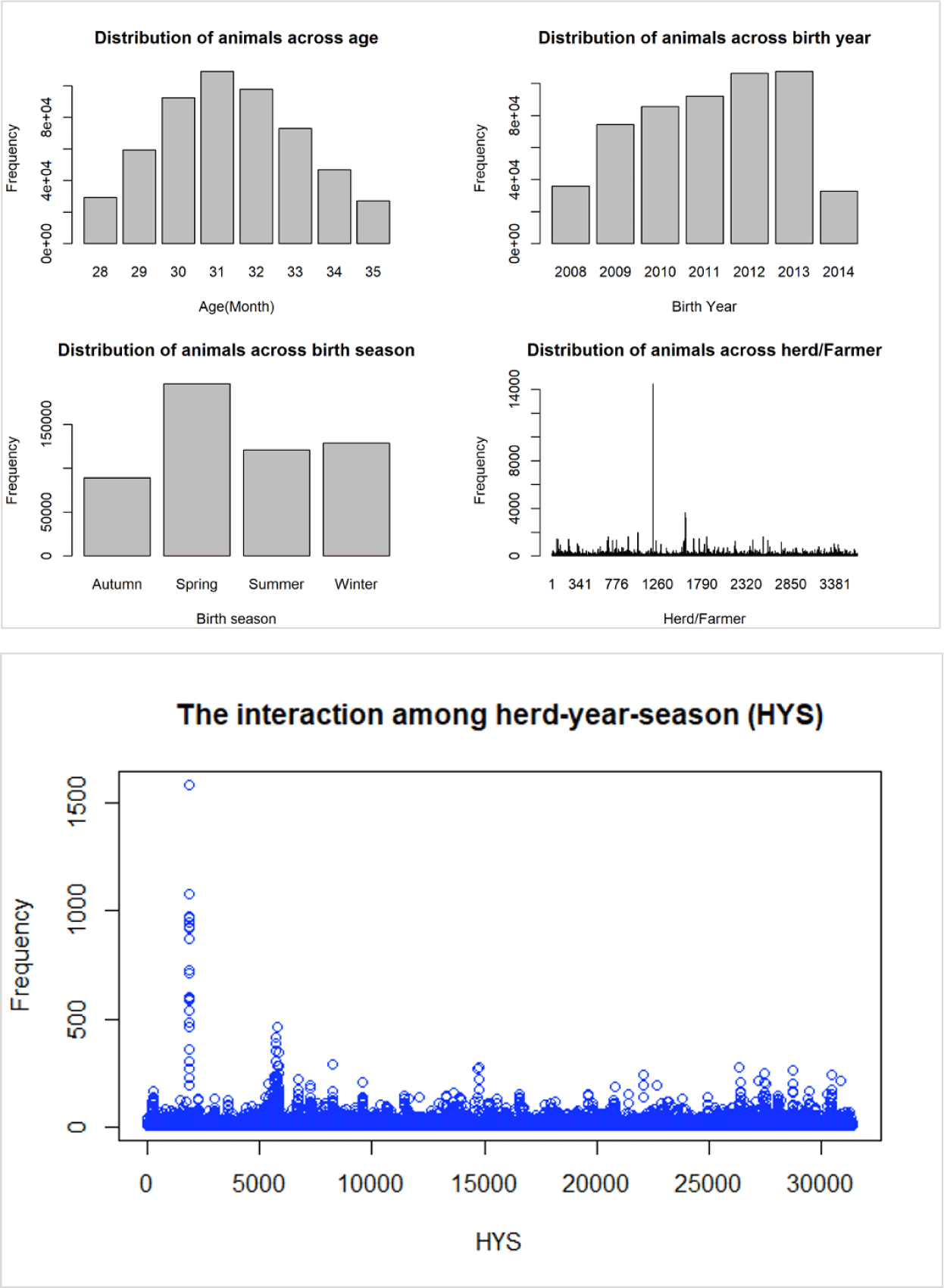
All individuals were slaughtered at various ages (28–35 months), and phenotypic measurements were taken on the chilled carcass. Phenotypic data on carcass traits included CWT, eye muscle area (EMA), backfat thickness (BFT), bodyweight (BW) at slaughter, and meat-index (MI). EMA and BFT were measured at the 12th and 13th rib junction after a 24-h chill, and cold CWT measurement was taken at that time. The fasted live BW in kilogram was taken prior to transport to the abattoirs. Only 52% of animals in the dataset had BW records at slaughter. The MI represents the retail cut percent, which is predicted from a linear index of CWT (kg), EMA (cm2), and BFT (mm). MI=68.184 − [0.625 × BFT] + [0.130 × EMA] − [0.024 × CWT] + 3.23.
