INTRODUCTION
As the population grows, so does the demand for meat and, in turn, the density of livestock farms. This significantly impacts the lives of the animals raised on these farms, particularly pigs, which are a crucial source of animal protein worldwide [1]. Animal products, including meat and milk, contribute approximately 33% of the protein consumed by humans [2], and pork is the second-most highly consumed meat globally, valued for its affordability and relatively low cost [3]. Several factors influence pig farming, including the farm size, livestock-raising methods, environmental conditions, disease management, and feed consumption rate [4]. Pigs raised under different conditions exhibit distinct behaviors and growth patterns. Those reared intensively tend to grow larger and reach maturity more quickly than their counterparts raised in diverse environments [5]. However, intensively raised pigs often show aggressive, abnormal behaviors, such as navel stabbing and tail and ear biting [6]. Moreover, diseases are responsible for 25% of pig deaths globally [7], and ensuring pig health is vital for production success, necessitating vigilant monitoring for any infections on the farm [2]. Early detection of health issues is crucial, with diseased pigs promptly identified, isolated, and treated to prevent spread.
Over the years, the pig farming industry has witnessed remarkable advancements in management practices and technology adoption to improve efficiency, productivity, and sustainability [8]. Among these technological innovations, acoustic-based precision technologies have emerged as a promising approach for enhancing management practices in pig farming [9]. Utilizing acoustic-based precision technologies involves deploying sound sensors and data analytics tools to monitor various aspects of pig behavior, health, and environmental conditions through the analysis of sound waves and vocalizations [10]. These technologies offer numerous advantages, including non-invasiveness, continuous monitoring capabilities, and the ability to detect small changes in pig behavior and welfare indicators [11, 12]. Accordingly, they have received attention from researchers, industry experts, and policymakers aiming to improve pig farming efficiency while prioritizing animal welfare and environmental sustainability.
Pig vocalizations can be powerful indicators of their health, particularly with regard to respiratory infections, which are a major concern in intensive farming [13]. Coughing is a prevalent symptom of respiratory diseases, which are often marked by distinct sounds upon airway expulsion, serving as the bodily responses against respiratory infections. These sounds can aid in screening and diagnosis, as they give initial indications of various airway and lung conditions manifesting early in respiratory illnesses [14,15]. To maximize this potential for screening and diagnosis, we require equipment capable of collecting and analyzing livestock data effectively. Current research into acoustic-based precision technologies for pig farming encompasses a diverse range of applications, including health monitoring [16–19], behavior analysis [10, 20–22], environmental monitoring [23–25], and automated management systems [26–29]. Studies have demonstrated the effectiveness of acoustic sensors in detecting early signs of disease, monitoring behavior, improving reproductive efficiency, and mitigating environmental stressors. Furthermore, advancements in sensor technology, data analytics, and machine learning algorithms have expanded the capabilities of acoustic-based systems, enabling more accurate and efficient monitoring and management of pig production systems.
While acoustic-based precision technologies hold promise, their application in pig farming faces challenges, including the difficulty in collecting data accurately amid environmental noise, issues with interoperability, high initial costs, and privacy concerns. Additionally, integrating acoustic technologies into pig farming demands careful planning, farmer training, and adaptation. These factors must all be addressed in order to unlock the technologies’ potential to improve pig farming efficiency, welfare, and sustainability.
Early detection of illnesses and assessment of their severity on farms are invaluable to farmers as they facilitate prompt interventions and management. Sound analysis may play a major role in these regards, facilitating the recognition of signs of distress and the classification and grading of various respiratory diseases and their symptoms. Acoustic techniques offer distinct advantages over alternative sensing technologies, such as imaging, thermal, laser, and motion sensors, as they are cost-effective, non-invasive, and allow for the simultaneous monitoring of numerous animals without disrupting their natural living conditions [30]. In recent years, there has been growing acknowledgment of the value of sound analysis for comprehending animal behavior, health, and well-being. Against that background, the objectives of this review were to provide an overview of the current trends and advancements in acoustic-based precision technologies for pig farming, analyze the strengths and limitations of the existing research, identify gaps in our knowledge, and propose future research directions. The objectives of this review were to provide an overview of the current trends and advancements in sound-based precision technologies for pig farming, analyze the strengths and limitations of the existing research, identify knowledge gaps, and suggest future research directions.
PRINCIPLES AND THEORY OF SOUND-BASED PIG HEALTH MONITORING
Sound is a form of energy produced by vibrations that travel through a medium, typically air but also liquids and solids [31]. These vibrations create pressure waves that propagate through the medium. When they reach the ears, they cause the eardrums to vibrate and the brain interprets that as sound; alternatively, the pressure waves are detected by instruments [32]. Sound waves are represented graphically through waveforms, showing changes in air pressure over time. In a simple sine wave, the height of the waveform represents the amplitude (loudness) of the sound, while the length of each cycle represents the frequency (pitch) of the sound (Fig. 1A). Digital sound is converted from analog through sampling, where each sample represents the amplitude at a specific moment. These samples are then stored as binary data in digital audio files, with fidelity determined by the sampling rate and bit depth (Fig. 1B). A spectrogram visually represents the frequency spectrum of a sound signal over time, displaying intensity through color or grayscale shading. Time is shown on the horizontal axis, and frequency on the vertical axis (Fig. 1C).
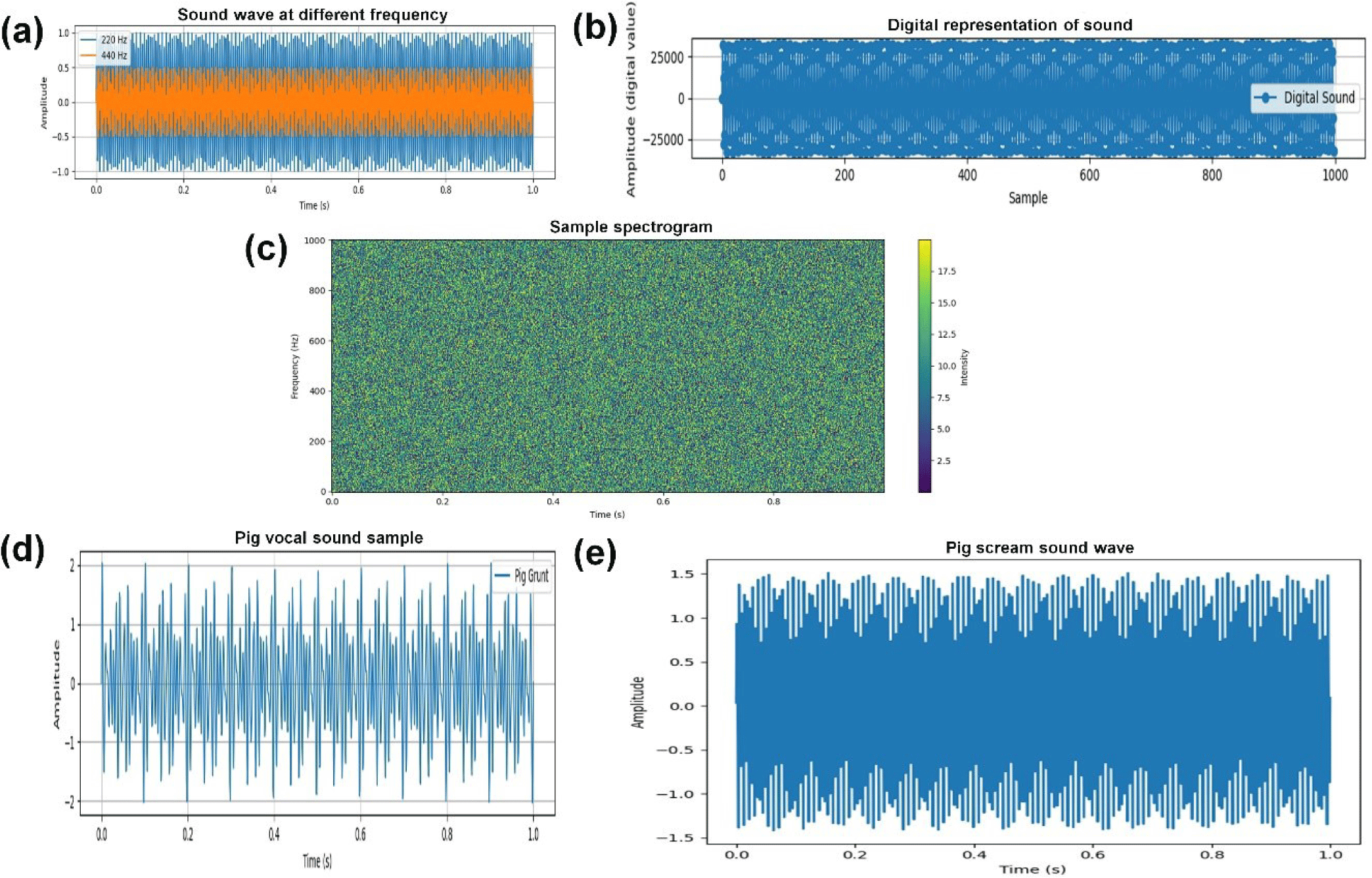
Animal sounds refer specifically to those animals produce as means of communication, defense, mating, or expressing emotions [33]. Animal sounds can vary greatly in frequency, amplitude, duration, and complexity, depending on the species and the context [34]. Many animals utilize vocalization, employing specialized structures such as vocal cords or syrinxes. The complexity of these sounds ranges from simple calls to intricate songs (Fig. 1D). Animal sounds possess distinct acoustic features such as the frequency range, duration, amplitude modulation, and spectral composition, which are crucial for communication and convey information about the animal’s identity, location, and behavior (Fig. 1E). Bioacoustics is a field dedicated to studying animal sound production and behavior, for which techniques such as audio recording and spectrogram analysis are applied [35]. Through bioacoustics studies, researchers may gain valuable insights into animal behavior, ecology, and evolutionary processes.