Meat quality traits included MS, meat colour (MC), FC, and meat texture (MT) which were each graded at the time when carcass traits were measured. MS was recorded based on visual inspection by trained technicians using the Beef Marbling Standard (BMS) for grading the carcass. The BMS system classifies the meat from 1 to 9 scale of marbling, with a 2% intramuscular fat content difference between each point score [24]. Similarly, trained technicians graded the other three traits (MC, FC, and MT) manually. MC was assessed and graded from very light red (grade 1) to dark red (grade 7). Similarly, the FC was assessed and graded from polar white (grade 1) to creamy yellow (grade 6). The texture of the meat was evaluated on a scale from very fine (grade 1) to coarse (grade 3) (Table 2).
Variance components and heritabilities were estimated with a series of univariate animal models. For each trait, the model included the contemporary group (as defined above) fitted as a fixed effect and age fitted as a linear covariate in the model. Genetic and phenotypic correlations were estimated using a bivariate model with similar fixed and random effects as the univariate model. ASReml version 4.1 software [25] was used for the entire data analysis. Prior to the main analysis, we performed a model comparison between a model with and without maternal (permanent environmental) effect. Based on the Akaike information criterion (AIC) value, the model without maternal effect best fitted the data [26]. The model was represented as:
where X is an incidence matrix for observations y. Age as a linear covariate and contemporary groups (HYS) as a fixed effect in vector b, Z is an incidence matrix for random animal additive genetic values in u, and e represents random environmental effects. u and e were assumed to be distributed as , , respectively where is the additive genetic variance and is the residual. Where A is a numerator relationship matrix for all animals using 13 generations and I is an identity matrix.
A stochastic simulation was used to study how the genetic evaluation of the carcass traits was affected when the animals were sorted based on early age performance traits such as yearling weight. A beef cattle population was simulated with the input parameters described in Table 3, using R [23]. The simulated base population consisted of 100 sires and 2,000 dams, which were assumed unrelated and not in-bred. The mating ratio of sire to dam was 1 to 20. A total of 4,000 progeny were produced in a single generation. All progenies had an early age measurement (EM) for yearly BW and were subsequently measured for carcass traits later in the production cycle (Late measurement [LM]). All progenies were split into 10 contemporary groups, each with 400 animals. The contemporary groups were generated using two alternatives to sort the progeny; 1) based on the early trait measurement (EM), 2) randomly assigned to the contemporary group. The simulated data was consisted of ten replications for each scenario. In each scenario, the genetic evaluation was performed on carcass traits (LM). To examine the range of possibilities for EM and LM traits, a variety of heritability and correlation values were used for data simulation (Table 3).
Linear mixed models were used to estimate the breeding values and variance components [16] implemented in ASReml version 4.1 [25] using the model described in equation 1. The fixed effect (contemporary group) was fitted only to the LM trait in the sorted scenario. The bias in the variance of the estimated breeding values (EBV) was measured through the regression coefficient (slope) of the true breeding values of 100 base sires on their EBV in each replication. The estimated bias in EBV was the mean of the 10 replications.
RESULTS
Variance components and heritabilities for the studied traits are shown in Table 4. In the current study, the estimated heritabilities for carcass traits ranged from 0.37 for EMA to 0.45 for MI. However, meat quality traits showed low to high heritability and the estimates ranged from 0.004 for FC to 0.55 for MS. The phenotypic and genetic correlations between studied traits are shown in Table 5. The genetic and phenotypic correlations within carcass traits varied from 0.05 to −0.94, and 0.03 to −0.94, respectively. Similarly, the genetic and phenotypic correlations within meat quality traits ranged from 0.42 to -0.94, and 0.04 to −0.61, respectively. The genetic correlations between carcass and meat quality traits varied from low (0.04) to medium (0.44). All correlations were estimated with small standard errors ranging from 0.00 to 0.08.
As expected, the random allocation of animals to contemporary group was unbiased; however, bias was observed when animals were sorted into contemporary group based on EM traits. The degree of bias varied depending on the heritability and the correlations between EM and LM traits.
As indicated in Fig. 2A, the EBV was biased (overestimated) when the genetic and residual correlations between the simulated traits were −0.8 and 0.8 respectively. Slight underestimated EBV was observed when the genetic and residual correlation between the EM and LM traits was higher (0.8). These results indicated that the bias was higher for the sorting scenario when the correlation between the two studied traits was higher. But slightly biased EBV was observed when the genetic and residual correlation was lower (−0.2 and 0.2) (Fig. 2A). The result showed that changes in genetic and residual correlations affected the magnitude of bias in the EBV. The current results showed that the EBV was highly biased when the correlation between the sorting (EM) and the subsequent evaluation (LM) trait was higher, and sorting bias was lesser when the correlation between the two traits was weaker. At higher genetic (0.8) and residual (−0.8) correlations, considerably overestimated EBV with a bias of 0.54 was observed (Fig. 2D). However, less biased EBV was observed when the residual correlations were lower (Fig. 2D). Overall, overestimated, and underestimated breeding values were observed at the combination of high genetic and residual correlations (Fig. 2D). With similar genetic and residual correlations, a slight increment of bias was observed when the heritabilities of both simulated traits changed from high (0.5) to low (0.2).