Acoustic-based technologies can detect sounds of piglet crushing, and diseases in pigs can manifest in various symptoms also detectable using these technologies, which often indicate underlying health issues that require attention. Pigs experiencing discomfort due to conditions such as lameness, respiratory problems, or gastrointestinal issues may produce increased vocalizations, which can serve as early warning signs of potential health problems [36]. Various disease conditions detectable by acoustic-based techniques are listed in Table 1.
Category | Condition |
---|---|
Respiratory diseases | Coughing |
Sneezing | |
Snuffling | |
Grunting | |
Digestive diseases | Moaning |
Groaning | |
Screaming | |
Vomiting | |
Piglet crushing | Piglets squealing |
Screaming | |
Ear biting | |
Tail biting |
Common pig sounds associated with diseases include coughing, sneezing, screaming, squealing, grunting, and barking sounds, which indicate respiratory issues, distress, or discomfort in pigs [37,38]. Coughing, in particular, is the pig sound associated with respiratory diseases [2,13,39]. Cough sound analysis in pig health monitoring often indicates respiratory issues such as pneumonia, influenza, or other respiratory infections [40], and their detection is crucial for preventing growth reduction, weight loss, or mortality in pigs [41]. Moreover, abnormal vocalizations in pigs during handling, transport, or confinement can indicate stress, discomfort, fear, or anxiety [42]. Monitoring and addressing these signs are vital for ensuring animal welfare across the farming, research, and transportation sectors.
Additionally, changes in the pitch, frequency, or intensity of vocalizations may indicate pain or distress, especially in piglets subjected to crushing incidents [43]. Piglet crushing, where mother pigs or sows accidentally crush piglets while lying down or moving, is the primary cause of pre-weaning piglet mortality [44]. While the precise factors contributing to sow-inflicted piglet crushing remain unclear, potential reasons include inexperience, illness, sow behavior, and differences in body weight [45]. Crushing incidents are often identified by the sound of a squealing piglet, with screaming heard in many cases [38]. These distressing, high-pitched audible indicators reflect the intense discomfort and potential injury experienced by the piglet.
In this context, integrating acoustic monitoring technology with regular health assessments can enhance the early detection of diseases and welfare issues in pigs and piglets, facilitating timely interventions and improving overall animal well-being.
Automation serves as a tool for enhancing the welfare of pigs by detecting changes in their health conditions and behaviors [36]. As part of this automation, the accurate and automatic identification of pig sounds within farm environments is important. In precision livestock farming (PLF), microphones are used for sound-based monitoring of health and welfare-relevant animal sounds within farm facilities [11]. This technology offers a convenient means of automatically and continuously monitoring animals’ health conditions through their vocalizations. When gathering data from pig farms, it is paramount to adopt approaches that minimize the animals’ stress levels [46]. To achieve that goal, microphones can be positioned throughout the farm, such as above pens for general monitoring, near feeding areas to track feeding behaviors, and in areas of social interaction among pigs [8]. Different types of microphones used in pig farms are listed in Table 2, and typical microphone settings and placements in pig farms are shown in Fig. 2, highlighting the importance of optimized settings for effective monitoring and management. These sensors are important in continuously monitoring pig health and behavior, providing valuable insights that support the application of proactive interventions and thus the achievement of improved welfare outcomes [2]. The choice of data acquisition sensor can significantly impact the sound quality, given the potential for noise interference in farm conditions, which may ultimately compromise the detection accuracies and monitoring capabilities [17]. Different models have been developed, and familiarity with the specifications of a sound sensor or microphone is important for its correct usage in a farm setup. Various devices were previously used in studies engaged in sound data collection, including unidirectional cardioid microphones [39,47], omnidirectional electret microphones [48], digital camcorders [49], an audio–video system [50], recording pens [18], and sound sensors attached to pig body parts [51,52].
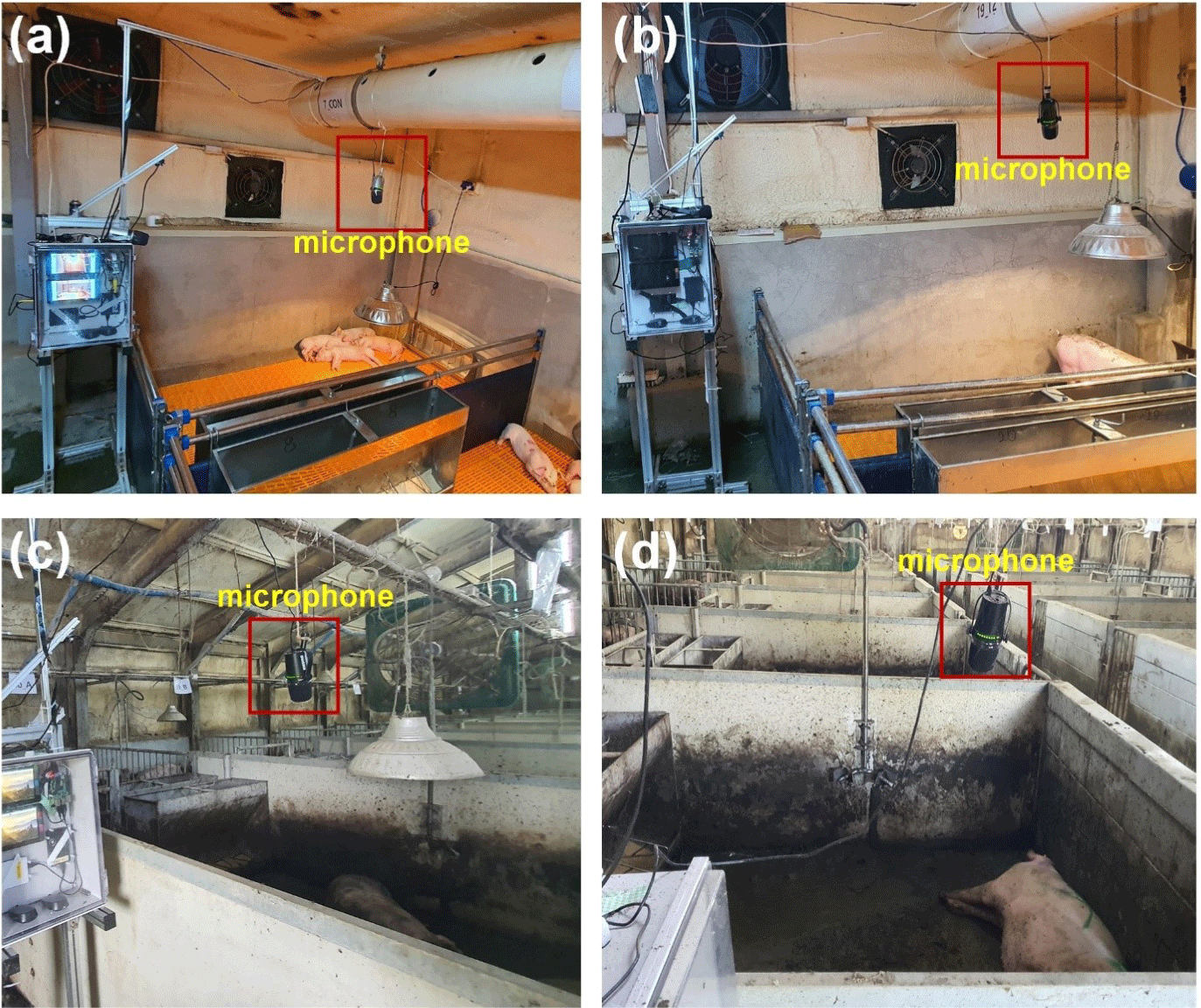
Processing and analyzing pig sounds can serve various purposes such as monitoring pig health, detecting distress, or assessing environmental conditions. There are typically four key steps in sound analysis, each contributing to a comprehensive understanding of the acoustic data [53]—sound recording, individual sounds’ labeling, sound feature extraction, and classification—as illustrated in Fig. 3. In the initial step, sound data are captured using microphones placed strategically within the pig enclosure or relevant environment. The microphone quality and placement are critical for capturing accurate and comprehensive pig vocalizations. Microphones are typically positioned around pig pens, considering factors such as height, distance from walls, and noise sources such as fans and ventilation units [53]. In various studies, a common sampling rate for microphones was 44.1 kHz [10,11,14,27,29]. However, due to experimental constraints, it is common practice for only one microphone to be used, which may harm the recording quality [28,53]. Preprocessing pig sound data is thus crucial to enhance the recording quality and extract meaningful information. Preprocessing involves filtering, pre-emphasis, framing, windowing, normalization, resampling, artifact removal, and compression. Filtering techniques such as low-pass, high-pass, and band-pass filters remove unwanted frequencies, while pre-emphasis boosts high-frequency components, compensating for attenuation during recording or transmission [33–35]. Framing divides the audio into short, overlapping segments for analysis, and windowing reduces spectral leakage with functions such as Hamming or Hann. Normalization scales the signal’s amplitude, resampling adjusts the sampling rate, and techniques such as interpolation or median filtering remove artifacts. Dynamic range compression lessens amplitude differences for a consistent loudness level, enhancing perceptual quality [49,53].
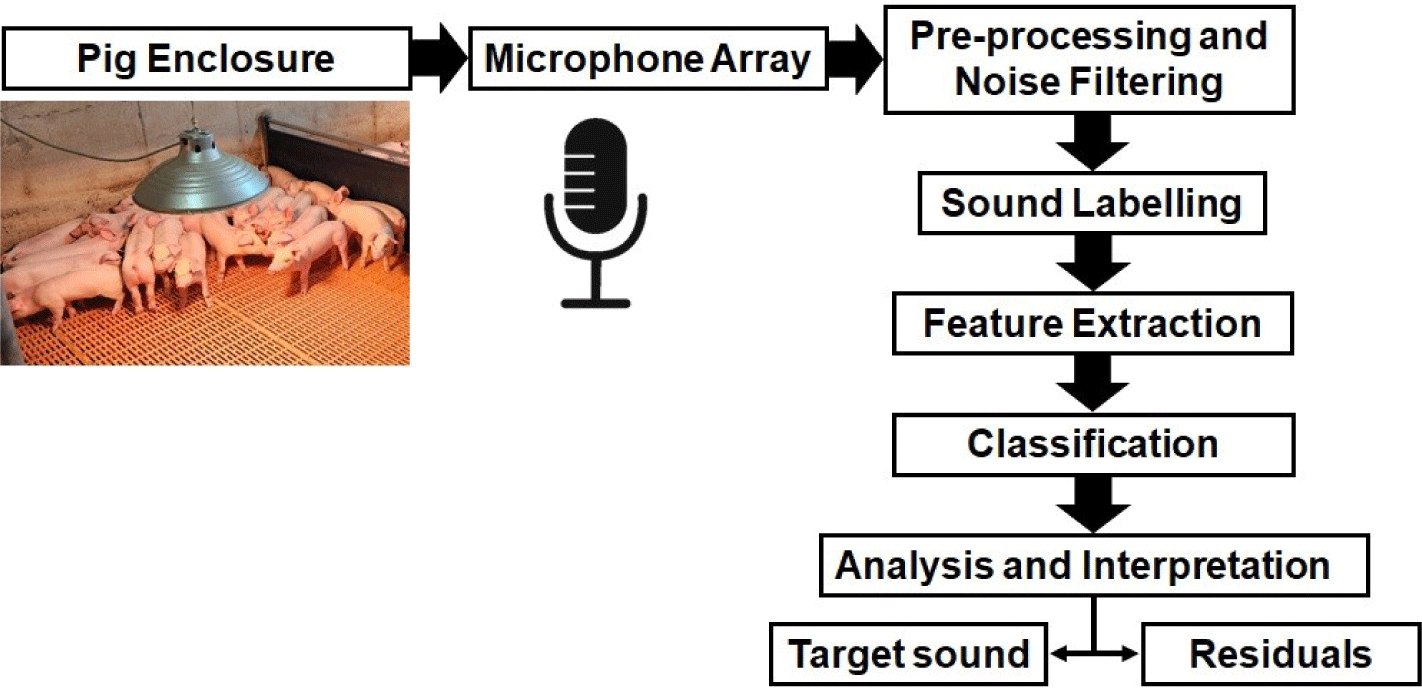
After capturing sound data, individual sound events are labeled or annotated with corresponding categories. In pig sound analysis, this means identifying and categorizing different pig vocalizations based on their acoustic features and the context, such as coughing, screaming, feeding, distress, or social interactions [11,47]. These labeled sounds provide the basis for further analysis and interpretation. Research on labeling and extracting pig sound segments is limited and often only covers manual labeling, though this requires expertise in animal research and lacks a unified standard approach [54]. Additionally, the scarcity of open-source pig sound databases presents a challenge for researchers and developers working in fields such as animal behavior studies, disease management, and even sound analysis technological development. Gaining access to diverse and comprehensive datasets will be crucial for advancing our understanding and developing applications in these domains [53,55]. Considering the diverse characteristics of pigs during their growth, it is also essential to capture information on the breeds, ages, weights, environments, and locations where sounds were produced.
Sound data undergo feature extraction to quantify their acoustic properties after labeling. Extracting features like mel-frequency cepstral coefficients (MFCCs) [56–59], spectral and temporal characteristics [47,53,58,60], and frequency-based descriptors [61,62] distinguishes different sounds and aids in classification. The mel-frequency cepstrum (MFC) represents the short-term power spectrum of a sound using a linear cosine transform of a log power spectrum on a nonlinear mel scale. The MFCC is widely favored for speech and sound recognition as it maps the linear spectrum onto a nonlinear mel spectrum of the sound signals, aligning with human hearing principles [27]. Fig. 4 shows a schematic diagram of the MFCC feature extraction process. While some studies use 13 MFCC features [63], others suggest an adaptive range of 2 to 91 [64]. Previously, the optimal results for spectral distortion (SD) distance measures were found when using a filter bank with 24 bands and a bandwidth of 220 mels [65]. Additionally, it has been noted that applying multi-taper methods in feature extraction reduces variance and enhances source separation. Thus, we may surmise from this that the number of MFCC coefficients needed depends on the specific application and the desired performance level. When analyzing audio signals, it is important to extract both temporal and spectral features in order to comprehensively understand the sound properties. Temporal features, such as energy and the zero-crossing rate (ZCR), are derived from the time domain and measure aspects such as the signal power and rate of sign changes. Spectral features, including MFCCs, gammatone cepstral coefficients (GTCCs), and linear predictive coding (LPC), are obtained by converting the time-based signal into the frequency domain using techniques such as the Fourier transform (FT) and short-time Fourier transform (STFT). Fig. 5 shows that various spectral and temporal characteristics, along with frequency-based descriptors, are essential for effective sound feature characterization and extraction. These frequency-based descriptors capture essential audio signal characteristics, facilitating robust sound feature extraction.