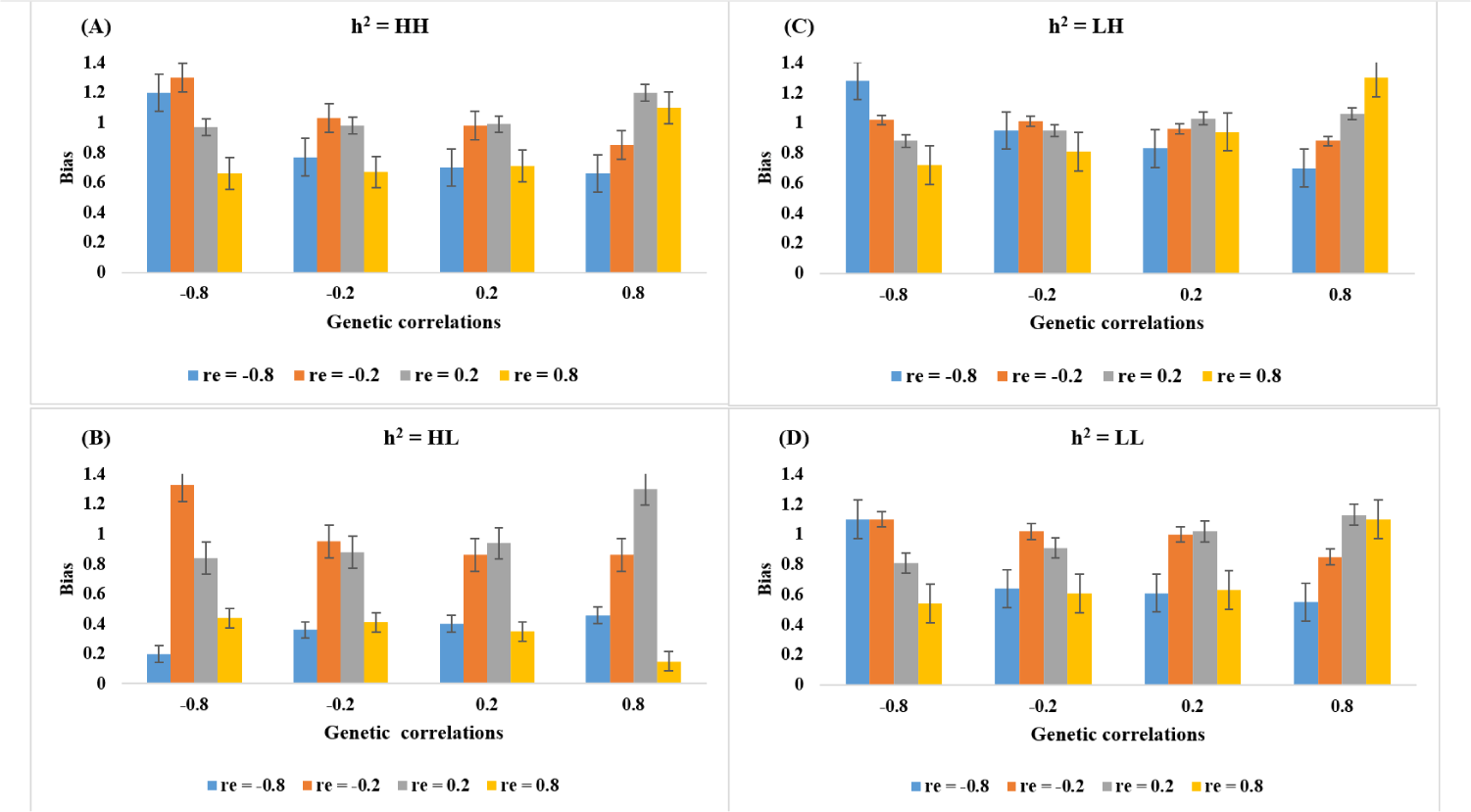
We assessed the bias generated by sorting animals in the genetic evaluation using traits with different heritabilities (high-low and low-high). In the randomly sampled scenario, the regression coefficients (slopes) in all alternatives were equal to one and no change of bias was observed with changing of input parameters including heritability of the traits (results not shown). However, we observed different magnitudes of bias with the changing of input parameters between the two studied traits when animals were sorted into various contemporary group depending on the EM trait (yearly weight). Highly biased EBV of 0.15 (±0.01) and 0.20 (±0.02) were observed when genetic and residual correlations were 0.8 and −0.8, respectively (Fig. 2B). These highly biased EBV (overestimated) were observed when the heritability of the LM trait (evaluation trait) was low (0.2). However, relatively less biased EBV were observed when the heritability of the LM trait was higher (Fig. 2C). The highly biased EBV with low heritability of LM trait indicated that at constant genetic and residual correlations, genetic evaluation is further affected by the heritability of the evaluation (LM) trait than the sorting (EM) trait. Relatively less biased EBV was observed at low correlations with both high and low heritability of the evaluation (LM) trait (Figs. 2B and 2C). The low genetic and residual correlations may be responsible for the less biased EBV even though the heritability of the LM trait varied. Overall, with the low heritability (0.2) of the LM trait, the EBV was highly biased (overestimated), however, when the heritability of the LM trait was higher (0.5) the EBV was less biased. In addition, the bias of EBV was less when the difference between the genetic and residual correlations was lower whereas highly biased EBV was observed when the difference between the two correlations was bigger. Overall, our results indicate that the magnitude of the sorting bias depends on the genetic architecture of the two traits.