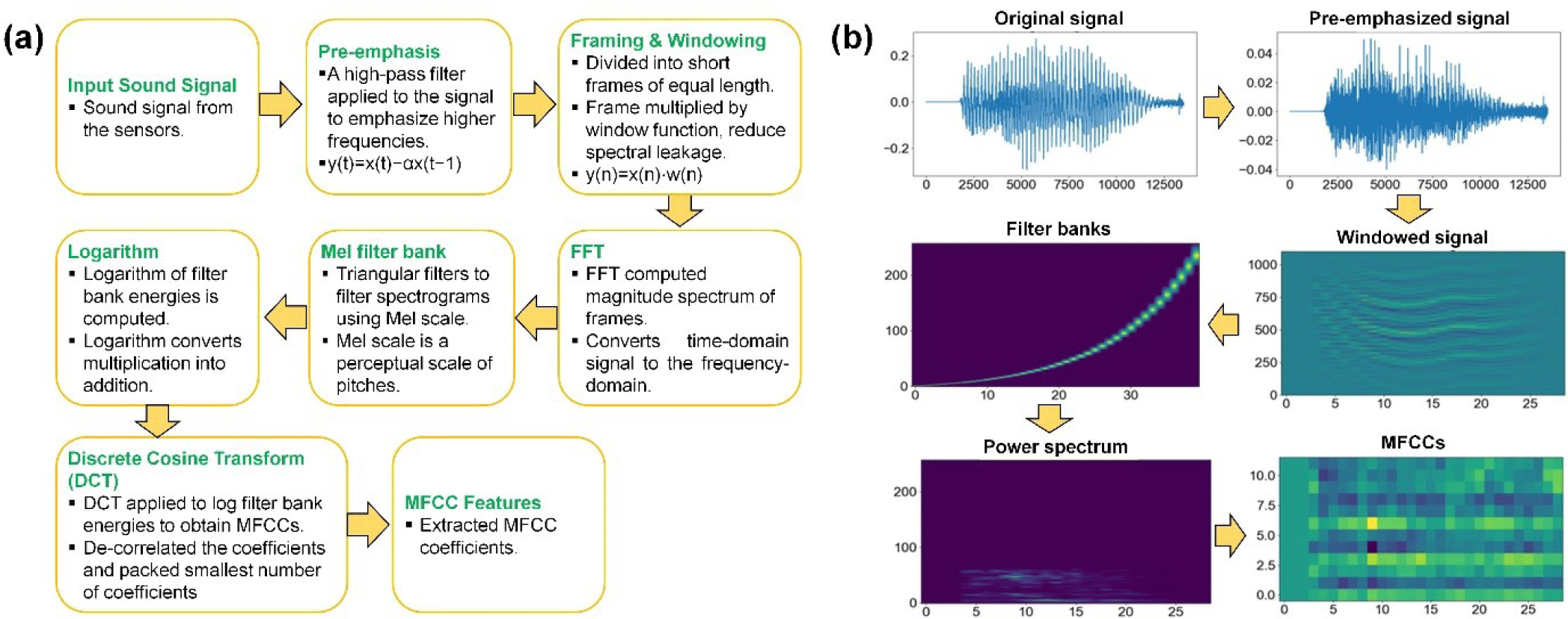
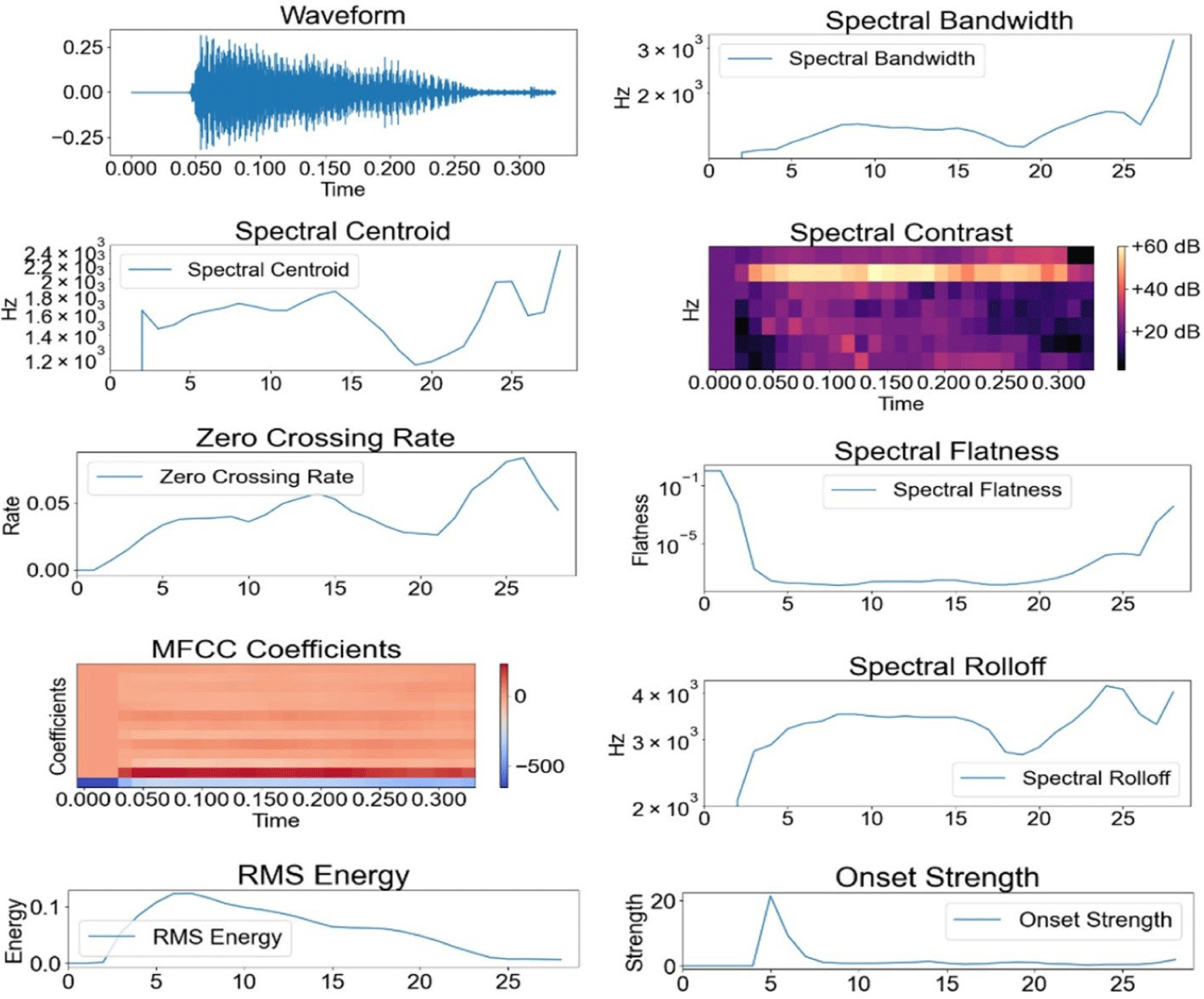
The features offer a concise yet informative representation of the sound data, facilitating a robust classification in pig sound analysis. In sound recognition, it is critical to select relevant variables in the feature extraction phase. This step involves combining the key variables that effectively represent the sound signal into a multidimensional feature vector [17]. In field conditions, background noises are abundant and inevitable; these can lower the quality of sounds in recordings and affect model recognition performance [17]. To improve model performance, sound data preprocessing techniques such as background noise filtering are used [27,47,49]. Additionally, the model may be trained with background noise data, and advanced feature extraction methods may be applied, such as the dominant neighborhood structure (DNS) algorithm; techniques such as these may lead to a superior performance in noisy environments compared to traditional methods such as MFCCs [25,26,49].
In classification, algorithms categorize sound instances into predefined classes using extracted features. Techniques such as support vector machines (SVMs) [13,57,66,67], random forests (RFs) [26,68], and convolutional neural networks (CNNs) [39,47,57,69,70] are commonly employed for this task. Learning from labeled sound data enables these algorithms to discern between various pig vocalizations and thus offer insights into pig behavior and health and environmental conditions. The continuous process of sound analysis is refined at each stage to enhance its accuracy and efficiency and the outputs’ interpretability [16,69]. However, the limitations in pig sound data processing include a dependency on manual labeling, lack of standardization, sparse open databases, limited feature extraction techniques, and variability in algorithms’ performances [58]. Future directions for development could involve automated labeling solutions, standardization initiatives, open databases’ expansion, advanced feature extraction techniques, and contextual information’s integration into classification algorithms to enhance their robustness and adaptability.
Several factors influence acoustic data on pig farms, including environmental conditions such as temperature and humidity; the farm layout and infrastructural materials; pig behavior, such as feeding and social interactions; the health and stress levels of the pigs, including disease conditions; piglet crushing by mother pigs or in crowded farm conditions; equipment noise from machinery; and human activity [2,71,72]. Background noise can interfere with the clarity of acoustic data and may require noise-canceling techniques or the careful placement of microphones to minimize its impact [36]. Fans and ventilation systems also cause major background noise, which hinders accurate pig sound data collection as it may mask pig vocalizations, lower the signal-to-noise ratio, and reduce the clarity of the audio [16,73], making it challenging to distinguish and analyze specific pig vocalizations.
Pig behavior also significantly influences the quality of acoustic data collected on pig farms. Vocalizations vary in type and intensity, with activity-based calls like feeding, fighting, and mating being louder and more distinct [2,74]. This can skew data toward specific behaviors if recordings capture mostly active periods. For instance, pigs have shown peak noise levels during feeding, with loud vocalizations while waiting for the food [75]. Furthermore, aggressive behavior such as pushing occurs around feeding areas. After feeding, noise levels then drop as pigs rest [53,76]. Additionally, piglets have quieter vocalizations compared to adults, posing challenges in detecting them in noisy environments [77]. Moreover, stress and discomfort can alter pig vocalizations, leading to data that may not represent the pigs’ usual state, rendering the data difficult to interpret [78]. Moreover, movements and interactions among pigs can generate additional noise, potentially obscuring the desired vocalizations in recordings [53,55]. Moreover, if pigs are particularly active or vocal during certain times of the day, it may be challenging to isolate specific acoustic signals of interest at those times.
The types and quality of sensors used for data acquisition also play a significant role. High-quality microphones capable of capturing a wide range of frequencies with minimal distortion are essential for accurate acoustic data collection [2,17,49], as is the placement of microphones [17], which should be strategically positioned to capture relevant sounds while minimizing interference from background noise and other sources [17,53]. Moreover, environmental factors such as temperature, humidity, and airflow can affect the propagation of sound waves within the pig farm [79,80], and these conditions may thus impact the accuracy and reliability of acoustic data acquisition systems.
Lastly, acoustic data acquisition systems on pig farms may be susceptible to interference from external sources such as nearby roads, industrial activities, or neighboring farms. Shielding or filtering techniques may be required to minimize this interference. Ensuring a reliable power supply and robust connectivity for data transmission are also essential for continuous monitoring and recording of acoustic data on pig farms. Addressing these factors through appropriate equipment selection, installation, and data-processing techniques can help optimize acoustic data acquisition on pig farms, for improved monitoring and management practices. These variables collectively shape the acoustic environment on the farm and can thus affect the quality and interpretation of sound data collected for various purposes, such as behavior monitoring and health assessment. Understanding and accounting for these factors are crucial for accurate analysis and effective management practices in pig farming.
APPLICATION OF SOUND-BASED TECHNOLOGIES FOR PIG HEALTH MANAGEMENT
Classification and detection of pig vocalizations involve analyzing and interpreting the sounds pigs make to assess their health, behavior, and welfare. This process utilizes advanced acoustic-based technologies and machine learning techniques to distinguish between various vocalizations, such as distress calls, contentment sounds, or social interactions. Hou et al. [16] improved pig vocalization classification using a multi-feature fusion method, enhancing features with principal component analysis (PCA). Features such as short-time energy, frequency centroid, formant frequency, and MFCCs were extracted, and then they were enhanced with PCA. Their approach employed a back propagation (BP) neural network optimized with a genetic algorithm (GA), which achieved high accuracy (93.2%), precision (92.9%), and recall (92.8%), proving effective for automatic recognition and feedback of pig vocalizations. Wang et al. [21] proposed a novel algorithm for recognizing sow estrus sounds using an improved MobileNetV3 lightweight CNN. Sound data were collected from 63 Canadian sows, denoised using fast Fourier transform (FFT), and underwent feature extraction using log-mel spectrograms (LM). The model achieved 97.12% precision, 97.34% recall, a 97.59% F1-score, and 97.52% accuracy. Compared to traditional methods, this approach more accurately captures the vocal characteristics of sows in latent estrus. However, certain issues remain. For instance, classification accuracy may drop when vocal signals have a low signal-to-noise ratio, which can be mitigated by using better microphones, advanced recording techniques, and more data. Additionally, overlapping grunts in low-frequency estrus sounds can lead to misclassification.
A low-cost, real-time sound-based pig abnormality monitoring system was proposed [22] for installation in real pigpens. The system uses an adaptive context attachment model (ACAM)-based noise-robust voice activity detection (VAD) algorithm to detect sound regions in noisy environments. Each detected sound region is converted into a spectrogram, and the CNN-based MnasNet structure generates sound features and classifies them to detect anomalies. The system achieved an F1-score of 0.947 in identifying abnormalities, even in noisy pigpens. The execution time was 0.253 seconds, which was 0.220 seconds faster than the basic MnasNet model. Wang et al. [26] proposed a VAD method to automatically segment continuous sound. Individual sounds were segmented and acoustic features extracted, including MFCC and power spectral density (PSD). Deep features were derived from spectrograms, mel spectrograms, constant-Q transforms (CQTs), and MFCC color matrix maps using the lightweight SqueezeNet network. Multiple classification models were obtained when feeding acoustic and deep features into different classifiers, including SVM, adaptive boosting (AdaBoost), and bidirectional long short-term memory (BiLSTM). The experimental results showed recall and precision rates of 93.1% and 91.6%, respectively, when detecting pig coughs in continuous sound, while the recognition accuracy for continuous pig coughs reached 91.4%. This method represents a significant advancement in pig health monitoring as it can operate autonomously in actual farm conditions, whereas previous studies were often limited to controlled laboratory environments.
Song et al. [27] presented an improved DenseNet-based model for pig cough sound recognition, enhanced with Squeeze-and-Excitation Network (SENet) attention modules. The developed SE-DenseNet-121 model was used to improve the accuracy of pig cough detection, which is crucial for providing early warnings of respiratory diseases in swine. Their study explored various combinations of MFCC features, finding that 26-dimensional MFCC + ΔMFCC provided the optimal performance. The resulting SE-DenseNet-121 model achieved an accuracy of 93.8%, recall of 98.6%, precision of 97%, and an F1-score of 97.8% for pig cough sound recognition. This improved model demonstrates significant potential for use in developing accurate and efficient pig cough sound recognition systems, which could be invaluable for the early detection of respiratory issues in pig farming. Ji et al. [81] proposed a novel feature fusion method combining acoustic and visual features to improve pig cough recognition accuracy. The acoustic features were extracted using root-mean-square energy (RMSE), MFCCs, ZCRs, and spectral characteristics from audio signals. Visual features, meanwhile, were extracted from CQT spectrograms using local binary patterns and a histogram of gradients. The acoustic and visual features were then combined into a hybrid feature set using the Pearson correlation coefficient (PCC), recursive feature elimination (RFE) with RF, and PCA. The fused feature set was evaluated using SVM, RF, and k-nearest neighbor (KNN) classifiers. The results showed that the combined acoustic and visual features achieved 96.45% accuracy in pig cough classification. This approach demonstrates the potential for multimodal feature fusion to enhance the precision of pig cough detection systems in providing early warnings of respiratory diseases.
Choi et al. [49] developed a noise-robust system for sound-event classification using texture analysis. One-dimensional sound signals were transformed into two-dimensional gray-level images through normalization, and the researchers applied the DNS technique to extract textural features. Experimental validation employed four classifiers: CNN, SVM, KNN, and C4.5. The system achieved an F1-score exceeding 96.57% on livestock data, demonstrating its superior performance in noisy conditions compared to other methods. Liao et al. [69] proposed TransformerCNN, a sound classification model combining CNN spatial feature representation with Transformer sequence coding. Eight features were used for this experiment: LM, MFCC, chroma, spectral contrast, tonnetz (CST), MFCC + CST (MC), LM + CST (LMC), and MC + LMC (MLMC). Through comprehensive qualitative and quantitative evaluations, the approach demonstrated a high performance level in classifying domestic pig sounds, with an accuracy of 96.05%. The model also exhibited robustness and generalization across different input features, for which it showed a consistent performance. Table 3 summarizes the different methods and algorithms applied in pig vocal and cough recognition, classification, and real-time detection.
Detection/classification | Classification technique | Feature Extraction technique | Accuracy | Reference |
---|---|---|---|---|
Vocal classification | BP + GA | Short-time energy, Frequency centroid, Formant frequency, MFCC | 93.20 | [16] |
CNN-MobileNet V3 | Fast Fourier transform (FFT), Log-mel spectrogram | 97.52 | [21] | |
MnasNet | ACAM, VAD | 94.72 | [22] | |
SVM, AdaBoost, BiLSTM | MFCC, PSD, CQT, SqueezeNet | 91.41 | [26] | |
SE-DenseNet-121 | MFCC, ΔMFCC, Δ2MFCC | 93.80 | [27] | |
SVM | RMSE, MFCC, ZCR, Centroid, Flatness, Bandwidth, Chroma | 96.45 | [81] | |
CNN, SVM, KNN | DNS | 96.57 | [49] | |
TransformerCNN | MLMC | 96.05 | [69] |
BP, back propagation; GA, genetic algorithm; MFCC, mel-frequency cepstral coefficients; CNN, convolutional neural networks; ACAM, adaptive context attachment model; VAD, voice activity detection; SVM, support vector machines; PSD, power spectral density; CQT, constant-Q transform; BiLSTM, bidirectional long short-term memory; SE, Squeeze-and-Excitation; RMSE, root-mean-square energy; ZCR, zero-crossing rates; KNN, k-nearest neighbor; DNS, dominant neighborhood structure.
Early detection of pig diseases using sound is an innovative and effective approach that has shown promising results in recent years. This method primarily focuses on analyzing pig vocalizations, especially coughs, to identify respiratory diseases before they become severe. Gutierrez et al. [14] aimed to classify porcine wasting diseases by analyzing cough sounds from pigs infected with porcine circovirus type 2 (PCV2), porcine reproductive and respiratory syndrome (PRRS) virus, and Mycoplasma hyopneumoniae (MH) and comparing those with normal coughs. Thirty-six pigs were studied, in which blood samples confirmed their infections. Cough sounds were recorded for 30 minutes using a video recorder, the recording was inputted into commercial software, and the cough sounds were labeled and analyzed using ANOVA and discriminant analysis. The results showed that normal coughs had a higher pitch compared to infectious coughs (p < 0.002). Chung et al. [13] analyzed coughing sounds for their frequency, intensity, and other characteristics in an effort to detect pig-wasting disease. Cough sounds from infected pigs were individually recorded and digitalized and then compared to normal pig sounds, which were recorded without other noise and labeled using auditory processing. The study found that a combination of MFCC and support vector data description (SVDD) could automatically detect pig-wasting diseases using cough sounds, with 94% accuracy.
Zhao et al. [18] introduced a novel method using a deep neural network (DNN)-hidden Markov model (HMM) for continuous pig cough sound recognition to detect respiratory diseases early. The process includes noise elimination with the Wiener algorithm based on wavelet thresholding, followed by feature extraction using a 39-dimensional MFCC. The DNN-HMM model categorizes farm sounds into pig coughs, non-pig coughs, and silence and achieves a 7.54% word error rate (WER). Yin et al. [39] developed a method for recognizing sick pig cough sounds using CNNs. The fine-tuned AlexNet model with spectrogram features was utilized for its image recognition capabilities. Spectrograms were constructed using the STFT and initially saved as 640 × 480-pixel images. These images were resized to 227 × 227 pixels, the optimal input size for AlexNet. The resized spectrograms were then inputted into the fine-tuned AlexNet model for classification, and the results showed that the proposed algorithm achieved a cough recognition accuracy of 96.8%, overall recognition accuracy of 95.4%, and F1-score of 96.2%. To reach these results, the study used preprocessing and data augmentation to tackle the complex acoustic environments in pig houses. This approach shows promise for the development of intelligent alarm systems for the early detection of respiratory diseases in pigs, thereby enhancing animal welfare and farm management.