DISCUSSION
The estimated heritability for CWT was 0.44±0.01, which is higher than most other reports in Hanwoo where the reported estimates ranged from 0.25 to 0.39 [9,11,27,28]. The main reason for the difference is the weights of the animals used in the current study were obtained from commercial farm and had higher weight. Similarly, the heritability of EMA in the current study was higher (0.37±0.01) than estimates in previous reports which ranged from 0.27 to 0.36 [8, 11]. The estimated heritability for BFT in the current study (0.44±0.01) is very close to previous reports on Hanwoo cattle ranging from 0.44 to 0.45 [2,9,11]. However, the MI trait showed high heritability compared to previous findings (0.26) reported by Do et al. [8] in Hanwoo cattle. This is because the MI trait in the current study was predicted from carcass traits such as CWT which were obtained from commercial data. In Hanwoo cattle, previously reported heritabilities for meat quality traits ranged from 0 for FC to 0.65 MS [2,8,9,11,27,28].
Slight variations have been observed between current and previous estimated heritabilities in Hanwoo beef cattle. These inconsistencies mainly have arisen from the data type used in each report. Most of the previous reports used small data set obtained from well-deigned progeny testing experiments that showed low variation compared to the data used in the current study obtained from slaughterhouses. Compared to previous reports, the data used in the current study is larger (half a million animals) and were obtained from more than three thousand farms in the Korea. Types of information such as pedigree and/or genomic data used in the analysis may lead to different values of parameter estimates. Some traits such as MS had different heritability estimates in different breeds because the traits have shown different genetic variation in different populations. For instance, the currently estimated heritability for MS was 0.55 and it is higher than the estimated in other beef cattle breeds reported by Davis and Simmen [29] (0.27±0.17), Ríos-Utrera et al. [30] (0.40±0.09) and Nephawe et al. [31] 0.46±0.06 in Angus and US purebred and composite steers respectively.
In the current study, CWT showed moderate and positive genetic (0.51) correlation with EMA, which is lower than the previous estimates ranged from 0.63 to 0.80 in Hanwoo cattle [8,9,28]. In agreement with the current results, Hwang et al. [9] reported a negative genetic correlation (−0.24) between EMA and BFT in Hanwoo cattle. In the current study, the estimated phenotypic and genetic correlations between CWT and BFT were 0.27 and 0.05, respectively; however, with small data set, Do et al. [8] reported a lower genetic correlation of 0.17 and higher phenotypic correlation of 0.31 between CWT and BFT. The highest genetic (0.94) and phenotypic (0.93) correlations were observed between BW at slaughter and CWT in the current study. These two traits are extensively studied in other breeds and they show high correlation [32]. Low genetic correlations of BW with EMA (0.30) and BFT (0.10) had been reported by Smith et al. [32] in Brahman cattle, which is in range with the current study. In the current study, the genetic correlation of MI with CWT was lower and moderate with EMA while higher (−0.94) with BFT. In agreement with our current study, high negative genetic (−0.95) and phenotypic (-0.97) correlations between MI and BFT had been reported in Hanwoo cattle [8]. This is because MI trait was predicted from the three traits (CWT, EMA and BFT), as a result, MI showed a high genetic and phenotypic correlations with CW, EMA and BFT traits.
In the current study, genetic and phenotypic correlations between meat quality traits varied from high to low. Previously, similar genetic and phenotypic correlations were reported between MS and MT traits in Hanwoo cattle using pedigree and genomic data [4,5,8], however, the phenotypic correlation between MS and MT reported by these authors were lower compared to current estimates. The high genetic correlation between MS and MT traits suggests that the traits are highly dependent. Given this strong correlation and the fact that MS has more genetic variation (high heritability), it is the easiest trait to use to select for high meat quality or both traits can be improved simultaneously. Compared with the current study, Do et al. [8] reported lower (−0.42 and −0.40) genetic correlations of MS with meat and FC traits, respectively using small sample size data obtained from progeny testing experiments of Hanwoo cattle. Slightly higher genetic correlation between colour traits (MC and FC) was reported using genomic data in Hanwoo cattle [4]. The genetic correlations of MT with MC and FC traits in the current study were similar to the previous reports in Hanwoo cattle [4,8]. In the current study, we observed low genetic correlation between carcass and meat quality traits. Similarly, several studies reported low genetic and phenotypic correlation estimates between carcass and meat quality traits in Hanwoo cattle [8,9,11,27].