Shen et al. [47] investigated a novel framework that combines acoustic features with deep learning features to enhance the accuracy of pig cough sound recognition. The proposed method integrated traditional acoustic features, such as MFCCs, time-frequency representations (TFRs) involving the CQT, and STFT, with deep features extracted using CNNs. The combined features were fed into a SVM using early fusion to identify pig cough sounds. This fusion approach combined the strengths of both feature types to improve the robustness and precision of cough detection in noisy farm environments. The CQT is more suitable for sound recognition in a pig housing environment than the traditional linear STFT. The study achieved a high recognition accuracy of 97.35%, demonstrating the effectiveness of the combined feature approach. The results suggest that this method can significantly enhance the early detection of respiratory diseases in pigs, providing a valuable tool for improving animal health management and welfare in commercial pig farming.
Shen et al. [57] introduced a novel method for improving pig cough sound recognition accuracy by combining MFCC-CNN features. These features were created by fusing multiple frames of MFCCs with single-layer CNNs. Classification utilized softmax and linear SVM classifiers, validated through field experiments. The results showed a significant performance enhancement with the MFCC-CNN features compared to MFCCs. The F1-scores were improved to 10.37% and 5.21%, and the cough detection accuracy improved by 7.21% and 3.86% for the softmax and SVM classifiers, respectively. Elsewhere, a novel approach was presented [73] for pig sound recognition using a combination of DNNs and HMMs. Audio samples were collected from 10 landrace pigs in various states, including eating, estrus, howling, humming, and panting. Audio data were preprocessed using Kalman filtering and an improved endpoint detection algorithm based on the empirical mode decomposition–Teager energy operator (EMD-TEO) cepstral distance. The system extracts 39-dimensional MFCCs as features for network learning and recognition. The DNN-HMM model, with five HMM states and three DNN hidden layers of 128 nodes each, achieved a high performance in recognizing different pig sounds, with recall rates of 73.7%–100%, an accuracy of 70%–95%, and a specificity of 92.6%–98.8%. Compared to the traditional Gaussian mixture model (GMM)-HMM, the DNN-HMM approach showed significant improvements in the average recall rate (12.42% higher), accuracy (17% higher), and specificity (4.14% higher). The study demonstrated the effectiveness of this hybrid deep learning approach for accurate pig sound recognition, which has potential applications in the automated monitoring of pig health and behavior in farm settings.
Chen et al. [70] introduced a novel method for estrus sound recognition using the fusion of two representative features and CNNs. The study extracted features using MFCCs and Chirplet MCCs (CMCCs), combining these features as inputs to 1D-CNN models. The approach improved sow estrus prediction accuracy in real farm conditions, achieving a high test performance with an accuracy score of 0.96. Table 4 summarizes the different methods and algorithms for pig disease detection and monitoring using sound on a pig farm.
Detection/Classification | Classification Technique | Feature Extraction Technique | Accuracy | Reference |
---|---|---|---|---|
Sound | ANOVA | Digitalized | - | [14] |
SVDD | MFCC | 94.0 | [13] | |
DNN-HMM | MFCC | 92.46 | [18] | |
Fine-tuned AlexNet | Spectrogram with STFT | 95.4 | [39] | |
SVM | MFCC, TFR, CQT, STFT, CNN | 97.35 | [47] | |
SVM, Softmax | MFCC–CNN | 96.68 | [57] | |
DNN-HMM | Kalman filtering, EMD-TEO, MFCC | 83.0 | [73] | |
CMCC-CNN | MFCC | 96 | [70] |
MFCC, mel-frequency cepstral coefficient; SVDD, support vector data description; DNN-HMM, deep neural network–hidden Markov model; STFT, short-time Fourier transform; SVM, support vector machine; STFT, short-time Fourier transform; TFR, time-frequency representation; CQT, constant-Q transform; CNN, convolutional neural network; EMD, empirical mode decomposition; CMCC, Chirplet mel-frequency cepstral coefficient.
Ferrari et al. [25] explored the use of pig vocalizations to evaluate heat stress in swine. The study aimed to enhance our understanding of animal welfare by analyzing how pigs respond vocally to stressful conditions, particularly heat stress. The researchers found they could distinguish between different stressors in pigs, such as pain and heat, with an accuracy of 81.12%. The methodology involves using sound analysis techniques to monitor and interpret pig vocalizations, which can serve as indicators of their thermal comfort and overall well-being. This approach offers a non-invasive and efficient means of assessing heat stress in swine, potentially leading to better management practices in livestock farming and thus supporting animal welfare and productivity. Traditional methods often rely on manual inspections or reactive adjustment, while sound-based monitoring enables continuous, non-invasive observations for faster issue detection and precise interventions, which reduce resource wastage and thus promote more sustainable agricultural practices [25]. Vandermeulen et al. [38] identified specific sound features of pig screams that indicate stress, aiding in animal health and welfare monitoring. After analyzing seven hours of labeled data from 24 pigs, the authors used the sound features to develop a detection method with physical meaning and explicit rules. They transformed the sound data using FFT, chirp group delay (CGD), and fundamental frequency calculation (FFC) to obtain frequency information. Pig screams were found to have a distinct formant structure, adequate power, high frequency content, and sufficient variability and duration. The detection method achieved 72% sensitivity, 91% specificity, and 83% precision. Its application for the continuous monitoring of pig vocalizations on farms may help farmers detect health or welfare issues early and thus improve their animal care practices.
Moura et al. [46] developed software for the real-time monitoring and analysis of piglet vocalizations to assess their stress levels. The researchers created a system that can detect and analyze distinct sounds from piglets and correlate those with stress levels. The software uses a combination of sound analysis techniques, including linear prediction coding, and artificial neural networks to identify stress vocalizations in commercial piggery environments. The system interprets piglet sounds and classifies different stress conditions by focusing on specific acoustic parameters such as signal duration, resonance frequencies, and amplitude. The research demonstrated that vocalization analysis offers an efficient, non-invasive method for identifying stress in piglets, potentially allowing for real-time welfare assessment in commercial pig farming. This approach could provide farmers with a valuable tool for detecting stress-related issues early, thus enabling timely interventions to improve animal welfare and productivity. da Silva et al. [78] developed software predicting stress in piglets based on their vocal calls during various stressful conditions (cold/heat, pain, hunger, thirst). Vocal signal intensities were analyzed from 40 piglets under stress conditions, with unstressed piglets serving as a baseline. Data organization and paraconsistent logic were used to handle uncertainties arising from overlapping vocal signal intensities. The results showed a high accuracy in predicting pain-induced stress (93.0%), but lesser accuracy in predicting stress-free conditions (normal). Nonetheless, the method effectively resolved uncertainties in overlapping signals, with particular success in distinguishing pain-related vocalizations due to their higher intensity and longer duration.
Early identification of tail biting is crucial for animal health and welfare as it allows for early intervention. Heseker et al. [82] detected pig screams in audio recordings with the goal of identifying tail biters among 288 undocked weaner pigs in six pens using a binomial, generalized, linear mixed-effects model (GLMM) and linear mixed-effects (LME) model. When a biter was visually identified and removed, the previous days’ recordings were analyzed for screams (sudden loud noises above 1 kHz) and tail-biting events. It was found that 52.9% of the 2,893 detected screams were due to tail biting in the pen. Audio analysis identified biters 1–9 days before they were visually detected. The corresponding screams could be detected earlier than physical signs, which suggests that vocalization analysis may provide an effective early warning system for tail biting. Cordeiro et al. [43] investigated the use of vocalization signals to estimate pain levels in piglets during common farm management procedures. The researchers recorded vocalizations from 20 male piglets under four conditions: normal circumstances (pain-free), marking with the Australian method, tail trimming, and castration. Analysis of the sound signals revealed that the vocalizations’ pitch frequency (Hz), maximum amplitude (Pa), and intensity (dB) increased progressively from pain-free pigs to those undergoing marking and further increased for tail trimming and castration procedures. There was no significant difference in vocal responses between tail trimming and castration, suggesting similar pain levels for these procedures. The study demonstrated that specific acoustic parameters of piglet vocalizations, such as pitch, amplitude, and intensity, can offer reliable indicators of pain levels. This research contributes to the development of non-invasive methods for assessing animal welfare in pig farming, which may allow for more timely interventions and improved management practices to reduce pain and distress in piglets.
Chapel et al. [44] compared vocalization patterns between piglets crushed by sows and those manually restrained by humans. The authors recorded vocalizations from 10 sows and their litters 48 hours after parturition, collecting 631 calls from crushed piglets and 659 calls from restrained piglets. Analysis of the acoustic properties revealed significant differences between the two groups. Crushed piglets exhibited lower fundamental frequencies (523.57 Hz vs. 1,214.86 Hz) and narrower bandwidths (4,897.01 Hz vs. 6,674.99 Hz) in the loudest portion of their calls compared to restrained piglets. Additionally, crushed piglets had a lower mean peak frequency overall (1,497.08 Hz vs. 2,566.12 Hz). These findings highlighted important distinctions in vocalization patterns between piglets experiencing crushing events and those undergoing human restraint. The study suggested that future research should measure sow reactivity to these different types of vocalizations, to improve research practices and potentially develop more effective methods for reducing piglet crushing in swine production. Illmann et al. [77] investigated piglet distress vocalizations under simulated crushing and isolation conditions, exploring variations with age, body weight, and health status. The researchers observed that piglets squeezed on day 1 emitted more intense distress calls than those on day 7, and that lighter piglets vocalized more during squeezing than heavier ones, while their health status did not significantly affect the vocalization intensity during squeezing. Furthermore, neither age nor weight influenced the vocalization intensity in isolation; instead, these factors had a combined effect. The findings as such indicate that vocalizations reflect piglets’ vulnerability and need for maternal care, particularly in scenarios resembling crushing by the sow.
Wang et al. [80] investigated the relationship between animal cough sounds and environmental air quality. They analyzed cough sounds from 84 weaners alongside four key air quality factors: air temperature, relative humidity, ammonia concentration, and dust concentration. Their study found significant differences in cough sounds among weaners exposed to varying air qualities, as indicated by PSD analysis. The developed recognition algorithm, utilizing principal mel-frequency cepstrum coefficients (PMFCCs), PCA, and SVM, achieved an impressive average recognition rate of 95% for sound samples collected across different pig houses. These findings suggest that cough sound analysis can provide qualitative insights into air quality conditions within commercial livestock buildings.
Sistkova et al. [75] examined how the time of day and season affect noise levels from pigs in pig housing with slatted floors. It was observed that pigs were louder during feeding times, when there was increased squealing. Seasonal variations also impacted noise levels, with higher noise typically noted in summer compared to winter. These findings highlight the importance of managing noise to raise pig welfare during farm operations, as excessive noise can cause stress for animals and workers alike. The authors suggested that implementing strategies to mitigate noise may enhance the acoustic environment in pig barns and thus improve overall farm management practices. Table 5 summarizes the different models and algorithms developed to recognize pig behavior and activity, identify tail biting and piglet crushing, and monitor farm environmental conditions using sound data.
Detection/Classification | Classification technique | Feature Extraction Technique | Accuracy | Reference |
---|---|---|---|---|
Behavior/activity | Power spectrum density | Average peak frequency, fundamental frequency, duration | 81.12 | [25] |
Rule-based classifier | FFT, CGD, FFC | 87.00 | [38] | |
Polynomial adjustment | FT | - | [46] | |
Decision tree | Acoustic response | 93.0 | [78] | |
Tail biting/piglet crushing | GLMM+LME | Pitch frequency | 52.9 | [82] |
Vocalization pattern | Pitch frequency, maximum amplitude, intensity | 78.20 | [43] | |
Mean maximum frequency | Acoustic properties | 25-75 | [44] | |
Vocalizations pattern | Intensity | - | [77] | |
Environmental factors | PCA + SVM | PMFCCs | 95.00 | [80] |
ANOVA | Noise level | - | [75] |
FFT, fast Fourier transform; CGD, chirp group delay; FFC, fundamental frequency calculation; FT, Fourier transform; GLMM, generalized, linear mixed-effects model; LME, linear mixed-effects; PCA, principal component analysis; SVM, support vector machine; PMFCC, principal mel-frequency cepstrum coefficient.
Wearable sensors are less developed for applications in pigs than in cattle. Of the studies to date, Yoshioka et al. [83] proposed a recording system to detect respiratory diseases in pigs using body-conducted sound (BCS). This system can capture biological signals in groups, such as the individuals’ heartbeats. A piezoelectric sensor extracts and records these sounds via frequency modulation (FM) waves. In that way, this system records individual pigs’ sounds from a distance. An experiment was performed in which BCSs were recorded before and after PRRSV inoculation on days 3, 5, 7, and 10. Acoustic analysis showed significant differences in the ZCR and MFCC before and after inoculation, suggesting that respiratory diseases could be detected early through acoustic features. The authors’ concept of wearable-sensor-based disease detection using wireless data acquisition is shown in Fig. 6.
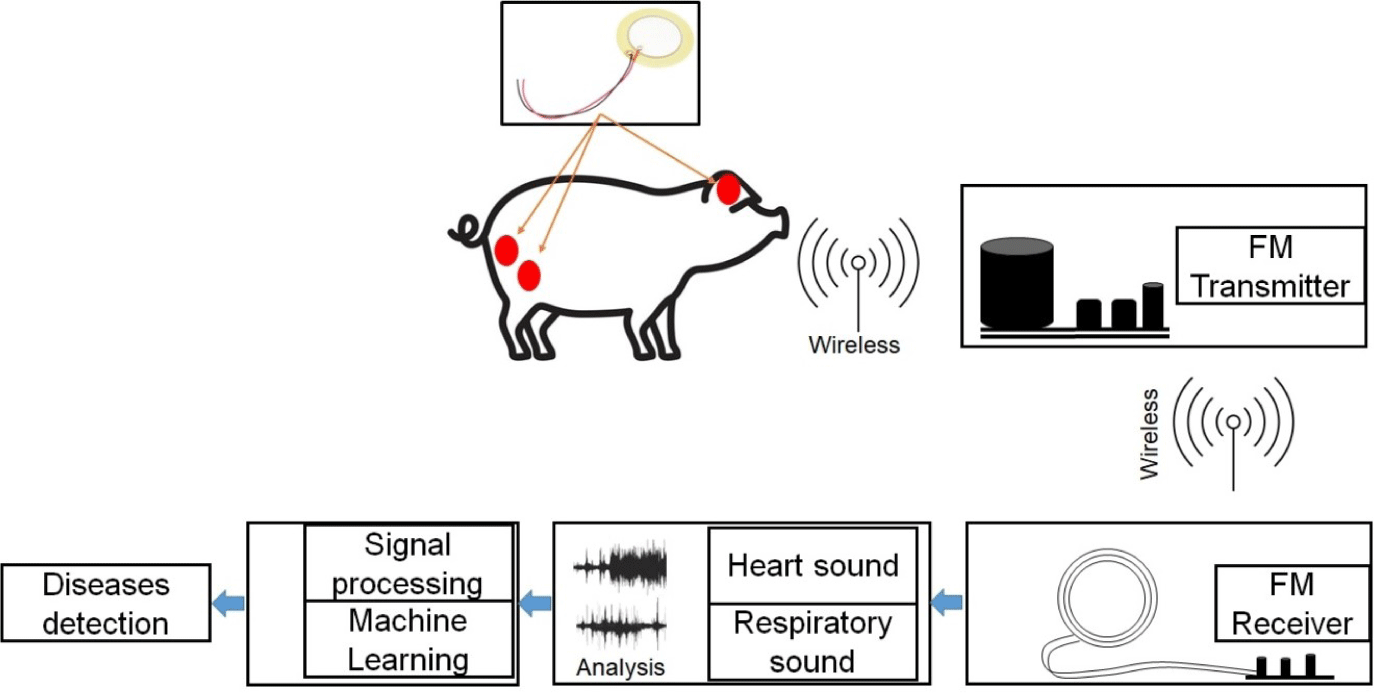
Cheng et al. [84] developed a wireless system to collect BCS with a piezoelectric sensor on pigs’ skin and respiratory sounds using a small, high-quality MEMS microphone, which does not burden the pig. Recordings are made using a Cymatic Audio LR-16 recorder. In their experiment, the data were converted into a waveform, and strong peaks were detected. Cheng et al. [85] later established an early detection system for respiratory diseases in pigs using BCS. A wireless recording system for the ear tip was developed to capture respiratory sounds and heartbeats. In pigs with PRRS, significant differences in ZCR and MFCCs were found before and after virus inoculation. These acoustic features suggest the recording system’s potential for use in the early detection of respiratory diseases. In a similar study, Tsuchiya et al. [86] proposed an early detection system for respiratory diseases in pigs using BCS. The system monitors respiratory sounds and heartbeats and extracts periodic components in BCS using independent component analysis (ICA) and adaptive signal processing (ALE). In the authors’ experiment, significant differences in the ZCR and MFCC features were found before and after inoculation, suggesting that respiratory diseases can be detected early by analyzing such acoustic features.
Wearable sensors in pigs hold potential for disease detection and monitoring, which may help prevent the spread of illnesses and support effective outbreak management, though they currently come with notable drawbacks. Their potential arises through the scope for continuously monitoring physiological signals such as heartbeats, respiratory patterns, and BCSs in a non-invasive mode that does not disturb the pigs, thereby producing data with greater accuracy than those generated through alternative means, which may be utilized to improve animal welfare, while saving time and labor for farmers through the automation of data collection and analysis.