Overall, the currently estimated genetic parameters laid within the range of previous reports in Hanwoo cattle, however, slight differences have been observed for some traits. The main reasons for the observed difference are the sample size used in the study, source of performance data (commercial farms versus well-designed progeny testing experiments) and type of the information used to estimate the relationship among animals (pedigree versus genomic data). In addition, various models were used to analyse the data, the random and fixed effects that are fitted in the model, and the random or non-random allocation of animals into contemporary group leads to different value of parameter estimation in the genetic evaluation of carcass traits. Furthermore, the scoring or grading system of meat quality is very subjective across studies and has an impact on the genetic evaluation of such traits.
The phenotypic data used in the current study was obtained from slaughterhouse and these carcass performance data were recorded on various age groups of animals ranged between 28 and 35 months. The estimated parameters in the current study are closer to previously reported estimates obtained from progeny testing experiment. This is indicating that commercial data obtained from slaughterhouse are useful and can be used to estimate genetic parameters in the evaluation of carcass traits. The commercial carcass performance data can be available in large amount from slaughterhouse compared to progeny testing experiment data, but we have observed some drawback in the commercial data. This is because, in most commercial farms, the desired carcass quantity and quality is specified by abattoirs based on the weight of animals, which is suitable for slaughter. As a result, most animals have arrived in abattoirs in homogenized weight which is called harvesting effect. Such selective formation of contemporary groups might lead to biased evaluation of genetic merit of animals for carcass trait. However, this type of bias in the genetic evaluation can be controlled using various models depending on the source of the bias. For instance, bias due to sorting of animals based on weight can be reduced or eliminated using multitrait genetic evaluation methods. The multitrait model can account for harvesting effect and thus produce more accurate and less biased parameter estimation in genetic evaluation.
Sorting of animals based on yearling weight potentially affected the genetic evaluation of the latter measured traits. The current study has verified that the non-random evaluation of animals led to a bias in genetic evaluation.
The sorting bias observed in the current simulation study has a similar effect to the selection bias described in the literature [14,15,33]. The theory of selection bias was established clearly by Pollak et al. [15]. They demonstrated that potential bias was found in the genetic evaluation of the second trait, which was selected based on the first trait. Similarly, in the current simulation study, when animals were sorted based on the early measured trait, sorting bias was detected in the subsequent evaluation of the latter measured trait. Also, Pollak and Quaas [33] observed a selection bias in the predictors in single-trait analysis and the magnitude of the bias of the EBV depends on the correlation among studied traits which is in agreement with the current findings.
A recent study by Macedo et al. [34] has studied a selection bias in genetic evaluation models. They found a selection bias in genetic evaluation using data with environmental trends compared to randomly sampled data into different contemporary groups. Similarly, in the current study, we found biased EBV when animals were non-randomly assigned into various contemporary group. Selection bias has been studied in sheep, Eiríksson and Sigurdsson [35] demonstrated that a selected group of the lambs was kept for replacement and therefore not measured for carcass traits, led to bias in the genetic evaluations. The authors demonstrated that the bias was higher for the selected ram group, indicating that this group consisted of rams that had higher genetic merit for carcass conformation trait than the rest groups. Similarly, we found biased EBV when animals with higher yearling weight were assigned into same group.
In the current simulation study, the structures of input parameters including heritability, genetic and residual correlations, and the difference between the two correlations influenced the genetic evaluations. The genetic and phenotypic correlation of traits potentially affects the genetic evaluation of animals in breeding program [15,33,36,37]. Our results showed that highly biased EBV was observed when the genetic correlations were higher which coincided with a report by Pollak and Quaas [33]. In addition, our result showed that highly biased EBV was observed when the difference between genetic and residual correlation was higher. Conversely, when the difference between genetic and residual correlation was smaller, the EBV was less biased and the regression coefficient was closer to one.
In the current simulation study, the bias of the EBV was assessed by allocating unequal heritabilities (high-low and the vice versa) for the two simulated traits. In this case, the observed bias of the EBV was not the same as a similar heritability (high-high and low-low) was assigned to the studied traits. Highly biased EBV was observed when the heritability of the LM (evaluation) trait was lower. This could be explained as genetic evaluation is more affected by the heritability of the LM trait than the EM (sorting) trait with the constant genetic and residual correlations. A recent study showed that allocating wrong heritability for the trait in a genetic evaluation led to a biased EBV in simulation study [34]. These authors established that in pedigree-based predictions, the use of incorrect heritability generates a strong bias in genetic evaluation using simulation data. Our result showed that different magnitude of bias was observed with two different heritabilities assigned to the LM (evaluation) trait. However, we have not proven that the observed bias linked to incorrect heritability that was allocated to the evaluation trait during data simulation. Knowing the source of bias and then using appropriate models for the genetic evaluation of animals is a key component of the process to estimate accurate breeding values. For instance, to overcome selection bias in genetic evaluation, multivariate evaluations methods had been proposed [16]. The decision to use a multitrait model versus a single trait model depends on correlations among the studied traits. In the first scenario of the current study, the sorting bias that was observed in the single-trait model was reduced by the bivariate model (results not shown). Similarly, Pollak et al. [15] showed that the observed bias associated with selection in the univariate model was reduced or eliminated by multiple traits evaluation methods.
A recent study by Macedo et al. [34] has studied a selection or sorting bias in genetic evaluation models. They found a selection or sorting bias in genetic evaluation using data with environmental trend compared to a randomly sampled data into different contemporary groups. Similarly, in the current study, we found biased EBV when animals were non-randomly assigned into various contemporary group. Selection bias has been studied in sheep, Eiríksson and Sigurdsson [35] demonstrated that a selected group of the lambs was kept for replacement and therefore not measured for carcass traits, led to bias in the genetic evaluations. The authors demonstrated that the bias was higher for the selected ram group, indicating that this group consisted of rams that had higher genetic merit for carcass conformation trait than the rest groups. Similarly, we found biased EBV when animals with higher yearling weight were assigned into same group.
CONCLUSION
The genetic parameters estimated for carcass and meat quality traits in Hanwoo cattle population indicate the extent of genetic variability among the studied traits that could be exploited through selection programs. Particularly, carcass traits showed high genetic variation in Hanwoo population. On the other hand, results from the current study have revealed the existence of negative (unfavorable) genetic associations between a carcass and most of meat quality traits. This implies that long-term selection for carcass traits could negatively affect meat quality traits, which are highly valuable in Hanwoo cattle. Moderate genetic correlations between EMA and MS suggest that genetic progress for carcass traits such as EMA may result in more marbled meat. This is important because, marbling is the major price-determining factor in the Korean beef industry. Very low or near-zero correlations between CWT and most of the meat quality traits, suggest that selection based on CWT may have little or no influence on the performance of meat quality traits. In addition, negative genetic and phenotypic correlations between carcass and few meat quality traits reflect the adverse effects in a single trait selection program. Commercial data obtained from slaughterhouse is a potential source of carcass performance data and useful for genetic evaluation of carcass traits to improve beef cattle performance. However, using commercial data could produce biased EBV because in this data, animals are mainly sorted based on live weight prior to slaughter and this non-random selection of animals to slaughterhouse affects the genetic evaluation of carcass traits which is obtained from abattoir. Overall, the current simulation study contributed fundamental information on harvesting effect and how the genetic architecture of studied traits affects the genetic evaluation of carcass traits.