However, the significant drawbacks of wearable sensors at present include the high costs for their development, installation, and maintenance, which can be prohibitive for many farms; the complexity of data interpretation, requiring advanced algorithms and skilled personnel, which can be resource-intensive; the risk of sensor malfunction or data loss due to technical issues, potentially affecting system reliability; the potential for animal discomfort from sensors; and the influence of environmental factors such as humidity and dirt on sensor performance. Despite these issues to be overcome, the advantages that wearable sensors offer in early disease detection and enhanced animal welfare make them a promising tool in livestock management.
SoundTalks (SoundTalks NV, Leuven, Belgium) is a pioneering technology that has garnered significant attention for its capabilities in automatic respiratory disease detection using cutting-edge artificial intelligence (AI) algorithms. SoundTalks is an AI-powered technology that autonomously monitors the respiratory health of pigs from the nursery to their slaughter by recognizing and quantifying cough sounds. Sensors track environmental conditions such as room temperature and humidity, and an alert system notifies the farmer about potential respiratory issues and sudden temperature fluctuations. SoundTalks has two main components: a monitor and a gateway, as shown in Fig. 7A. The device features a microphone and environmental sensors fixed to the monitor, along with LED lights for alert indication. It collects sound data within a 10-meter radius and wirelessly transmits all data to the gateway, which can receive data from multiple monitors within a 30-meter range and which then sends the data to the AI cloud for processing and analysis. Farmers can conveniently access the data online via a PC or smartphone app, provided they have a strong and uninterrupted internet connection.
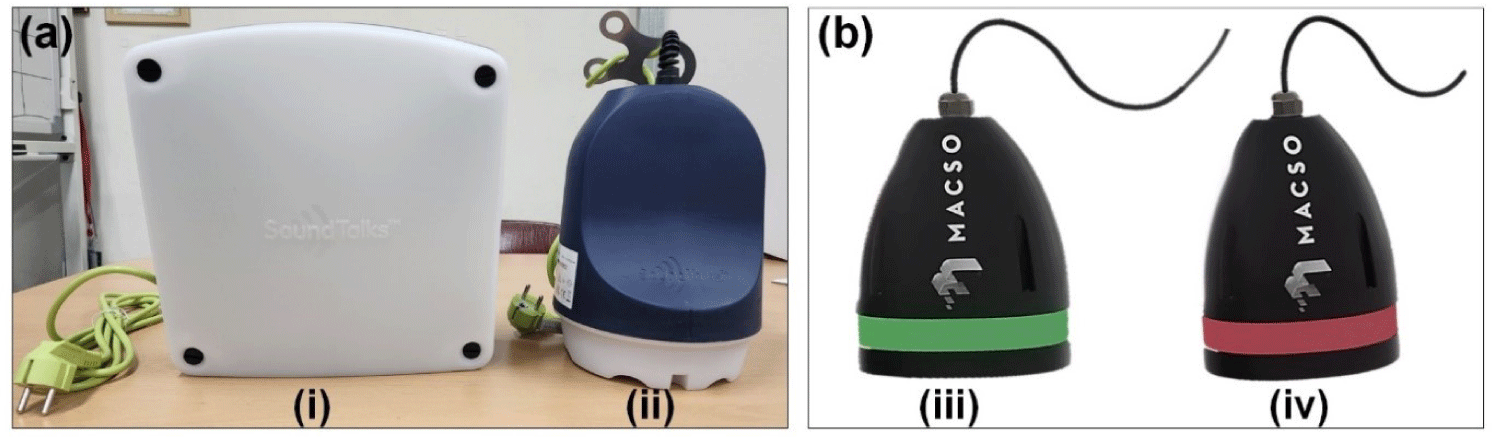
MASCO (MACSO Technologies Limited, CA, USA) has introduced an innovative solution “AgTech” for detecting pig sounds and using AI algorithms to identify sick pigs within farm settings. This cutting-edge system offers easy installation and boasts a high accuracy and enables continuous respiratory health monitoring, significantly enhancing efficiency and addressing challenges posed by skilled labor shortages. The advanced AI platform powered by MASCO provides farmers with a 24/7 audio-monitoring solution, which means respiratory health can be monitored even when farmers are off-site. Each audio sensor is trained to recognize early signs of respiratory illness, as shown in Fig. 7B. The system promptly alerts farmers when such signs are detected, facilitating timely interventions.
The solution also offers access to historical farm data via an intuitive dashboard, empowering farmers with valuable insights that support their informed decision-making. MASCO has demonstrated proficiency in accurately detecting distinct pig sounds through “AgTech”, and the firm has expressed an ongoing commitment to revolutionizing farm management practices with state-of-the-art technology.
Fancom (Fancom BV, Panningen, The Netherlands) introduced the Pig Cough Monitor (PCM), which monitors pig respiratory health through an innovative automated design. The PCM continuously analyzes sounds recorded within the pig house and enables the real-time observation of coughing events, facilitating the early detection of diseases and minimizing the need for antibiotics. This PCM system comprises a control unit equipped with analysis software and two strategically placed microphones. By consolidating the data streams from the two microphones, the on-farm solution provides a comprehensive overview of farm operations. The system filters recorded sounds to differentiate between general pig noises and coughing episodes. Graphical representations of these data are provided to end-users through the F-Central FarmManager software, with alarms triggered when preset thresholds are surpassed. Farmers can then assess coughing levels based on established norms to determine whether they are acceptable, enabling better decision-making and enhanced efficiency. The opportunity provided to intervene when unacceptable coughing erupts supports efforts to ensure an enhanced animal performance, consistent growth, and increased profitability. Moreover, the software is designed to streamline farm management, ensuring that all critical information is easily accessible and actionable for optimal farm performance.
CHALLENGES AND FUTURE PERSPECTIVES
Closely monitoring each pig being farmed can provide information on even minor abnormalities early, allowing for early disease prevention. In this space, automated sound-based approaches are being investigated for their potential to provide more accurate results than traditional visual inspections, addressing issues with observational subjectivity [87,88]. However, despite the advancements, sound-based precision technologies in pig farming still face several challenges. A primary issue is noise interference, as farming environments are inherently noisy due to machinery, human activity, and other animals [71-—73], which make it difficult to isolate relevant sounds. Furthermore, pigs produce a wide range of complex vocalizations that can indicate various conditions, from normal behavior to stress or illness, complicating the interpretation of these sounds [35,42,59]. Accurately distinguishing between these vocalizations requires sophisticated algorithms and significant computational power [25,26].
Additionally, environmental factors such as barn acoustics, temperature, and humidity can affect sound quality and detection accuracy. Moreover, the installation and maintenance of high-quality sound detection systems can be costly and may require technical expertise, which may not be readily available in all farming contexts [30,39,89]. Moreover, the lack of standardized protocols for sound data collection and analysis hinders the comparison and integration of data across different systems and farms [43,46,62]. There are also concerns about data privacy and security, as the continuous monitoring of sound data could potentially expose sensitive information about farm operations [8,9].
Despite these challenges, the future of sound-based technologies in pig farming looks promising. Advances in AI and machine learning are expected to significantly improve the accuracy and efficiency of sound analysis, enabling more precise monitoring of pig health and behavior. Moreover, integrating these systems with other precision farming technologies, such as IoT devices and real-time data analytics platforms, could provide comprehensive solutions for enhanced farm management [13,17,19]. Furthermore, ongoing research and development are likely to produce more robust and cost-effective sound detection systems, promoting their uptake by a wider range of farmers [29,31]. We also forecast that efforts to standardize sound data collection protocols and enhance data privacy and security will improve data sharing and integration across systems and farms, boosting farmer confidence [63].
As technology advances, sound-based precision tools are set to become essential components of smart farming, driving more efficient, sustainable, and humane pig farming practices. Their current limitations in sensor capacity and efficiency motivate ongoing research and resource optimization efforts to overcome the challenge of achieving high performance at a low cost, which is vital to fulfilling these tools’ potential in the agricultural sector.
CONCLUSION
Sound-based precision technologies have significantly advanced pig farming by enhancing management practices and productivity. The integration of sophisticated sensor technologies and advanced data analytics has revolutionized monitoring capabilities, allowing for detailed observations of pig behavior and health and environmental conditions. Recent improvements, including the use of machine learning techniques such as neural networks and deep learning models, have enabled more accurate classification and interpretation of pig vocalizations, promoting more efficient decision-making and proactive management. These technologies also offer valuable solutions to pressing challenges in the industry, such as achieving environmental sustainability and resource management. Acoustic sensors help optimize resource use and reduce energy consumption by continuously monitoring environmental parameters such as ventilation systems and feed distribution, and they facilitate the early detection of stressors such as heat or poor air quality, which enables timely interventions that mitigate negative impacts on pig health and farm performance. However, several challenges remain, including the need for standardized data collection protocols, improved interoperability between different sensor systems, and cost-effective implementation strategies. Addressing these challenges through ongoing research and development will be crucial for maximizing the benefits of acoustic-based precision technologies. While we have made remarkable strides with their development, further refinement and innovation are necessary to overcome the existing limitations. As these technologies continue to evolve, they hold the potential to significantly enhance management practices, improve animal welfare, and contribute to a more efficient and sustainable future in pig farming.